Copyright & Artificial Intelligence Listening Session - Visual Arts
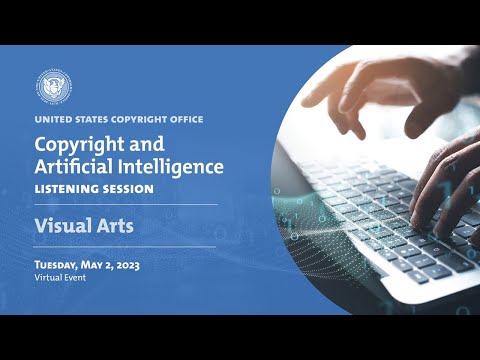
>> Mark Gray: Hello everyone. Thank you very much for joining us today. We are going to start our session now. Welcome to the United States Copyright Office's Listening Session on Artificial Intelligence and the Visual Arts.
Today, we are going to be discuss a variety of issues in the visual arts space. My name is Mark Gray. First off, I am an assistant general counsel here in the Office of General Counsel. Before we start our first panel, I would like to introduce Maria Strong for opening remarks. Maria is an associate registrar of copyrights as well as the director of policy and international affairs here at the U.S. Copyright Office. Maria.
>> Maria Strong: Thank Mark, and welcome everybody to the Copyright Office's Public Listening Session on Artificial Intelligence and Visual Arts. On copyright law, works of visual arts are broadly defined as pictorial, graphic, and sculptural works. Some examples include two-dimensional and three-dimensional works of fine, graphic, and applied art, photographs, prints and art reproductions, maps, globes, charts, diagrams, models, and technical drawings, including architectural plans. Because the visual arts include a wide variety of works, today we will ask broad questions to facilitate discussion across each participant's area of expertise. It's likely that almost everyone on this webinar has seen various images that deep learning text-to-image models can produce based on text prompts.
We've heard concern from artists and photographers about what the training and deployment of these models might mean for their livelihoods and their industries, both in terms of the input of their own images into these models as well as the excitement and concern related to the outputs. And the purpose of our session today is to discuss these issues. We want to hear how the public is thinking about policy issues raised by these technologies.
To begin to address the copyrightability and registration issues raised by works generated using AI tools, the office recently issued new registrated guidance in mid-March. That guidance makes clear that applicants have a duty to disclose the inclusion of AI-generated content and works submitted for registration. It outlines how to do so, how to update pending applications, and how to correct the public record on copyright claims that have already been registered without the required disclosure. There was a lot of interest in today's event. Unfortunately, we were not able to accommodate all requests to speak, but this is not the last chance to share your views on AI with the copyright office. As we've said before, and we'll say again, there are two more listening sessions happening later this month.
And down the road, we will be requesting written input through a public notice of inquiry. Please visit our website, copyright.gov/AI for more information and resources on our AI initiative.
Finally, we thank our panelists in advance for contributing to today's discussion and conversation. This is a complex topic and a deep personal one for all our panelists whether they are users or developers of AI technology, artists whose works help train that technology, or creators contemplating how AI will affect their careers. We are all looking forward to a thoughtful and respectful dialogue.
Let me turn the mic back to Mark Gray to outline the various logistics for today's session. Thank you. >> Mark Gray: Thank you very much, Maria.
So as a quick reminder before we get into specifics, today's listening session is the second of series of listening sessions that we are doing here at the copyright office going through the end of May. Each of our sessions is going to look at different topics, different types of works, and as a result is going to have different panelists and may even use different formats. So after today, we have two more sessions scheduled.
There is a session on May 17th, Wednesday, which will be focusing on audiovisual works, which would include movies and video games, and our final session will be on May 31st, which will focus on musical works and assigned recordings. The purpose behind these listening sessions is to inform the office's overall AI initiative. So some of the questions our panelists raise may be ones that we seek to explore further in written comments later this year. So please keep in mind that while there are a handful of my colleagues here from the copyright office on today on video, the rest of the office is in the audience and is listening, and all of this is going to help inform of our work. The schedule for today, the session format is going to be two panels of different sets of speakers followed by a third segment where a set of additional speakers will get the chance to share brief remarks. We are making a video recording of this, both of this session as well as the other three.
We are trying to get those online within three weeks of each session taking place, so please keep your eyes peeled for that if you have any friends or colleagues who don't have the opportunity to watch the session today. Before we get started, a few Zoom housekeeping notes. If you are a panelist who is not speaking at the current session, please keep your camera and microphone off and on mute, and then likewise, if you are a panelist, please keep your camera on and be ready to go off of mute when you're speaking. We will be recording this session today.
As I mentioned, the recording will try to go up in about three weeks from today, and we have enabled Zoom's transcription functionality for those of you who are interested in following along with captions. The way we're going to do the first panel is we're going to start with a brief introduction and short statement by each of the panel participants, if they so desire. We'd like you to try to keep those to two minutes. We're going to be keeping an eye -- the moderators may need to cut you off if it goes a little long just so we can keep everything on schedule. After those introductions and brief remarks, we're going to do a moderated listening session.
The panelists have received a set of broad questions in advance. Those are meant to prompt and guide discussion, but panelists and participants are welcome to share any other relevant perspectives or experiences they think are important for the copyright office to hear. If you are a panelist, please try to use Zoom's raised hand functionality, and we will try to call on you in the order that you raise your hands just to keep the conversation organized.
