Data Harmonization of Large Digital Technology Datasets for Aging and Dementia Research
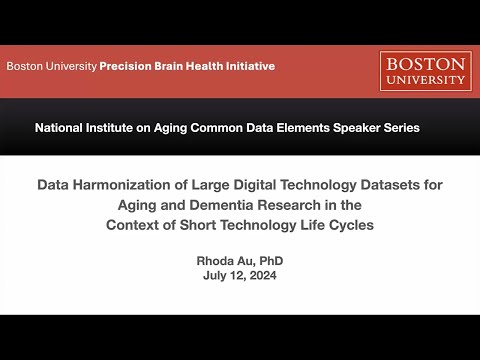
Mastan Narne: Okay. Good. To go. Karyn Onyeneho: Greetings everyone, and welcome to the National Institute on aging Karyn Onyeneho: common data elements. Webinar. Karyn Onyeneho: my name is Dr. Karen on Yanaho, and I'm the the Advisor for genomic data sharing with the National Institute on aging in the division of neuroscience.
Karyn Onyeneho: Before we get started today, I just wanted to go over a few housekeeping notes one of them being our our audience is going to be muted. However, we have in while that Karyn Onyeneho: with with the audience being muted. We have disabled the chat feature. However, the QA. Window is enabled, because we want to solicit your questions throughout Karyn Onyeneho: today's Q&A segment and the talk from our speaker. Karyn Onyeneho: I also wanted to share that today we have cart captioning which is being provided by Miss Adrina Atkins.
Karyn Onyeneho: And lastly, we have Mr. Narn Mr. Rowan, who's with us today to provide us with our it support needs. Karyn Onyeneho: And so I'll go ahead and get started. I am very, very pleased Karyn Onyeneho: to welcome you all to our 4th installment Karyn Onyeneho: of our monthly webinar as part of our common data Elements Speaker series that actually began back in April, and will go through December of this year. The Series features a dynamic lineup of speakers who will highlight best practices, standards and applications that optimize the usage of data resources as well as effective data management and enhanced data analyses. Karyn Onyeneho: Our Speaker series is designed to allow the Nia community to connect with Alzheimer's disease and Alzheimer's disease related dementia and aging research while facilitating discussions about resulting research findings Karyn Onyeneho: and other information as it relates common data elements better known as Cdes. Karyn Onyeneho: So this afternoon we are so delighted to have Dr. Rota O. As our featured speaker for today's webinar.
Karyn Onyeneho: which is titled Karyn Onyeneho: data harmonization of large digital technology data sets Karyn Onyeneho: for aging and dementia research Karyn Onyeneho: in the context of short technology life cycles. Karyn Onyeneho: Dr. O. Will discuss the linkage of Cdes with large digital technology data sets for aging and dementia research Karyn Onyeneho: with highlights about effective approaches towards applying Cdes for data harmonization. Karyn Onyeneho: But before we get started. I wanted to share that Dr. O. Is Professor of Anatomy and Neurobiology, Neurology, medicine, and Epidemiology at the Boston University, Bonian and abedition. If I hopefully said that right school of medicine and school of public health. Karyn Onyeneho: her research focuses on aging and dementia, as well as included as well as includes related cardiovascular risk factors, brain MRI measures and neuropathology to cognitive performance. Karyn Onyeneho: Doctor O also serves as one of the principal investigators of the Framingham Heart study brain aging program. And she's also the Director of Neuropsychology. Doctor O. Received her doctoral degree from the University of California in Psychology. With that, without further ado. Doctor OI will now turn over the presentation to you to get started for our lecture today.
Rhoda Au: Thank you. Thank you so much for having me. I I I'm I'm actually happy to be here. I'm honored to be here. I also wanna to make a caveat statement, which is that you know, I I'm a neuropsychologist. So I actually don't handle the data, but because I've been so involved, particularly with the Framingham Heart study. You know, I've had to worry about data harmonization. I've had to worry a lot about data sharing. And so that's what I'm gonna share with you today. But I do wanna Rhoda Au: mentioned that a lot of what I will share with you is actually the work of my colleagues. And but hopefully it will meet sort of the purposes of this. So just very quickly. These are my disclosures. Rhoda Au: and where I wanted to start, I'd like to always provide some context. So this is the scientific vision. Right? This is when we do research. What we're trying to do is figure out, how do we do it? In a way that includes everybody. We want to do representative research? That both in terms of represents the global population, all of us. Rhoda Au: But it also needs to include the voices of the scientists as well, and that equally needs to be represented globally. Rhoda Au: Now, the problem with how we do research is typically we have people come into a clinic, you know. Then we have. We give them, you know, all sorts of questionnaires. And we do also all these different procedures. And what's that? What that has led us to is we're doing really, really Rhoda Au: limited research when we think about this from a global perspective. So if we think about Alzheimer's disease, for instance, it's a global pandemic. But this is the reality of where it's actually happening.
Rhoda Au: So you know, as a result of this work, we actually have lots and lots of gaps in our research. So we have diversity graph gaps in terms of race ethnicity, income, geography. We also, we also think about dementia as a life course disease. And yet most of our data is in the, in the upper decades of life. We're actually missing a lot of the data earlier. And then the bigger problem is is that we run all these cohort studies. Rhoda Au: and whether they're 80 specific cohorts, or if you even think across all research cohorts, you know, I work with the Framingham Heart Study, which in its name did not start out as an ad cohort study, but has evolved into one. But if we think about all the cohort work that's happening all over. It's actually relative to the representation of the world population just to drop in the bucket. Rhoda Au: So what we've been doing is trying to think about, how do we actually get to a global solution where we really are maximally, including anybody anywhere. And what we've hit upon is what I have right here, which is really a minimal viable protocol. We can all collect clinical data, you know, to some degree we can collect blood. And we can collect digital
Rhoda Au: and we particularly can collect digital because of the penetration and digital in the space in the, in the research world or in the general world, and we'll get to that in a little bit. And then we can layer on to that additional technologies, for instance, or additional procedures that can happen when you're in a in a higher resource setting. So together, we can build sort of the pyramid of a comprehensive research data resource. Rhoda Au: So one of the things that we try to focus on on the digital front, on the technology front is really taking advantage of the smartphone Rhoda Au: because because it is the most penetrating technology in the world. So currently, there's about 6.7 billion users who own a smartphone. And that number is gonna continue to rise. So we're talking about almost worldwide penetration. And one thing that's important to work to understand about a smartphone is, it has embedded in it multiple sensors Rhoda Au: which you can think about as data collection tools.
