CITRIS Research Exchange: Steven Johnston on Bioconvergence -- Transforming How Humans Live and Age
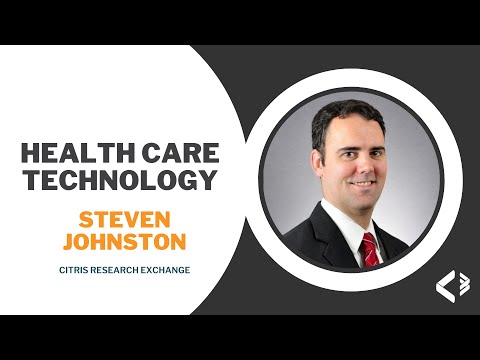
- Good afternoon and welcome to the "CITRIS Research Exchange." My name is David Lindeman, I'm the Executive Director for our CITRIS Health Program and I'm delighted to welcome you to again, our 14th year of the CITRIS Research Exchange Seminar Series. We've hosted a remarkable lineup of technology innovators in-person at Sutardja Dai Hall over the years, and we're glad to have everyone join us today virtually as we continue the history and tradition of this program and through our Spring 2023 series.
Before we begin our talk today, I'd like to make sure we highlight a few upcoming events that CITRIS is hosting. Of course, our next CITRIS Research Exchange will actually be held in two weeks and Wednesday, February 22nd, same time, 12:00 to 1:00 PM and the topic will be, "Unraveling the Computing Bottleneck for Autonomy" by our own Ricardo Sanfelice, who's Professor of Electrical and Computer Engineering and Director of the Cyber Physical Systems Research Center, as well as our director of CITRIS Aviation at UC Santa Cruz. So please be sure to check the CITRIS webpage for registration details and further updates. We look forward to you joining us in two weeks. Furthermore, we are delighted to announce the Edge in Tech Program, CITRIS and the Banatao Institute's Berkeley and Engineering Program will be held on March 2nd.
And the topic this year is "Smarter Tech for Resilient Future." This will be a virtual symposium highlighting the experience of experts using emerging technologies to advance innovation for a more sustainable infrastructure. Again, a fantastic opportunity. We look forward to both students, faculty, and colleagues joining us for this program. And we encourage you to go to the CITRIS website to register. So, for today's program, we'd like to, we're doing a little bit different.
We would like for you to share any questions you would like to offer the speaker in the Q&A box. However, there's so much excellent material, we will not be responding to Q&A during the program, but Steven has kindly offered to respond to individuals via email and be able to answer your questions in the future. So, please share anything you have with us and you will also be offered his email for direct communication.
So without further ado, I'd like to share that we are delighted to have Steven Johnston, who holds dual roles at Merck, KGaA in Darmstadt, Germany, as well as the MD Electronics as Vice President and Head of Technology, Transformation and Enablement in the Electronics Business Unit, as well as Vice President, Head of Technology Scouting and Ecosystem Enablement in the Corporate Science and Technology Organization. In his role, he's responsible for leading Merck KGaA's electronics engagements, not only internally with Merck's Healthcare Group and Life Sciences business units, but is able to work with external partners to enable technology, converge and differentiated product development. In addition, he is part of Merck KGaA electronics leadership team, and he's responsible for defining their strategic engagement plans with the semiconductor ecosystem. So in his Corporate Science and Technology Organization role, Dr. Johnston leads Merck's, KGaA enabling technology scouting organization to identify and launch disruptive technology and new business growth programs, and they span electronics, healthcare, life sciences, ecosystems, and other product areas. We're thrilled to have Steven Johnston join us today, and you're in for quite a wonderful program.
Steven, the floor is yours. - Excellent. Thank you so much for that very kind introduction and good afternoon to everyone. I very much appreciate the opportunity to speak here at the CITRIS Forum and talk about what Merck is doing in the bioconvergence space. So as I pull up my materials, as I was looking, thinking today about how to frame my talk, I was debating, do I go deep in one particular area or do I go broader, but maybe potentially more shallower? And assuming that folks are joining from multiple types of disciplines, multiple backgrounds and domains expertise, I decided to go for the latter.
But as David was saying, be happy to have any follow-up conversations with anyone. You can see my email address on the bottom of the screen here to have deeper conversations on any of these topics that I'll be talking about. So as I look at bioconvergence and what it means for all of us going forward, I somewhat liken in my mind to autonomous driving in the sense that just as electronic and automotive technologies have converged to enable the electrification of vehicles and the early stages of autonomous driving, I believe that digital technologies and biology will increasingly combine over this decade and beyond to transform how humans live, age, and interact with the environment around them. So in the next 45 minutes or so, I'd like to highlight some of the work that Merck KGaA is doing to make bioconvergence a reality for all of us now and going into the future. I liken back to a quote that Steve Jobs once said, I think that the biggest innovations of the 21st century will be at the intersection of biology and technology. A new era is beginning.