Please keep in mind this is a listening session and not a debate, so there will be other opportunities in the future for people to engage more directly with convening views, but the purpose today is really to help the office air out a variety of ideas and issues and perspectives for us to guide our own thinking. As a final note, I see we have some questions in the Q and A from the audience. Unfortunately, this is a listening session for the participants. We are unable to accommodate audience questions.
So, thank you so much for your interest. Please keep your eyes out on our website for future public participation and comment opportunities, but we cannot take any comments today, unfortunately, from you. With that, I'm going to hand it over to our moderators for the first session, Emily Lanza and Nick Bartelt.
Emily is a counsel in our Office of Policy and International Affairs, and Nick is an attorney advisor in the Office of the General Counsel. Emily, the mic is yours. >> Emily Lanza: Thank you, Mark, and welcome everyone. We will begin with introductions in the order as stated on the agenda. So first up, Scott with Adobe, would you like to go ahead? >> J. Scott Evans: Sure. Thank you for having me today.
My name is J. Scott Evans, and I'm senior director and associate general counsel at Adobe. For over four decades, Adobe's mission has been to empower our creative community with the tools that they need to express their imagination and earn their livelihoods in areas like photography, art, music, filmmaking, and design. AI and generative AI, specifically, have profound impact in these areas. So we really wanted to make sure that we, as we harness the power of this new technology, we're doing so in a way that empowers creators. Last month, Adobe launched its generative AI technology, Adobe Firefly.
Firefly's initial text-to-image model was designed to be commercially safe. That is, it was trained on images licensed from Adobe Stock, openly licensed content, and content in the public domain. We want our tools to be good for enterprises and the creative community. When it comes to copyright, we know that the issue of training is one where the creative community has concerns. For this reason, through a technology Adobe developed called Content Credentials, we're enabling artists to attach a do not train tag that will travel with their content wherever it goes. With industry adoption, it is our hope that this tag would prevent the training on content that has the do not train tag.
We're working with generative AI technology companies to respect these tags. From an output standpoint, for much of our professional creative community, generative AI serves as the front door to the creative process. They're changing the image.
They're adding colors, editing, adding elements. They're adding their own human expression to the work. So we need a way, a transparent way to track this expression. Here, content credentials can function much like an ingredients label. They'll show you where the image came from and what edits have been made to it. So for generative AI, it gives the creator a way to show that they started with a AI-generated image but most importantly to demonstrate the human creativity they brought to the work.
Finally, content credentials will bring a level of transparency that is much needed with the age of generative technology. Adobe is automatically attaching a content credential to images created Firefly to indicate the image was generated by AI. We're working to drive transparency standards so that together we can deploy this technology responsibly and in a way that respects creators and our communities at large. I thank for having me today, and I look forward to engaging further on these issues. >> Emily Lanza: Thank you, Scott.
Next up is Ben with Stability AI. >> Ben Brooks: Thank you to the copyright office for hosting us here today. I lead public policy for Stability AI, a leading developer of open source AI models designed to unlock humanity's potential. These include, as many of you know, the latest versions of stable diffusion, which is a model that takes a text prompt from users and translates that prompt into a new image. Users can interact with these models either through a hosted service, like an app or an API, or they can freely use, integrate, and adapt the open source code subject to our ethical use license. Stability has also launched a number of other image models as well as a suite of language models.
Stable diffusion is a type of latent diffusion model. So these models use content to learn the relationship between words and visual features, not unlike a student at a public gallery. Based on this acquired understanding and with creative direction from the user, these models can help to generate new works. In this way, AI should be understood as a tool to help artists express themselves. It's not a substitute for the artist. Instead, AI can help to simplify the creative process.
It can help existing creators boost their productivity as part of a wider workflow, and it can also help to lower barriers to entry for people who simply don't have the resources or training to realize their creative potential today, including those with life-altering injuries or disabilities. As with other assistive technologies, from paintbrushes to cameras to editing software, the user ultimately determines the content and use of any generated images. I do want to acknowledge today the depth of feeling on these issues among creators and developers. AI is changing rapidly, and we understand that it can feel highly disruptive.
We welcome a dialogue with all members of the creative community about the fair deployment of these technologies, and through this session today, I can share some details about how we're working towards that goal in practice, whether that be through new training techniques, authenticity standards, and best practices for things like opt outs. So thank you very much. >> Emily Lanza: Thank you, Ben. Next up is Alicia. >> Alicia Calzada: Hi.
I'm Alicia Calzada. I'm the deputy general counsel for the National Press Photographers Association. First, I really appreciate the invitation for NPPA to be a part of this event. This is very important to us and our members. Before I was an attorney, I was a photo journalist for 20 years, and through NPPA, we serve -- we are the nation's premier organization for visual journalists. We serve still photographers and videographers, and frankly most of our members do both.
Some of the things we do include working to support the First Amendment rights of visual journalists. We also advocate for their copyrights and for greater copyright protection and for a strong copyright system. We also have a code of ethics that is the industry standard among visual journalists, and that is, of course, a very important piece of what I hope we'll get into today. NPPA has a few concerns related to AI. The first, of course, is copyright protection for photographers against unauthorized use of their images and unauthorized copying. So we do support legislation that accomplishes that.
For us, it's not just about money. As I mentioned, we care about ethics, and for visual journalists, their representation is one of their most valuable assets. And so the right to control the use of their image and protect against misuse is very important. When their photos are used in an unethical manner, it impacts them. It impacts the entire industry, frankly. And we also think that news consumers have a right to know the source and the authenticity of the contents that they're consuming, the news that they're reading and watching.