Rhoda Au: Now, it turns out, Nia is already on board and trying to support sort of bringing digital tools into this space. So here are some of the large cohorts, you know, studies that are already bringing digital in a pretty large way into their world. And what I'm gonna do is oh, and sorry about that and and then on top of that, this is really sort of an idea of how much Rhoda Au: digital is really penetrating from all over. There are so many Internet connected devices. And so this is why there's an opportunity here. Opportunity here from a cohorts perspective. And again, thinking about, how are we gonna fill all those gaps that currently exist? This is the. This is the opportunity before us. Rhoda Au: So one of the things, though, that we want to think about, though, is the reality of the technology life cycle. It turns out that there are many different technologies which can get become obsolete in a very short period of time. So this really gives you sort of the typical idea of a life cycle and the and that the timeframe for any one technology is actually very
Rhoda Au: sure, because one of the things that happens as we're all pretty aware of is when you release any technology. Rhoda Au: there's constantly new versions. Right? There's new versions. There's new updating. It's happening on an ongoing basis. And so the the issue here when we're thinking about now, data, harmonization and longitudinal. Rhoda Au: How are we gonna deal with the fact that the our tool that we're working with is a is something that continuously is changing in order to actually stay relevant. So I thought that this I, you know, thought this was a very interesting
Rhoda Au: graph that showed how many devices Rhoda Au: is the average person, at least within the Us. Using today. And not surprisingly, the smartphone is, is the most is the one that's most used. But if you look, there's actually a number of other devices that people are using, and because they're using all these devices. The question is is. Rhoda Au: how often are they updating it? And it turns out they're updating it all the time, including daily. So you have to be thinking about data harmonization. Now with the fact that the date, the tool that you're using to collect this data is changing and updating all the time. And that's obviously gonna have an impact on your Rhoda Au: on your harmony, your data harmonization efforts. Now, the other big problem with technology is that is the fact that it becomes obsolete very, very quickly. So here are both technologies that were were Rhoda Au: extraordinarily transformative when they 1st came out on the market. And some a number of these don't exist today, and there's some now on the verge of being completely obsolete. So that's 1 of the issues that you have to deal with not only just the rapid updating, but the fact that it may actually become obsolete in the not too different, not too distant future.
Rhoda Au: So this is the problem when you're trying to do large scale cohort studies that are longitudinal nature. And again, you're gonna need to continuously worry about the fact that the tools are changing the way in which is collecting the data is changing. And therefore your data harmonization is something that just never ends. Rhoda Au: So what I want to do is give you some of at least some of our experience in this realm, starting with Framingham and then extending beyond that. So, Framingham, I just want to remind everybody. Framingham started 1,948. We just actually celebrated our 75th year started with the original cohort of 5,124 people. They came in every 2 years. And just let you know our last cohort from a person from this cohort just passed away last year. Rhoda Au: Then their children and their children's spouses were brought in in 1971 Rhoda Au: and then we brought in actually a racially, ethnically more diverse cohort, smaller cohort to reflect the changing demographics in the town of Framingham at that time. That's the omni-gen one, and then in 2,002, we brought in the 3rd generation. So this would be the grandchildren of the original cohort, and then we continue to expand our racial, ethnic, diverse cohort as well. Rhoda Au: So you know, what did we do for many, many years we brought? We brought these people in for these regular health exams. The original cohort was every 2 years. The other cohorts have been every 4 years, and this is just to give you a sampling of all the different kinds of data that's been collected. Now, mind you, this has been collected since 1948, right on an ongoing basis. So you can also imagine the fact that the way in which we measured it also started to Rhoda Au: evolve over time. And then in addition to the core health exam. There's a number of ancillary studies, including the ones that I'm involved, which which are related to the brain.
Rhoda Au: So I just to give you a snapshot of the Framingham brain aging program we actually started formally through Nih funding in 19 actually, it was 1,989 was our very 1st nia funded, grant related to dementia, and then we have brought in the original other cohorts on top of the Rhoda Au: original cohort when we started this. So now we're looking at all surviving members across all cohorts of Framingham within the brain aging space. And then, most recently, we've had a U. 19 grant funded by the Nia that's continuing this work on an ongoing basis. Rhoda Au: So you know what I hope you're getting a picture of is how much data we're collecting, both through the health exams, through other ancillary studies and then through our own. Rhoda Au: And then on top of that we started to add digital technologies into Framingham. So one in 2,011, we actually started with the digital pen. And the reason that this was so transformative from a research standpoint is what you can see right now on the screen. This is this is now. Instead of using a regular pen. We now just simply substitute a digital pen. And now we're able to track a person's performance Rhoda Au: performance in real time. And what you can notice at the bottom is that there's different color coding that is representing changes in velocity as a person's going from one item to the next. So this is really getting at really granular levels of detecting in this case cognitive performance in a way that we've never been able to do before. Rhoda Au: We've also record, people's spoken responses to our neuro psych tests. And so we actually started this in 2,005 that we started recording, we have many different recordings. I think we have now over 12,000 digital voice recordings across our participants, including at baseline, when they were cognitively still intact, and some have now progressed to cognitive impairment, including diagnosed disease. Rhoda Au: And then and then, more recently, we've been moving to the smartphone application and that. And again, that's taking advantage of the fact that more and more particularly of the older participants, are now using smartphones. And now we're able to collect this cognitively related pay behavior in the comfort of their home.