I fully believe this and I'm amazed at the vast potential that bioconvergence offers humankind to advance how we live and age. And I believe that Merck, KGaA as a global leader in life science, healthcare, and electronics is uniquely positioned to drive this new technology frontier forward in close collaboration with ecosystem partners. So before I get into the technical details, let me spend a few minutes giving you some background on who Merck KGaA is and it helps set the stage on why we're so passionate about scientific breakthroughs and the bioconvergence opportunity specifically. So Merck has a 350 plus year history focused on curiosity. You can see the company was founded in 1668 in a small pharmacy in Darmstadt, Germany. And over the years, over the centuries, we've grown in terms of our focus and our capabilities.
And today, we're a roughly 25 billion market cap company with researchers and manufacturing capabilities around the world. We are unique. Within the US and Canada, we go by the names EMD Serono, Millipore Sigma, and EMD electronics. Outside of those two countries, we go by Merck KGaA. And since our founding over 350 years ago, we've truly become a global player with more than 60,000 employees operating in 66 countries, working on breakthrough solutions and technologies across the life science, healthcare, and electronics technology areas. And I believe it is this unique combination of life science, healthcare, and electronics all underneath one corporate umbrella that truly makes Merck KGaA uniquely positioned to drive this bioconvergence revolution that we'll talk about.
We're a global leader in each of these three segments and the combination of these technologies coming together is going to generate products that frankly, a few years ago, I don't think people were able to really comprehend were possible. So let's dive a little bit into the bioconvergence and let's start by looking at the human body. It's an absolutely fascinating specimen in science. The human genome consists of roughly, one and a half gigabytes of DNA data and there's roughly 15 trillion cells in the body. So you multiply these two together and you get roughly 23 zettabyte of DNA data that's inside of each of us.
Now, if we compare this to 2021, the overall amount of data that was generated in the world was estimated to be around 79 zettabyte. So within each of us is roughly one third of the amount of data that humans and machines generated together over the course of the past year. That includes all the videos, the emails, the IoT devices, and many, many other data streams. Looking forward, data generation is projected to grow to roughly 175 zettabyte in 2025, a very healthy increase of a hundred zettabyte over four years, but still that's only four people's worth of DNA data. So the collective information just inside the people joining us here today, vastly out numbers this global datasphere.
And my point in calling out these numbers is not to trivialize the amount of the size of the global datasphere, but rather to point out the enormous opportunity that's in front of us all to use the human body's vast amount of data more effectively. To do this though, requires us to first harness insights from the vast amounts of data that's within each of us, and ask the questions of how do we use these insights to fundamentally improve not only healthcare outcomes for broader society, but the personalization of these outcomes. And essentially is how do we make sense of this vast amount of data generate insights and correlations that would previously gone undiscovered and individualize these understandings to improve patient outcomes? The answer in my opinion is bioconvergence and the simultaneous deployment of advanced biology insights with big data insights powered by artificial intelligence and adjacent science disciplines like material science.
So much of science advances in evolutionary steps where one discovery will incrementally build on the insights of its predecessors and takeover, build the overall capabilities one step further. But with bioconvergence and the unique combination of scientific disciplines merging together to make it a reality, I think we're on a cusp of a much more revolutionary advancement. And to me, bioconvergence offers this opportunities, the possibilities of being as revolutionary society today as the assembly line was and the computer were decades ago. In the coming minutes, I'll give you some examples as to why I believe bioconvergence holds such promise for humankind.
So bioconvergence is going to open new opportunities and we envision that it's gonna unlock the personalization of medicine that is tailored to each of our unique molecular signatures. So treatment and aging plans that are unique to our individual genetic makeups, metabolism and diets, environmental surroundings and many other variables that make each of us, us. And also, unprecedented connectedness to everything and everybody. So simply put, it will fundamentally enable human to human and human-machine engagements that seemed like science fiction when we were all children. So this exciting thing is this revolution and the myriad of opportunities that it offers us has just begun, but no doubt, it will and it is transforming society as we know it today.
We at Merck KGaA define bioconvergence as a multidisciplinary approach that harnesses the synergies across data, digital and material science, as well as biotechnology to ultimately improve the speed and impact of scientific discovery. It holds the promise to enable highly personalized medicine, as I mentioned, a hundred fold or more efficiency increase in drug discovery that we'll talk about in a few minutes. Vastly improved healthcare treatment efficacy, and even broader incorporation of how the human brain works to guide the future of compute.
And we'll touch on many of these as we go through this hour. So if we take a couple steps back and say, what are the fairly high-level definitions of bioconvergence, the question starts to become what are the specific examples of science where this manifests itself? Why are these new areas of science emerging now? And what tools do we have available from a business and ecosystem shaping perspective to bring this concept to life? So one way to look at these mega trends is by classifying them into the free buckets that are shown on this page. The future of computing, production and development of new treatments, and R&D enabling technologies. And while each category is fascinating in and of itself, the connections and the interactions between them is what truly fascinates me. So the connection between chip development and novel therapeutics offers ways to use the power of semiconductors to revolutionize biological treatment options going forward, and for the future of computing and storage to learn from biology with step function improvements and power efficiency and density.