Finally, a concern that we are monitoring is that journalists, like many photographers do use technology in some ways that are, in fact, quite ethical, and so we're watching what the copyright office is doing as they frame the question of what is copyrightable. We understand that something entirely AI created might not be copyrightable, but we want to make sure that in making policy we don't risk the copyrightability of photographs that for generations frankly have used special timers and triggers, such as the kind of things a sports photographer or a nature photographer might use. So those are some of things that are sort of on our radar related to AI, and we're definitely looking forward to this session to continue conversation on these issues.
>> Emily Lanza: Thank you, Alicia. Next up is Sarah. >> Sarah Conley Odenkirk: Hi. Thank you very much for including me in today's conversation. My name is Sarah Conley Odenkirk, and I'm a partner with Cowan, DeBaets, Abrahams, and Sheppard.
I cohead the Los Angeles office and also the art law practice group. My deep involvement in the implications of emerging technology in vessel arts goes back almost 30 years with my dedication to representing artists and also working to establish public policy around visual art in public spaces. The combination of these elements in my practice has positioned me well to do a lot of advising around the impact and implications of blockchain technique and now AI both from the standpoint of the impact on creators as well as on public policy. It's crucial to maintain the focus on the impact the technologies have on artist and artist abilities to continue to create and innovate. This becomes complex when we cannot easily determine when, where, and how potential copies and other copyright infringements may be occurring.
In order to explore possible futures, we need to start by breaking down the processes used in AI into their component parts as the analysis will likely suggest different solutions at different points. Figuring out fair, enforceable, an economically sound solutions to questions raised at the point of training AIs will differ from determining how to treat the output artists coax from these platforms. We also must distinguish the generic, generative process employed by commercial AI platforms from the more bespoke process of generative art as a medium employed by artists. So I urge the copyright office to consider the impacts on artists in light of the new structures that are made possible with these technologies and to adhere or even strengthen principles underpinning the copyright law that support balancing the interests of artists' innovation and creativity with the market forces. It may be time to consider more than just guidance, more than just analyzing what is considering copying or protectable.
I would love to see the copyright office take the lead in championing technical solutions that meaningfully address the way content is scraped, sourced, and used and explore realistic ways to track IP rights and compensate creators. >> Emily Lanza: Thank you, Sarah. Next up is Carla.
>> Karla Ortiz: Back in April of last year, I saw a website called Weird and Wonderful AI Art. It had the names of many of my peers alongside work that looked like theirs but wasn't. I thought, this is a new experiment. Well, I asked my peers whose names I saw on that website, and no one knew what this was, and no one had been asked to be a part of it. So we tried to reach out to the folks who were running the website, folks who were also selling merchandise that looked like the studies they were doing.
We asked them to please take down the work of the artists who didn't want to be there. Instead, we got ghosted. I thought this was small enough to ignore, but little did I know this would be my first encounter with generative AI.
Fast forward to September-ish, and the larger generative AI models like Midjourney and Stable Diffusion are now mainstream. So I research again, and I am horrified by what I found. Almost the entirety of my work and the work of almost every artist I knew was scraped and utilized to train these for-profit models. I was mortified that this was done so without anyone's consent, credit, or compensation, that once AI models are trained on our work, our work could not be forgotten, and that generative AI companies were even encouraging users to use our names to generate imagery that can look like our work. For example, Polish artist, Greg Rutkowski, who in December between Midjourney, Stability AI, and the very problematic Unstable Diffusion, Greg's name had been used a prompt for image generation about 400,000 times. If there is one thing I want everyone to remember is that this high technology is entirely fueled by the ill-gotten data it is trained upon.
It is unlike any tool that has come before, as it is an innovation that uniquely consumes and exploits the innovation of others. No other artistic tool is like this, and I know. I've used most of them. In my opinion, to reward tech that relies on the proceeds of theft by granting it copyright would just add insult to injury.
Oh, also, my name Karla Ortiz. I am an award-winning artists who works in film, game, TV, galleries, you name it. I worked on Magic The Gathering, Guardians of the Galaxy Volume 3, Loki, and most notably known for my design of Dr. Strange for the film adaptation. I am also a plaintiff in one of the first class-action lawsuits against generative AI companies, specifically Midjourney, Deviant Art, and yes, Stability AI. Hi.
>> Emily Lanza: Thank you, Karla. Next up is Curt. >> Curt Levey: Hi there.
I'm Curt Levey, president of the Committee for Justice. We're a nonprofit that focuses on a variety of legal and policy issues including intellectual property, AI, tech policy. There certainly are a number of very interesting questions about AI and copyright. I'd like to focus on one of them, which is the intersection of AI and copyright infringement, which some of the other panelists have already alluded to.
That issue is at the forefront given recent high-profile lawsuits claiming that generative AI such as DALL-E 2 or Stable Diffusion are infringing by training their AI models on a set of copyrighted images, such as those owned by Getty images, one of the plaintiffs in these suits. And I most admit there's some tension in what I think about the issue at the heart of these lawsuits. I and the Committee for Justice favor strong protection for creators, because that's the best way to encourage creativity and innovation, but at the same time, I was an AI scientist long ago in the 1990s before I was an attorney, and I have a lot of experience in how AI, that is, the neural networks at the heart of AI, learn from very large numbers of examples, and at a deep level it's analogous to how human creators learn from a lifetime of examples.