Rhoda Au: So so now, what I wanna turn to is, how is all this data collection now translated into harmonization data harmonization. Because we're collecting this data on ongoing basis. And we have to actually integrate it. The other thing that we've been trying to do, because, despite our best attempts to be a little to bring in our omni, Gen. One and gem 2 cohorts. Rhoda Au: we're still largely a highly biased racially, ethnic cohort being predominantly non hispanic white. So one of the things that we've been trying to do is share our protocols very broadly and then work with other collaborators who have more diverse cohorts. And to harmonize it with our data. So this is something that we have to do on an ongoing basis. And this is just to give you an idea Rhoda Au: of how we're doing it now because we collect digital data, we also have to worry about, not just the structured data, which is what people typically think of when they're talking about data collection and data harmonization. We also have to think about now, unstructured data, which is the digital data, and that can include imaging as well as data from various devices.
Rhoda Au: One of the things that I would like to emphasize is when we do a lot of our work our harmonization work, and when we develop sort of the programming the processes to do it. We always do it with the mind of being able to share it, so that everything we do is open source. We share not only the data we share the all the code that we did when we process that data. Rhoda Au: And that's really important. If you're going to move forward in this kind of much more open science, because you don't want people to have to reinvent the wheel in terms of what did you do to process your data to get it to the format that is in right? So that's why we we share that. Now, in addition, I mentioned, you know, we have the digital data harmonization. And it turns out, digital data is very complex in terms of Rhoda Au: all its different dimensions. We have to de identify and Qc. It just like you would. But we also have to figure out ways to process it. And there's lots of different options right now for processing different types of digital digital data. But they're a fair amount of them are proprietary. So one of the things that we again really emphasize is trying to use open source toolkits to develop Rhoda Au: our processing measures so that methods so that we can share them. And then when we do work with industry partners. We actually ask to do it in a way so that we can share data. And they can provide us things that are non proprietary that we can share with the general community. And then and then one of the things that we've had to worry about is, how do you Rhoda Au: with then sensitive digital data? And I'm going to speak a little bit more about all this kind of digital data harmonization work. So so Rhoda Au: so as we all know, you know, one of the Rhoda Au: challenges when you're trying to harmonize data, particularly across multiple studies, is the fact that even you, you can even have very similar study protocols. So we've done a Framingham. We've been collecting the same kind of data for many decades, but it turns out we do it a little bit differently. And we actually were not really good at maintaining sort of the same naming conventions.
Rhoda Au: and that, of course, becomes much worse when you're trying to harmonize with other data with other resources. So one of the things that we've been trying to do is figure out, how do we do data harmonization much faster? Rhoda Au: you know, in terms of trying to get to these common data elements. And so one of the things we've been playing around with is this idea of natural language processing so with the Alzheimer's Disease data initiative that houses a number of these different data sets, we started to look at some of the data sets that sits on their end and started to figure out, how do we find a much more automated way to do data harmonization? So this is an example Rhoda Au: of 2 the jaros data sets. So they're you know, they're 2 different groups, one in U Europe, one in Japan. But it turns out that even though they are the same type of study, they're basically sub studies there, there's a lot of Rhoda Au: you know, there's a lot of differences in the way they collected the data, even though they have relatively the same protocol. And of course there's the way in which they stored it, which was different naming. So so one of the things that my colleague Jing, who? Who's been leading this
Rhoda Au: did is she decided to try a number of different natural language processing methods. So this is sort of examples of different kinds of methods. You know, doing large language models something that she, you know, it's called fuzzy Matching. And so there's all these different methods. I don't, you know. It's not to go into the details of each one of these methods, but to to try to see which of these different Rhoda Au: kinds of ways of doing things will actually allow you to get to much more much more quickly harmonized data across multiple data sets. Rhoda Au: And so so these are just some examples where she was kind of de determining which method actually worked the best when it came to variable matching. When you had all these discrepancies between the 2 data sets, and it turns out this E. 5 method that she had tried out was the best. That's not to say, this is the best. It's just an example of
Rhoda Au: how you can use name natural language processing to help facilitate something that tends to be a pretty manually intensive exercise. Rhoda Au: Now my colleague of Vijaya College, Coloma, has taken on the challenge of image harmonization. So we have, for instance, been acquiring MRI images at Framingham since 1999. And and what's happened is the MRI machines. Of course, technology has evolved. So today, you know, state of the art is the 3 Tesla machine. But we started with a 1 Tesla machine. And then we also Rhoda Au: in between that used a 1-five Tesla machine. So we're now trying to study changes in people's brain structure over time. But we keep changing the mapping strength of the machine because that's what the technology state of the art is doing so. So Rhoda Au: so there's been a lot of work done around sort of this concept of imminent harmonization. But one of the things that like of the G. Vj. Is trying to do is think about rather than think about. How do we harmonize in the more traditional ways that have been out there for imaging harmonization. What happens if we just started with the raw image itself? Rhoda Au: So rather than processing it, you know, trying to extract features, for instance, like free surfer, and then harmonizing and and then always anchoring it to some baseline, etc, because that's all very time consuming his idea is, how do we just take the raw images themselves and use sort of deep learning methods in order to push that forward. So he's actually published in a number of
Rhoda Au: studies that you can take a look at at different ways in which he's been thinking about doing harmonization. But the most important thing to take away from this is that he's always starting the raw image. So he's not doing any processing of that image in the way that's traditionally done. Rhoda Au: And so one of the things that he's been trying to figure out is, how do you take scans that were Ca collected longitudinally off of different kinds of magnet, straight machines, and make them comparable. And so he used this sort of machine learning method in which he takes 1.5 t images. Rhoda Au: and he actually made them comparable. So he's kind of increased it. He sort of use this game theory inspired machine learning approach, and he made them comparable to a 3 t. Like image, and in that way he can sort of equate that. So this is a very different approach to thinking about image harmonization which is to alter the image itself