And we don't call that infringement when a human does it, so it's hard for me to conclude that it's infringement when done by AI. Now some might say, why should we analogize to humans? And I would say, for one, we should be intellectually consistent about how we analyze copyright, and number two, I think it's better to borrow from precedents we know that assumed human authorship than to invent the wheel over again for AI. And look, neither human nor machine learning depends on retaining specific examples that they learn from. So the lawsuits that I'm alluding to argue that infringement springs from temporary copies made during learning. And I think my number one takeaway would be like it or not, a distinction between man and machine based on temporary storage will ultimately fail, maybe not now but in the near future. Not only are the relatively weak legal arguments in terms of temporary copies the precedent on that, more importantly, temporary storage of training examples is the easiest way to train an AI model, but it's not fundamentally required.
And it's not fundamentally different from what humans do. And I'll get into that more later if time permits. But I think the good news is that the protection for creators of the works that are used as training examples can and will come from elsewhere.
Where the generated output is too similar -- >> Emily Lanza: Thank you, Curt. I'm going to have to -- sorry, I'm going to have to cut you off there. >> Curt Levey: Okay.
>> Emily Lanza: But -- >> Curt Levey: Sure. >> Emily Lanza: -- we'll have time for and during the questions to continue. Rebecca, would you like to go ahead, please? >> Rebecca Blake: Yes. I'm happy to go ahead, and I'm apologizing in advance for the construction that has just started up outside my window. My name is Rebecca Blake.
I'm the advocacy liaison for Graphic Artists Guild. The Graphic Artists Guild is a trade association representing the interests of visual artists other than photographers, illustrators, designers of all types, production artists, cartooning and comic book artists, animators, and others. Our mission is to protect the economic interests of our members, and in that vein, we've long advocated for greater copyright protections for individual artists, fair labor, and trade practices, and policy which supports small creative businesses.
We welcome this opportunity to weigh in on AI generative technologies. Our members include artists who have embraced generative AI in the creation of their own original works and artist who for various reasons have not adopted the use of generative AI or in fact see it as a threat to their livelihoods. While we support the ethical, legally compliant development of AI as a tool for visual artists, we have serious concerns about the copyright and ethical questions raised by AI generative technologies. These include the inclusion of copyrighted material in the training datasets without permission or notification, which we see as a copyright infringement not excused by fair use, protections for artists' works as inputs into AI generative platforms, the unfair competition in the marketplace resulting from the massive generation of images, which may ape existing artists' styles or replicate artists' works, confusion with the registration of works containing AI generated material, and existing barriers to the affordable registration of works created by visual artists other than photographers. And I hope we can go more into this in the subsequent questions.
>> Emily Lanza: Thank you, Rebecca, and last but not least, James. Would you like to conclude the introductions, please? >> James Gatto: Yes, thank you. Hi. My name is James Gatto. I'm honored to have the opportunity to share some views here today on the important copyright issues with AI. I'm a partner in the DC office of Sheppard Mullin where I lead our AI practice. I've been an IP attorney for 35 years.
I'm also a member of ABA IP section, AI machine learning task force, but the views expressed today are solely my own. I've been doing work with AI for about two decades, but like others, I'm seeing a significant increase in that work due to the meteoric rise of generative AI. Clients have a lot of questions. I applaud the copyright office's initiative to issue preliminary guidance on the examination of applications involved AI-generated content.
I know there's great debate in the community on these guidelines, on authorship issues with AI, the level of human involvement needed, and issues with joint authorship. I hope these listening sessions will result in the copyright office keeping an open mind on whether to tweak their guidance and provide further clarity on some of the procedural aspects of the guidance. Some of the issues for which clarity would be helpful are the following. When does the level of detailed input or prompt by a human provide sufficient basis for the output to be deemed original intellectual conception to the author and therefore protectable? What is the relevance of predictability in the authorship analysis? This concept was part of the basis for the Kashtanova decision, but does not appear in the guidance. What level of detail is needed to comply with the duty of disclosure regarding use of AI? What is the copyrightability of a work where a human uses AI generated content as inspiration art but does not copy it? And what are the criteria for determining if AI generated content is more than de minimis such that it should be explicitly excluded from the application? AI is a powerful tool, and to promote the constitutional mandate, the copyright office should develop policy that promotes rather than deters its use.
As a result of the guidance in the Kashtanova decision, at least many companies that rely on copyright protection for their content, including game [inaudible] companies, artists, and many others are concerned about using generative AI and in some cases restrict or limit employees' use of it. That's not consistent with the goal of promoting the use of technology. So we hope through these sessions we get to a happy medium where artists' rights can be respected and tools can be used to facilitate the creation of their expressive works. >> Emily Lanza: Great. Thank you, James, and thank you, all, for those introductions and welcome again.
So to begin our discussion, I'll start off with a question. How is the training of artificial intelligence models affecting your field or industry? What should the copyright office know about the technology's use of training materials when considering the copyright issues related to training? And also, please be specific in your answers in terms of kind of when part of the visual arts ecosystem you're talking about. So, great, I already see hands. So Karla, you're the first on my screen.
Can you please go ahead. >> Karla Ortiz: Yeah, absolutely. So our -- so basically, the training of artificial intelligence is already affecting my part field of entertainment, specifically concept art as illustrators, anything that requires a painter. We're already seeing the affects of these tools, you know, in our industries. Something to consider is the training of these tools is very important.
When considering these tools, you can't just focus on the output. You have to see the entire process as a whole. And as a whole, these tools, you know, particularly some of the tools around here, like Stability AI, specifically under the pretext of research gathered 5.8 billion text and image data from across the internet to train various AI ML models for commercial purposes.
Again, it was trained upon for research and then switched immediately to for commercial purposes. Technologists like Andy Baio calls this, you know, loophole data laundering. But another thing that's important to note is that this was done so without consent, credit, or compensation. The work of myself and almost all of my peers are in those datasets. Again, and also our names are, you know, encouraged to be utilized as prompts so that users can get something that mimics or feels similar to our work. I personally am of the belief that the work generated by these models is impressive only because it is based upon the works of artists.
And again, this is done so without consent. And we're not even talking about all of the issues when it comes to propaganda, identity theft, and so on. One of the things that I will say as well that the copyright should consider, and I won't take much longer of anybody's time, so people can have their say. As one of the few artists in this panel, you know, there's various others as well, but I'm a teacher, and I can tell you that anthropomorphizing these tools to equate it as human-like is a fool's errand.
I've spoken to countless machine learning experts, such as Dr. Tim McGebru [phonetic], such as Professor Ben Chow [phonetic], and they all agree that is not what's happening. This is a machine.
This is mathematic algorithm. You cannot equate it to a human. And to further add and to give the perspective of an artist, and artist doesn't look at a bunch of like 100,000 images and is able to generate like hundreds of images within seconds. An artist cannot do that. Yes, I am -- I have my influences, but it's not the only thing that goes into my work.
My life, my experiences, my perspective, my technique, all of that goes into to work. Furthermore, something that I feel like a lot of people miss in these discussions is technical artistry, and one of the hardest things you can do ever in the arts is to be able to successfully mimic another artist's style or another person's work. It's the hardest thing. I consider myself masterful.
I can't even do it. In fact, it's so rare that they even have documentaries on Netflix showcasing the few artists that can successfully mimic let's say a Leonardo da Vinci. And depending on what that artist does with that successful mimicry, if they sell it or if they do anything commercial with it, you know, that could be potentially be called forgery. So I don't know why -- >> Emily Lanza: Thanks Karla.
Sorry -- >> Karla Ortiz: Oh, yeah, no, no, no. >> Emily Lanza: Sorry to -- >> Karla Ortiz: It's totally cool. No, no, no. >> Emily Lanza: Yeah, we just have a whole [inaudible]. >> Karla Ortiz: No, no, no. Totally great.
Just wanted to drop that in there. Bye. >> Emily Lanza: All right. Thank you, Karla. James, you're next on my screen. Please go ahead.
>> James Gatto: Great. Thank you. I'll try to be brief. So I mean obviously one of the core issues with training AI model on copyright protected content is whether it's infringement and/or if fair use applies. And largely, that's going to be a fact-specific question depending on the details.
I think that to the extent there, you know, are any policy considerations or guidance the copyright office, you know, can provide in that, that might be helpful, but there is a pretty significant existing body of law on that, kind of the broad legal test. I think some of the areas that should be considered consistent with what Scott said from Adobe, there's a lot of tools out there that can be used that help mitigate the problem, and whether those tools should be mandated or, you know, some other role the copyright office can play with respect to them would be helpful should AI tool providers be required to be more transparent on the content they used to train their models. I think that's an important is should there be greater use of tools that prevent AI from using copyrighted works to train AI, similar to how [inaudible] techs work to prevent search engines from indexing certain web content.
The technology is there, and some of the concerns can be abated if these tools become mandated or just widely used. And the last point I'll make is maybe not directly relevant to visual arts but just -- you know, there's other content that using it is not a problem because it's licensed. So whether it's open source software that's being used to train AI code generators or images that are under a permissive license like Creative Commons, as long as there's not prohibition on commercial use, the use of it may be permissible, but the question is then are there license compliance obligations that need to be met and, you know, whether and how those should be dealt with in these contexts. Those are just a few of the issues I think would be helpful to consider. >> Emily Lanza: Thanks James.
Alicia, you're next on my screen. Please go ahead. >> Alicia Calzada: That's a really interesting point about things like Creative Commons that actually do have conditions to, you know, what seems like on the surface an unlimited license, but actually there are things you have to do in order to earn that license. Back to the question about how it affects our industry. The primary concern, as I mentioned earlier, in our industry, really is an ethical one.
And journalists rely on copyright as a means of controlling how their work is used. And it's one thing to say, isn't it neat what this computer can do while you're, you know, just goofing off with friends or doing research or that kind of thing, but, you know, when these works start being used to create deep face or images that are used to promote civil unrest, there are a lot of ways that news images can be abused through this kind of a process in very, very negative ways. And telehealth journalism industry really is concerned about where that's going to go and how it impacts the industry as a whole. You know, we already have editors who have, for decades, you know, paid very close attention to work that they don't, you know, to work that comes in to ensure the quality of the sourcing and that kind of thing, but on some level there's things out there in the world that we worry about people seeing and thinking is journalism when it really isn't.
>> Emily Lanza: Thanks Alicia. Next, Curt, you're next on my screen. Please go ahead. >> Curt Levey: Sure. Let me -- let me first briefly finish what I was saying about the good news for protection for creators despite the fact that I do think it's getting harder and harder to distinguish between what humans do and machine do.
But regardless of how they're trained, where the generated output is similar to one of the examples in the training data or really any preexisting work, it's a derivative work or an outright copy. And the licensing requirements for derivative works need to be as strictly enforced as for non-AI works. And then, second, and some of the others have alluded to this, since the source of the training data is typically unlicensed data, I should say publicly available data, or webscraping, we need strict enforcement of the website or database terms of service, and Mr. Evans mentioned do not train tag.