Rhoda Au: and using machine learning and harmonize it to the equivalence of a later technology. Rhoda Au: So this is just a very quick summary of sort of what is the whole image processing pipeline that he's put together? But I think, what's important to take away from this is that a lot of this work that he's been doing is automated. So he tries to develop hands free methods. Because again, you don't wanna you wanna get away from the manual labor, intensive harmonization methods. Rhoda Au: So then I wanna shift to sort of digital voice. As I mentioned, we've been collecting digital voice for since 2,005. And so what happens over time is that the voice recorders change the quality of the you know, the devices themselves, and the fidelity in which that they can record has been shifting. Then you have differences in the voice quality. It's Rhoda Au: you know, as a Rhoda Au: as as the fidelity changes, then it changes the the quality of the voice that you're obviously analyzing. And then there's the fact that when you're collecting digital voice across many different cohorts you have things like Rhoda Au: they. They speak different languages. For instance, because you're trying to collect data in their native language. And that's the advantage of digital voices that allows you to do that in people's native language. But then you have the problem of how do you harmonize that data? Even within the Us. Within English speakers we still have differences in in the voice. When you think about. Rhoda Au: for instance, in some of the Southern States where they have a Southern accent here in Boston, where I'm at, we have this East Coast East Boston accent down in Texas. So if you think about across the Us. Even among English speakers, there's going to be differences in the voice in speaking it.
Rhoda Au: So one of the things that we've been thinking about is, then how do you harmonize across? All these different entities you have to worry about whether there's background noise. You have to worry about the quality of the audio. And so we're using again, different open source automated processing tools in order to try to Rhoda Au: to to take different audio recordings and make them much more equivalent similar to how Vijay was trying to do it with the images, and then we use open source tools in which to try to extract features as well. Rhoda Au: and then on the, and then here are some additional back end kinds of things that we'll do. There's actually a lot of different kinds of techniques in which you can go in either to process the audio file in their raw format, or to extract acoustic linguistic features. I think what's important in the digital voice harmonization world is is that this is all very new. So there aren't any gold standard. Rhoda Au: So one of the things that we're doing right now is we're actually experimenting with different kinds. And part of the reason that we do this is because different kinds of analyses require different aspects of the digital voice. Rhoda Au: So in thinking about, you know, how are we gonna move all these things forward. As I just mentioned, there are very little gold standards when it comes to digital. So one of the things the only way in which we're gonna accelerate. Both the harmonization of the data which is going to be necessary in order to push for the science and then keeping in mind that the digital data now is going to be changing
Rhoda Au: all the time, for all the reasons that I talked about right? So how are we gonna actually make this happen quicker? And the only way I can think of to do that is to share the data. Rhoda Au: And so so we work with the Alzheimer's disease data initiative. A lot of the current interoperability platforms actually can't take in Digital data and certainly not digital data in its raw format. And so so we work with the Addi in terms of pulling the data in you know, they provide sort of a secure enclave and then being able to push it out to researchers wherever they are. Rhoda Au: The other thing that we're doing is we're working with the global research and imaging platform. This is a nonprofit entity. And we're trying to now build a lot of the Rhoda Au: collection processing and analysis tools that you're gonna need to do all the kinds of work, the data harmonization work that I just talked about. And what again, we're trying to do this in an open source way, because everything we do under grip is supposed to become publicly available, accessible Rhoda Au: up.
Rhoda Au: So I did wanna take a few minutes just to touch upon some Rhoda Au: data. Harmonization challenges. When it comes to sensitive data. There's you know. Certainly in the past, we certainly know about genetics data. But now, as we're going to the digital realm, you know, ocular scanning has become very popular, you know, doing retinal scanning as a as a non invasive biomarker has actually, Rhoda Au: has been starting to emerge as an alternative to some of the much more expensive biomarkers. They, you know the link, you know, to the brain relative, the eye, the eyes really giving a window into the brain. But, as we all know, retinal imagings are then images themselves are identifying their retinal, imaging us devices all over the place that are used now to identify you, your person. Rhoda Au: and then a digital voice also has the same issue of being personally identifying. We certainly have all experience. You hear someone you hear a voice. And you think, Oh, yeah, that's that person. And so it's inherent into that voice print that there can be a sensitive data. So the question now is is, how do you do harmonization? The kind of work that I've just been talking about when you have such sensitive data. Rhoda Au: So this is work that my colleague Cody Karjati, is doing with the Alzheimer's Disease data initiative we're doing at Framingham. And we're really trying out. Now, these new federated data sharing
Rhoda Au: approaches. And so really this idea that you can kind of put a firewall to protect the des, the sensitive, I identifying information you can create, secure, enclave in order that people can work with it. And so these are alternatives to, you know, sending out data, because once you send out sensitive data, you kind of lose control and and then there's, of course, the the issue of Rhoda Au: then when you lose that kind of control, how do you protect privacy and confidentiality? So I think that this federated data systems are becoming much more interesting, particularly as you start to go globally where you have governance issues. You know there are countries that do not allow their data out of the country. And so these federated systems are the solution, because that's the only way in which we're gonna be able Rhoda Au: to bring data. All this disparate data, different kinds of data formats, different data from other countries. You know, how are we gonna bring it together in order that we can harmonize the data in order. And to get to that sort of much more representative data resource that we need to push our science forward. So this is an example of sort of what's the architecture for? How to do this? Rhoda Au: And again, you know, providing sort of these secure Enclave, letting people work with it or the other way is to send in your scripts. Let them run it, and then push out the data to you, the de identified data. So as I, I'm sort of wrapping up here. Rhoda Au: What I wanted to do is is mention that, given how different. All this is we have to be actually really careful about who we think we can invite to help us in this harmonization challenge. And really, the goal here is we really need to learn to work with everyone
Rhoda Au: you know, I like to, as we're moving down this path. I I like to encourage people to think different and to not just think different, but also be different. And then, finally, I think the other thing that I like to mention is, is that all this work is actually really, really hard. And so the biggest messages? We really need to make sure we never give up. Rhoda Au: And so with that I thank you and and I'm happy to take some questions. Karyn Onyeneho: After. Oh, thank you so very much. Hopefully you can hear me. Okay. Karyn Onyeneho: What a what a wonderful presentation! I greatly appreciate many of the insightful and thought provoking discussion points that you had covered during your presentation.