That's a good example. I also, when you said what should the copyright office be aware of, I wanted to say a little bit more about temporary storage and why that's not fundamentally required. Generative AI learns from a very large number of examples, and so does a human artist or author. The artist or author is not born with that ability. He or she learns from countless examples of art, photography, music, written works, etc. And, you know, more and more the human views those examples on a website.
The human may purposely make copies of the examples he views, and even if he doesn't purposely do it, his computer makes a temporary copy as he views the image, reads the written work, etc. Yet, we all dismiss that copying as fair use, even if, you know, if we even acknowledge it at all. So what AI training does is not very different.
For convenience sake, the examples are put in a database which learning algorithm cycles through, and that is temporary copying, but humans, like I said, often copy for convenience sake as well. And once the AI cycles through the examples in training, the examples can be thrown away. The trained model consisting of millions or billions of weights analogous to the synaptic connections in the human brain retains no copies of the training examples. Human memory, on the other hand, does remember at least some specific examples. So in some sense, there's less of an infringement danger with AI than humans.
But to be fair, neither humans nor AI depend on retaining the specific examples they learn. So again, the problem with relying on the temporary copy argument is that it's not really necessary. You could train the AI model by having it scroll through the very same images or written works that the human learned from. In fact, the AI model could learn from, you know, data being relayed by a mobile robot that, you know, visits art galleries throughout the nation. Someday, you know, that may be how it's done. Think Google maps.
Either way, my point is that hanging one's hat on temporary copying is skating on very thin ice. >> Emily Lanza: Thank you, Curt. Next up is Rebecca. Please go ahead. >> Rebecca Blake: Yeah.
Gosh, there's just so much to unpack from that previous answer. Very quickly -- >> Emily Lanza: Rebecca, you muted yourself. Can you unmute? >> Rebecca Blake: I'm so sorry. >> Emily Lanza: That's all right. >> Rebecca Blake: Very quickly, some of our members completed eschew using AI image generators. They're concerned about the ethical concerns with the way that image datasets were built.
They're worried about copyrightability, and they're worried about exposing their clients to infringement. Other members of the Graphic Artists Guild in fact use AI image generators. For the most part, we're hearing that they use it for ideation but not for the creation of completed works, or they use it to generate elements of a much larger work, for example, to create background graphics. We do have one member who in fact is very -- has a career in AI generative -- for an AI image generator, part of that new generation that has achieved a career. However, we've been trying to gauge job loss, job creation, job loss, and we're in very, very, very early days to be able to do that.
It's something we need to start tracking now that these generators have been out almost a year. However, we do hear a lot of anecdotal evidence of job loss. It's in particular sectors. That is hampered by the fact that many of the artists working in these areas in fact signed NDAs or are reluctant to go on the record discussing projects that they've lost because they're afraid of retaliation. They work in a very small industry. Of our members who do use generative AI, one member stated that he was able to take on larger projects with a smaller workforce, so that does indicate that generative AI permits a streamlining and less hiring of artists.
And another member stated that because she used AI generative technology, she was able to cease contracting to a certain number of designers. So, again, that indicates a benefit to one member but at the loss of another. So that's speaking to the job market. But I wanted to address two other things. So, first of all, was this idea, this equivalency of the way machine learning works to the way human learning works. This is a false equivalency for a very, very major reason.
When a human learns to draw, they will ape. They will copy the styles or the existing works as illustrators they admire. This is very common in the learning process. But there are ethical considerations, copyright considerations, and best professional practices that professional illustrators follow that take them away from the wholesale copying of either a style or in fact of an image itself. This does not occur with machine learning. The machine is not driven by a creative process, a desire to develop ones own style, ones own mark, ones own creativity.
It simply reiterates a style that it has been learned on. So there is no equivalency in the output. The second thing I just wanted to touch on very briefly was this idea that there can be times or codes or metadata, which is embedded in images, which in fact permits one to track whether or not an image can be used for inclusion in the dataset, whether it can be adjusted into a platform, etc. There's a huge issue with that, which is that section 1202 of the Copyright Act permits the removal of copyright management information including metadata if that removal is done knowingly or with reasonable grounds to know it will induce, etc., etc., infringement. We believe that section of the act needs to be modified so the removal of CMI, including metadata, without permission of the copyright holder is prohibited, regardless of whether or not it's done knowingly to permit infringement.
We've seen metadata and CMI as key to being able to protect artists' works in an AI environment, but that failure in section 1202 needs to be addressed. >> Emily Lanza: Thanks Rebecca. And next up is J. Scott.
Would you like to go ahead? >> J. Scott Evans: Sure. You know, at Adobe, we believe that if AI is done right, if this is done right, it benefits both creators and consumers of content because it does nothing but amplify human creativity and intelligence. It doesn't replace it. And so, what we see as a major issue here is that creators have -- now have unlimited resources to attribute the work, especially when generative AI comes into play. One of the important things that we need to do as a collaboration with artists and technology is to put creators at the forefront of this technology. Creators want control over their work is used in generative AI training, and we need to give them the tools in order to make those decisions.
We know many creators that are very excited about this technology and want their creativity to be used in training these models. They are very excited about them. But we do understand that there is a segment of the community that is not excited and wants the ability to prevent the use of their art in training, and they should have an ability to do so.
And that's the reason Adobe has developed the content credentials. We work very hard with setting up an open source industry standard with the content, the coalition of content and providence and authenticity, the C2PA. It's an open standard that platforms and hardware manufactures can put into their products that will allow you to put these content credentials that will surface them to users and developers of AI technology so that those cues can be followed. And that's something that we thing that's very important.
We also think there may be technology where artists could harness this technology by training models based solely on their own style or brand and then commercializing that and having that technology and understanding that there are different ways that this technology can be used is very important. One of the ways the copyright office, I think, can help in this is to encourage industry to adopt these open standards that will give artists the ability and tools in order to identify whether they want to participate or don't want to participate and encouraging that kind of proactivity among the companies that are developing this technology, to give artists a tool to control their creative works. >> Emily Lanza: Thank you.
And Sarah, please go ahead. >> Sarah Conley Odenkirk: Thank you. I think I'm going to be reiterating a number of things that have already been said, but first I'd like to say that, you know, I think that there's a lot of reason to be concerned about AI in general. There are big issues, bit global, ethical issues that definitely need to be addressed. Unfortunately, I think we do need to somewhat separate those questions from these questions that we're talking about with regard to copyright issues in order to parse through things, otherwise we're going to very quickly get side tracked with, you know, scary, potential future possibilities, which I don't think we should ignore, but we need to separate that out of the copyright conversation for now.
There's clearly a lot of potential in addressing some of the training issues through metadata and through some of the tools that Mr. Evans was speaking about as well as some other tools that have been developed and people are looking to in order to protect the content. And I'd like to underscore what Rebecca said with regard to section 1202, and you know, really needing to be concerned about the way in which the metadata can be taken off of content and thereby allowing it to be misused and really keeping creators from being able to track that data.
So I think that the final point that I want to make is with regard to paying attention to the purpose of the use that the images are being scraped and collected for, and if what we're talking about is using those images for the purpose of creating a commercial venture, a commercial product that's to be used to earn money, that's a very different use than artists looking at images and using tools in order to generate art. And while they're obviously connected, I think we need to look at them very separately in terms of figuring out what policies and laws and approaches we can take to protect creators in the front end of that process. >> Emily Lanza: Thank you, Sarah. So before we move on to question two, I just want to make sure everyone had a chance to speak. Ben, would you like to add anything to question one before we move on? >> Ben Brooks: Yeah.
Thank you, Emily. I think just on this question of impact, I think these go to a broader set of issues around style and authenticity. I do have remarks on training specifically for later. But I want to reiterate what I said at the beginning, which is that we see AI as a tool to help artists express themselves, but it's not the substitute for the artist. That said, we obviously support efforts to improve creator control over their public content. And we're focusing those efforts in three areas in particular.
So one is around access to content. Today, already, datasets like LAION-5B respect protocols like robots.txt that indicate whether a website can sense to automated dot aggregation.
But we're also developing new ways to help creators qualify the use of that public content for AI training. So one of the things we've done is we've committed to honoring opt out requests from creators in the next wave of Stable Diffusion releases. And going forward, I think this was a point alluded to by J. Scott.
We're also exploring new standards for opt outs so that the opt out metadata will travel with the content wherever it goes, subjects them to the problems that have been flagged just a little while ago. The second area we're focusing on is authenticity of content. So we're working to implement content authenticity standards like C2PA with the content authenticity initiative so that users and platforms can better identify AI-assisted content.
By distinguishing AI-assisted content, these standards can help to ensure that users apply an appropriate standard of scrutiny in their interactions with that content. It can help to limit the spread of disinformation through social media platforms, and ultimately, it can also help to protect human artists from unfair mimicry or passing off. And the third and final point I just want to make is the work that we're doing to improve the quality of datasets. So, for example, by improving the diversity and reducing the duplication in training data, we can help to mitigate the risk of things like overfeeding, which is where the system erroneously overrepresents certain elements of a particular image from the dataset.
So, for example, if you've only ever seen sunsets, you might think that the sky is always orange. In addition, by improving diversity in our datasets, we can be more representative of diverse cultures, language, demographics, and values, all of which can help to mitigate the risk of bias in those outputs. So I think the final point on this question I'll add is, you know, we believe the community will continue to value human-generated content, right. We carry a complex digital camera in our pockets everywhere we go, yet we continue to value painting. Likewise, Photoshop didn't destroy photography.
We have machines that can run faster than athletes, but we continue to place a premium on sport. And the same will be true of visual arts in the post-AI creative economy, particularly when we have some of these content authenticity standards in place. >> Emily Lanza: Thank you, Ben. I'll turn it over to my co-moderator, Nick, for the next question. Thank you.
>> Nicholas Bartelt: Thanks Emily. And thanks everyone. I think the focus of the first question that Emily had asked was a little bit more on the input and the training.
So I think we'll shift the discussion a bit to ask what should the copyright office be aware of regarding how these AI systems and some of you have already touched on this, how these AI systems generate works of visual art, and then as sort of a sub question there, I'll ask, because I know we have limited time, is that, you know, are there any copyright considerations that vary based on the type of visual works that are at issue there. So I see James' hands first, and I know Karla, we had lost you a minute ago, but I see you're on there too. So we'll go through -- go ahead, James. >> James Gatto: Great. Yes. Obviously the operation on the AI tools vary, and each case is fact specific.