Karyn Onyeneho: I would even say, especially the importance of Cde Linkages, and how to use effectively digital technologies, not just thinking about where we are currently, but also in the future. And how do we adapt that to our global users? Right? So that we can actually do data harmonization Karyn Onyeneho: so that was really great. We finished a little bit early, which is actually a good thing, because we can now allow about 10 more minutes towards our QA. So right now we are going to turn turn our presentation over to our QA. Segment. Karyn Onyeneho: because we want to answer some questions from our audience. Karyn Onyeneho: We hope that during Dr. O's presentation you were engaged. I know. A few questions came in Karyn Onyeneho: and so I will make sure that I address as many questions as possible those that were sent over that we don't get to cover today as usual. Make sure that we answer them and send those out all of those who've registered
Karyn Onyeneho: for today's lecture. Karyn Onyeneho: At this time. I do encourage that in the next 30 min or so I think. Please populate any questions that you may have for Dr. O. Into the QA. Window which is at the bottom of your screen. Karyn Onyeneho: So let's get started. Dr. O, I wanna ask one of the 1st questions I was interested in is, could you? Could you share with us? You know, some strengths as well as limitations associated with common data elements that one should bear in mind. Rhoda Au: Sure. So I mean the obvious strength with the you know, common dated elements is, it's in its words, right? Right? It's the fact that we're going to bring together measures that are similar in a concept.
Rhoda Au: and find ways in which to make sure that they overlap right and that they are harmonized. But I think part of the problem that happens when we go down this common data elements route Rhoda Au: is that in trying to mix and match these different variables, which, whether they're coming from different cohorts that have measured the same thing same at measure of interest, or, if you've done it longitudinally, and it's changed over time. Right? So those are so those are. That's the goal is to get them Rhoda Au: equivalent. Rhoda Au: But the but what happens in reality is when you do that. Rhoda Au: you actually reduce Rhoda Au: your overall variable Rhoda Au: data set right so you can take. So you'll take studies that have thousands and thousands of variables and and what's great is when you harmonize them. Now, you're able to easily facilitate analyses right? But what you've lost
Rhoda Au: is you've lost a lot of the variables in the process. So you end up coming up with really a pretty minimal data set overall. So you've lost the power of all the data that you've collected. Rhoda Au: So that's so. Again, I think that the strengths are that you can push forward analyses at least using sort of what we have are the traditional epi epi and bio stats tools. But in the process you you have, I would say, watered down the science that you're now able to pursue. Karyn Onyeneho: It's actually, really, really important. So thank you for addressing, you know not just what those limitations are. But how can we actually mitigate those? Maybe the next question I have for you is could you explain us? If there is truly a difference between the concept of data, harmonization and common data elements or Cds. Rhoda Au: Yeah. So I think that I think we often think of the 2 as being equivalent. And what I would like to actually encourage people is to think about them as being different. So C-d-es are just what we describe right? Traditionally, we try to get to that common denominator. But then I explain to you what we lose in the process. And it turns out that now that we have lots and lots of different kinds of analytic tools, the idea of harmonization is actually much broader. Rhoda Au: So, for instance, you know my colleague Vijaya cola Talma, he he! He never wants anything that's drilled down to a common, anything right? He likes to say. Just give me all the data. Give it to me in all its messiness. And with my data science AI methods. I am gonna figure out how to harmonize them sufficiently Rhoda Au: for my kinds of data analytics. So I really think that one of the things that we need to start considering is, it is what is our broader definition of what we mean by harmonization, and it turns out that harmonization is probably different, depending on what your analytics strategy is. And that's really at least for me, you know, that's what working with the data science. AI,
Rhoda Au: it's really interesting moving into sort of that big data realm. And they always talk about messy data, right? And you know, and and I've now understood what they really mean by messy data is not necessarily data that's been collected. Rhoda Au: Poorly. Rhoda Au: but rather, they're talking about, you know, data that's been, that's, you know, followed scientific protocol standards, etc, but is messy because it isn't in a format that easily translate into what we consider a common data element. Rather, they say you give me all this well collected data that is mismatched, and I will be able to figure out how to manage it. Rhoda Au: So I that's why I worry about when we say, Oh, we gotta harmonize this data. There's actually levels of harmonization depending on what your analytic strategy is. Karyn Onyeneho: Yeah, I really appreciate your insights there, right? You've also addressed not just the issue of mixing and and matching but also the missingness. And I think over time, I'm thinking, you know, with the changing demography of the United States like you talked about earlier in your presentation. That is something that we have to be very, very mindful of, especially if some of the data collection that we want to in the future harmonize. Includes you know
Karyn Onyeneho: how we understand or how we define. Some of those data or metadata in relation to, for example, race or ethnicity or ancestral groups. Karyn Onyeneho: So I appreciate you sharing that and also thinking about how all of that would be contingent upon developing an actual analytics strategy. So thank you for actually differentiating between the 2, because oftentimes they can be conflated. And that's a question I have for you is, you know, could you? Could you share with us. What should someone who's designing a longitudinal study that will span Karyn Onyeneho: over many years? I can think of one already, such as the Nih. All this research program. Who's hoping to go 10 years, if not more, in the research that they're doing. But thinking about that. You know what? What follow up strategies? Should one bear in mind who might be involved with such a study in relation to the context of data, harmonization. Rhoda Au: Yeah. And so this is also in the context of digital technologies. Right? So we're trying to think about, how do you doing a large scale cohort. Study longitudinally with the fact that I just told you digital technologies are changing all the time. Right? Rhoda Au: And so you know. So one of the things that I