We recognize it's the challenge for the copyright office to give guidance for all scenarios, but there are a number of fact patterns that are common, and I think what will be helpful, one thing that will be helpful is kind of like the patent office did with patent eligibility guidelines, if the copyright office could provide examples of situations that use generative AI that they would deem to be copyright protectable, that would be helpful. The other thing is, just to take one use case, so I do a lot of work with NFTs as well, and there's a lot of concern around the use of generative AI for some of these NFT projects. If I create NFTs that represent images, and for example, I specify two images of dogs, each having a different collar that I design and different colors, and I use AI just to generate the permutations of those artistic elements that I created under my control saying produce those permutations, AI should just be deemed a tool, even though it's output from a generative AI. The question is, as you scale that up, and maybe some of the parameters are a little bit looser, where does the line get crossed between it being my creative expression and it being too much input from general AI? That's one practical use case that we've seen in a number of these NFT projects. So I think that there's many other -- I'll be brief so others can talk, but there's many other, I'd say, common uses cases that we're seeing, and I think that any input or guidance or examples that the copyright office could provide would be very helpful, to, you know, assist those in trying to figure out where the line is and recognizing that, you know, through our fact-specific differences.
Thank you. >> Nicholas Bartelt: Okay. Thanks James. Actually, because I know Karla had her hand up before we had switched the question, we'll go Karla and then Ben and then Curt. So go ahead, Karla.
>> Karla Ortiz: Wholeheartedly appreciated. So something that I think the copyright office should be aware of regarding how AI systems generate work of visual art. There's been some talk at the idea of like whether these models copy, remember, memorize, whatever the word, over fit, whatever the word really is, something I would like the office to know is that studies are being done concerning these issues. For example, there's research from the University of Maryland and the University of New York, they did a study that found diffusion models generated high-fidelity reproductions, which is basically plagiarism, at an estimated 1.88%, and it is estimated
by these researchers to be higher. [inaudible] numbers, but let's take a look at like Lensa AI, which uses Stable Diffusion, has about, you know, this was around December, has about 25 million downloads and gives the users about 50 trials each. At 1.88%, that's potentially 23,500,000 generated images that could be similar to training data, and we see this consistently with like, for example, Steven McCurry's famous Afghan Girl can be perfectly plagiarized by these tools, as it happened in Midjourney. Another thing that I'd like to, you know, folks to consider as well is like the music, you know, Stable Diffusion, no offense to them, but Stable Diffusion already has made the case for us. For example, I mean AI companies have already made the case for us, for example, Dance Diffusion was a music program developed by Harmoni, which has links to Stable Diffusion, and as they train their model, they stay clear from copyrighted data and only did public domain.
And one of the things that they quote on is because diffusion models are prone to memorization and overfitting, release a model trained on copyrighted data could potentially result in legal issues. Why was this done for the music industry but not the visual industries? And this also goes to the point to why opt out is inefficient regardless of what it should -- the stand should be opt in, because opt out places an undue burden on people who may not know the language, who may not be online, who may not even like know what's going on. Companies cannot just arbitrarily grab our copyrighted works, our data, and just say this is ours, and then later on we have to remove ourself, which is why opt out is important. The other thing that's really important, again, is transparency, and I know that Adobe, you know, is mentioning this, but for example, we need to really know what, for example, open license works mean. We really need to know and have an open dataset to see exactly what it means so that licensers who, you know, can actually like fulfill their licenses. >> Nicholas Bartelt: All right.
Thanks Karla. >> Karla Ortiz: Oh, I forget the guidance on you, but you guys go on ahead. If we have time later, let's do it. I'm sorry. >> Nicholas Bartelt: Okay. Yeah. I just want to make sure we get through everybody in our remaining 10 or so minutes.
>> Karla Ortiz: Yeah. [inaudible]. >> Nicholas Bartelt: So, I'll go back to you now, Ben. I think you mentioned what should the office be aware of how these AI systems generate work? >> Ben Brooks: Yeah, look, I think it's important that we properly characterize the training process, right. These models are not, as has sometimes been described, you know, a collage machine or a search index for images.
These models review pairs of text captions and images to learn the relationships, again, between words and visual features. Right. So that could be fur on a dog or ripples on water or moods like bleach or styles like cyberpunk. And with that acquired understanding and with creative direction from the users, those models can then help the user to generate new works.
So in this sense, training is, we believe, an acceptable and transformative use of that content. But there are some good instinctive examples as well. Stable Diffusion notoriously struggled to generate hands.
So it produced 2-finger hands or 12-finger hands because it doesn't know that a hand typically has five fingers, and it isn't searching a database of the many images with hands, right. Instead, it has learned that a hand is a kind of flesh-colored artifact typically accompanied by a number of sausage-like appendages. All right. And that all has real implications for how we should think about AI training in generation.
In other words, these models are using knowledge learned from reviewing those text-to-image pairs to help the user generate a new work. They're not using the original images themselves, and those images are nowhere in the AI model. >> Nicholas Bartelt: All right. Thanks Ben. And Curt, we'll go to you next, and just so everyone knows, we have about two minutes for the remaining five people with their hands up before this panel ends.
So if you have any concluding remarks, you know, kind of work them into whatever you have to say here. Thank you. >> Curt Levey: Well, let me answer the question, but also, in a sense, these are concluding remarks. A couple of the panelists feel strongly that machine learning is not like what humans do. So let me say more about why I believe it is very similar.
The human brain consists of neurons connected by synapses of various strengths. So when a human sees an example, those synaptic strengths are slightly modified. Modification takes place slowly, but you know, given a lot of examples, there's a lot of modification and learning. That is how we learn.
Neural networks consist of artific
2023-06-04 05:48