Rhoda Au: try to really emphasize Rhoda Au: is that, regardless of what you collect. Rhoda Au: I don't care what device. I don't care. You know what measure. You know what what the derive measure is. What's really important is to collect the raw Rhoda Au: data itself. Rhoda Au: because that's what's gonna help you future proof in the in the future. And so what happens with digital data
Rhoda Au: is that it's easy. It's relatively easy to collect low cost right? Multi sensor, particularly if you're doing off of a smartphone, for instance. It's also really easy to store. Rhoda Au: Right? You can do and and then what happens is is that over time as these algorithms continue to change and they'll change like an algorithm changes. Probably you know all those updates that they're talking. They're just changing the algorithm all the time they're trying to figure out how to make it better and better and better. So what happens is, if you only go with the drive measure. You're stuck with the drive measure that was measured. Rhoda Au: That was the best algorithm at the time. If you collect the raw sensor data that's being analyzed. Rhoda Au: then you can go back and you can re analyze it and bring it up to contemporary standards. Right? So that's what I try to tell people is, if you really are going to do this longitudinally, it's going to be really important to make sure that you get the raw sensor data, or else you can find yourself. You know you're going to be obsolete very quickly, because these algorithms change and fit. You know many people are Rhoda Au: familiar with the Fitbit. If you think about the 1st version of the Fitbit people complained about the fact, how inaccurate was in in calculating your steps, and over the years. Now, it's gotten more and more accurate. Right? So what happens if you had collected that data way back when you would have actually relatively inaccurate data relative to now the new data. So this is one of the ways you have to guard against the other thing you have to guard against, and particularly if you're gonna work with
Rhoda Au: companies and their proprietary devices, etc, is they they are. Rhoda Au: They're gonna go out of business. They're gonna go in a different direction. They're gonna become too expensive. They're gonna become obsolete, you know, there's all these different things. So one of the things that's really important. When you're designing your study, you you you can pick vendors. Rhoda Au: but you have to not design your study so that you're dependent on that vendor. And that's again, with this idea of raw data comes in, you know, when I collect raw digital voice, it doesn't actually matter Rhoda Au: whose device you know, whose application I use as long as I maintain that raw digital voice recording. So that's why the raw is so important when you're thinking about sort of doing these long term. Karyn Onyeneho: Yeah, thank you. That's greatly important. And I like the example that you use with the fit that I can immediately think of. Also the apple watch. How many times Karyn Onyeneho: they do request updates, which may be overwhelming. But I think to your point, it's incredibly important, especially in the space of aging. When we think about how that
Karyn Onyeneho: information, for example, it could be information being collected during your sleep. Karyn Onyeneho: You know, if not the raw data that's being collected and can be accessed in point. How gosh! If we lost that data over time, how that could really Karyn Onyeneho: I think, create many missed opportunities that threaten our goal to improve human health. So thank you very much for emphasizing the point of preserving raw data. I think that's also important. You know, earlier in your presentation, you know, you discuss with us how traditional approaches
Karyn Onyeneho: for research limits research. Karyn Onyeneho: From a global perspective. You know you also address the importance of diversity through various categories, such as race, ethnicity. Karyn Onyeneho: income, geography. But you also mentioned as a part of that diversity dementia at the earliest stages of life, right, which is oftentimes something that we don't really think about in inadvertently you were very thoughtful about discussing that as a part of you know, a global solution. That includes quote anybody anywhere. Right? You also discuss, you know, the use of digital technologies and additional technologies
Karyn Onyeneho: that could be used, for example, between low Karyn Onyeneho: medium and high Karyn Onyeneho: research enterprises. While bearing in mind those diversity targets, are you able to share with us other aspects of digital health technologies that we can use for data collection. I know, met you mentioned earlier what Adney is doing and other projects such as leads to help us fill those to help us fill some of those gaps. Rhoda Au: Yeah, I mean, I I well, I think that. Rhoda Au: So this probably goes back to my my earlier response as well. Right? So if we think about this, you wanna be collecting data across the life course in this case. Rhoda Au: Without being depend dependent Rhoda Au: on any one technology, on any one device on any one algorithm. So that's going to be really critical. So I think it's, you know, for me in thinking about, how do we do this, I do
Rhoda Au: tend to focus on the smartphone or the tablet. And the main reason is become from a global perspective. It, again, is the most penetrating technology. And it is essentially a computer and every person's hand that's able to collect on an ongoing basis. So Rhoda Au: and one of the things I think that it allows us to do then is it allows us to do it in the very young, because now we see that people who are very, very young, right? We see young children already, who are able to use tablets and smartphones in order to help collect the data. Rhoda Au: And then what you have to think about is what kind of data should we be collecting? Rhoda Au: So typically like with a cognitive test, we want to administer neuro psych test right? And that neuro psych test will shift and change in terms of the elements of what you could test. Someone who's 10 years old versus 20 versus 50 versus 90, right? Rhoda Au: If instead, we think Rhoda Au: about the fact that everything we do Rhoda Au: we do through our brain.
Rhoda Au: So everything we do, we're always emitting our cognitive capabilities. Rhoda Au: So we don't necessarily have to test cognition. We can start to infer it. Rhoda Au: So I mentioned digital voice, why am I interested in that? Because it's a cognitively complex task, and over time. Rhoda Au: through someone's speaking, I can track their changes in their cognitive abilities over their lifespan without changing Rhoda Au: the without having it be test specific. Right? Well, turns out that there are other measures that also reflect this. So when we when we're moving about our environment right when we eat, when we sleep, when we when we engage, when we are socially interacting, it's the same thing. We're always emitting these behavioral symptoms. So rather than testing them, we should be using these multi sensor.
Rhoda Au: You know, capabilities to bring in this digital data. And from that extract the behavioral functions of interest rather than measuring them through tests. So so it's we have to rethink. Rhoda Au: how do we measure these things? And what I like to do is mention. You know, we kind of do this already. Naturally. Rhoda Au: So if you, if you have someone complaining that they're having difficulties right, I'm having memory payment, etc, they come into their physician's office. One of the 1st questions will ask you is, Rhoda Au: When did you notice your symptoms? Rhoda Au: And the person might say, Well, you know, it's date back. I noticed a few years ago I was having trouble driving. You know. I got lost. I missed some bills. I forgot to turn off the slow, etc. Right Rhoda Au: then you ask their family member Rhoda Au: and their family member will give you a different set of examples, and if you ask another family member, they'll give you yet another different set of examples. Now here's the thing with all those examples, particularly in the early stages. They're not constant. You don't always forget
Rhoda Au: right. You don't always forget to turn off the stove. You don't always forget where you're going. You don't always you know, get lost, etc. Right? So so there's nothing static about what we're doing. So we use these static instruments to try to measure these measure these behavioral symptoms of interest. Rhoda Au: But in reality we're able to pinpoint it through these observations of natural behavior, right and and and it's actually far more accurate often compared to these static instruments. Rhoda Au: which I think are kind of. You know, they're kind of crude instruments. So this is really where I think that we need to be thinking about. How are we able to do that? And how then we can do that across the lifespan. So it's collecting data in a different way and thinking about how you can analyze it and pull out information rather than because we don't go around testing our family members right? And yet we we can observe it. So that's what we Rhoda Au: need to do digitally. We need to do what we already do naturally. And now we need to do it objectively with these instruments. Rhoda Au: so I hope that. Karyn Onyeneho: You.
Rhoda Au: Question. Karyn Onyeneho: It. It absolutely did. And I could immediately now think, even over time, how the Internet of things for IoT is gonna increase in popularity and how that will impact. As you mentioned, how are we thinking about collecting data? Retain retaining that data? And it's Ross form. So thank you for that. But we do have an another question. Before I ask Karyn Onyeneho: our audience was very impressed Karyn Onyeneho: by your presentations. I just wanted to give you those kudos as as I as I am as well. So one of the other questions that's being asked is from an administrative perspective. The question is, you know, do you have full time technical staff
Karyn Onyeneho: who actually work on data harmonization or processing or even data security? I know with data security, as all of us know. It is incredibly important now, right? When we think about the sensitive health data that is not only being collected but but shared. Could you speak more on that. Rhoda Au: Yeah. So you know, the Framingham Heart study is kind of kind of a large enterprise. Right? So we're funded. Actually, our brain aging program. You know, we're testing thousands and thousands of participants over the course of, you know, 3 to 5 years. So in this case, we do now currently have a dedicated data core that's working on this and includes Rhoda Au: I'm it includes for a staff perspective. It does include some full time staff from a faculty level is is still remains sort of, you know, part time, effort, but they're overseeing this. And I think that's really important. In general, as we're thinking about. Rhoda Au: You know, there's a lot of discussion about data sharing. There's a lot of interest in data sharing. And I think one of the messages is. It's actually, it's it's a lot of hard work to share data. It's actually hard to share data. If you've made it your commitment to share share data which we have on our Framingham brain aging program. It turns out it's actually really hard to do it. Rhoda Au: And so consequently, yes, you do need to have some dated dedicated effort. That's probably a different message for the Nia. To think about as they're funding these different projects is what are the resources that are being given to different projects. You know, different projects scaled right? It's gonna be scaled. If you have a small scale study, obviously don't need as much dedicated effort. But if you have these multi 1 million dollar per year enterprises, then you are
Rhoda Au: so so yes, so it it takes a lot. And and with digital because it's ongoing changing in the way that I just talked about, it's on an ongoing basis. Karyn Onyeneho: Thank you so much, and I hardly agree with what you've said. Another question that we have is, could you talk to us about. You know, the artificial intelligence and machine learning in terms of approaches and modifying Karyn Onyeneho: I'm gonna reverb with the question is saying, modifying low to high. I'm assuming imaging data to ensure that the data are correct. I know earlier you talked about the importance of image harmonization and some of the efforts that are being done. I believe, in in Japan and in Europe. Karyn Onyeneho: If you could talk more about that, that'd be great.
Rhoda Au: Yes, so I'll say Hi to Marilyn. And for this question, and yes, so I think what you're talking about, Marilyn, is the fact that you know the resolution on a 1 t scan is different from a 1.5 t scan from a 3 t scan. So how? How you're able to do that, and you know I will tell you the main thing you have to do on that rigor reproducibility. You actually have to acquire data on all those machines within the time, same timeframe on the same person. Rhoda Au: So we were fortunate that Abney did that Rhoda Au: right admin, because admin was transitioning. So this is great that the Ni funded Agney to do this on the side was to there was a subset of participants who are brought in, and they were actually measured on the multiple machines with, I think it was even within a 2 week period or something. I don't remember those details, but was in the same timeframe with, you know, same kinds of sequences. Rhoda Au: different magnet string. And that's how actually my colleague Vijay, was able to build sort of these, and then you can sort of do some of the Rhoda Au: you know confirmatory work with some of the other images that we have from other data sets. Karyn Onyeneho: That was great, and thanks for the question.
Karyn Onyeneho: You know, earlier in your talk you also discussed the reality of the technology life cycle. This includes the importance of those timeframes. Even the software updates in the example with Fitbit and even the apple watch because of these releases that can happen very often or not as often as they should. Karyn Onyeneho: In this regard as we think about data harmonization, one of the solutions and addressing that would be, you know, understanding that it is a changing paradigm. Right? We're in the tech technological error, and that we have to know that many devices that are being used today will change or become obsolete. Right? And how do we deal with that? You know? What other potential changes you know? Could we expect in the future? Given this rapid movement of technology as it's Karyn Onyeneho: changing and and how could this impact the data harmonization efforts in research for the future? Rhoda Au: Yeah. So so I've talked about sort of the need to make sure you collect raw data. Now, now, I'm gonna be realistic, too. You you're not necessarily always gonna be able to do that. So what's gonna be very important is probably to always have a set, you know, subset studies that did exactly what Admin did on the imaging side. So I know that there's a number of groups, and that's what they do. They collect. For instance, they'll collect sleep
Rhoda Au: data off of different devices in order to figure out, how do you harmonize across the different kinds of devices so that can be both within contemporary time, because there's lots of different devices now that collect sleep, for instance, or it could be longitudinally, as these devices evolve. So so one of the things that's gonna be really important is if you can't, particularly if you can't get the role. Rhoda Au: Raw data itself is that you're gonna have to at least do it on a subset and create those the data sets in order that you can do those real time comparisons right? You'll you wear the old Rhoda Au: version, you wear the new version, and then you have to harmonize in that way and do those kind of correction factors. That's really about the only way we're gonna do that. But that's me thinking about it from a regular, you know, kind of traditional data harmonization standpoint. I I suspect my data. Science, AI colleagues would would say, I'm probably worrying about more details than are necessary. Rhoda Au: So I know I I will have to say this, my colleague Vijay there! He often does a lot of these different steps, not because he needs to do it from a scientific standpoint, but it's like what Marilyn said. He needs to demonstrate the rigor reproducibility because the scientific community demands it from his perspective. If he could just be left to run on his own. Rhoda Au: He! He! He feels that you know the data science methods that he has doesn't actually require that he does this. But this is so he's. It's a compromise between what our technology allows us to do and what our science requires us to do. Karyn Onyeneho: Yeah, that was really great. I know we have only a few minutes left. I wanted to ask maybe one more question before we launch our poll to our audience. Karyn Onyeneho: and we'll hope we hope the audience stays tuned. You know you talked earlier about the importance of open science, which I wholeheartedly agree, you know, and using open source toolkits that enable sharing data more efficiently. Including partnerships. Right? And and how have having those partnerships are important? I really appreciate your your thoughts there? Could you talk more about just really the importance of open science and in relation to this topic today on data harmonization
Karyn Onyeneho: within maybe 60 seconds. Rhoda Au: Sure, I mean at the it's kind of like what I had said. We we need to accelerate science, you know we we our goal is to get there faster, better, quicker, cheaper, right? And that's what technology is supposed to allow us to do is really to compress those timelines. But if we if because this is still so new. Rhoda Au: the only way we're going to be able to accelerate this is to be able to data share. So imagine right now, there's lots of ways in which we're still working on how to effectively de identify. If I provide you my source code, right? That I've done. Maybe I've done a kind of Rhoda Au: pseudo good job, but not good enough. But if I put it out there, if I put it out as a data challenge. Then anybody, you know, we can invite talent everywhere. Right? That's what I was trying to say is, we don't want to be prescriptive with who we work with. Let's take anybody anywhere right that can come together and help take what I did and make it better. And then let's make it better. You know, I often use space exploration, you know. Rhoda Au: as my inspiration, because they set goals with no roadmap. Rhoda Au: So how do we create that roadmap? We could create it through data sharing. We share the data. I give it to you. I give you my codes, and then you take my code. I don't want you to start all over. You take my code, you make it better. Then you share your code, and someone else comes along, and they can make that better. And that's why it's really important. And because we're trying to do this.
Rhoda Au: this is a terrible disease that we're trying to deal with Rhoda Au: what we should be focusing on is, how do we get to some sort of solution as fast as possible? And we're gonna do it as a village rather than as an individual. Karyn Onyeneho: Thank you so much, doctor. Oh, what a great way to end today's session! I wanted to just thank you for joining us to our live audience more importantly, Doctor. Oh, thank you so much for your expertise, for the stimulating engagement and sharing insights for today's talk. Help us advance the field of Cds and data harmonization. All of you who are left, please. We'd like, if you could look at the questions on the screen. We hope in the future to have more stimulating talks Karyn Onyeneho: from Dr. O. And others who've been a part of the Speaker series. So let us know what aspects of today's lecture. Did you find most interesting, as well as the topics that you might want to see in the future? With that I just wanted to thank again our esteemed speaker, Dr. O. For taking the time today, and really just making us think really insightfully about some of what Karyn Onyeneho: is happening today in the digital health space and what we ought to think
2024-07-26 20:11