The convergence of production and development of new treatments is being advanced by building block R&D enabling technologies that are shown at the bottom of the page that provide researchers the tools that they didn't have even at the turn of the century. And to truly deploy AI into new application areas like drug discovery and development, there's been a close coupling of the application area with hardware deployment and insights into semi-hardware today, as well as emerging compute technologies. And while individual companies have a lot of resources and incredibly bright scientists to drive this forward, we are also looking at a situation, in my opinion, where no company is or can be an island. The complexity is simply too great and the idea is too vast for a hundred percent internal innovation approach. So here at Merck, and many other companies as well, we leverage all of the ecosystem capabilities that are shown on the right-hand side of this page to create and advanced concepts.
On the left side of the page, engaging with academia, consortia, startups, venture capital funding, national labs and research institutes. And this is an example, I believe that it truly does take a village to deliver these cutting edge scientific breakthroughs. So we've already seen the initial promise of what bioconvergence can mean for mankind. Think of rapid genomic sequencing to deliver the COVID-19 vaccine from what would've been many years to just a few months to save countless lives. And we're just starting to see a few examples of drugs targeted to a person's specific genomic makeup. These are remarkable advancements in science, but they're also just very much the tip of the iceberg of what is possible.
So through bioconvergence, we can imagine a future world where many millions of cells can be identified, classified, and sorted in under a minute, only using a square centimeter of silicon. Implanted systems that are scalable, ultra low power, capable of closed loop stimulation down to the single axon level exist for precise nerve stimulation to treat diseases. And I'll give an example of this in just a second. A hundred thousand or more biomarkers can be detected in minutes. Seamless brain to machine interfaces can be widely adopted. DNA-based archival data storage can be realized to retain 200 petabytes in one gram of DNA, you know, to deal with the ever increasing tsunami of data that man and machine are generating.
So I picked four examples today to cover in the area of bioconvergence, you can see them on the bottom left of this page. So we'll start with bioelectronic medical devices moved to AI-enabled health, translational medicine, and then bio-inspired computing. Within Merck KGaA, we have programs in all four areas as well as several others as we look to bring bioconvergence from the theory or the vision and make it a reality that touches all of our lives today and more so going forward.
So to make this vision happen, we really need to look at these opportunities not in the traditional singular lens of something being a medical problem or something being a physics problem or something being a chemical, or electrical engineering problem, but rather a multidisciplinary approach and multidisciplinary problem to be solved that's waiting in front of us. And this will require a completely new set of collaborations, a completely new set of skills for folks in the industries that typically haven't occurred between science disciplines. So essentially, while we're definitely still need deep technical experts in given scientific domains, we need both these technical experts to be broader than what historically was needed, as well as a set of what I call integrators to bring these domain experts together and work across towards a common vision of the future, and this multidisciplinary approach that it's really beyond what any one individual can handle. So let's start with one example here of bioconvergence, that being bioelectronics. And the opportunity that bioelectronic medical devices hold in becoming a paradigm shift for millions of patients. So while pharmaceuticals offer relief to many, there are those patients whose quality of life is severely impacted by diseases for which pharmaceuticals either have heavy side effects or do not achieve satisfying therapeutic outcomes.
So the use of a selective neurostimulation device, you know, for distinct nerves that trigger the body's biological disease fighting responses can be a game changer for these patients. So Merck KGaA is collaborating with a company called Neuroloop, a German-based startup on the development of a highly selective vagus nerve recording and stimulation bioelectronic medical device. This concept is shown in the picture on the left-hand side where the vagus nerve highlighted in yellow is surrounded by a multi-channel electrode that will allow individualized recordings of the signals traveling through the central nerve system of the body. And through this individualized signal recording, the device can then deliver personalized stimulation of that vagus nerve to affect various parts of the body. And so while still early in development, this is an exciting example of how bioelectronics may improve patient's quality of life for very hard to treat diseases and patients that may have struggled with pharmaceutical-based approaches in the past. Another example of this approach, an extension of this approach is shown on, is illustrated with another collaboration that we have ongoing with a company called Innervia.
We've all seen where devices have become more complex over time and thus compute demands increase. So now think of your cell phone from 10 years ago versus today, the capability, the complexity is night and day different. But opposing this thread of increased compute capability and performance is, the miniaturization over time of devices.
And you combine these two trends together and it necessitates a more efficient power utilization for the given device function. So to address this, Merck KGaA is bringing electronics innovation to biology through a collaboration with Innervia. This collaboration is investigating the use of graphene oxide electrodes to lower the electrical resistance and thus decrease the power consumption while maintaining a constant stimulation efficacy. These two collaborations together should leverage each other and scale so that we can deliver even more capability to patients using bioelectronics.
So we spoke before as well about AI-enabled health and AI is seemingly ubiquitous in all of our lives today. This is certainly true in the health space as well. So as we look about massive amounts of data and each person being different, as we spoke about before, you know, drug discovery typically will take 10 to 12 years with a roughly speaking 10% probability of success of a drug making it through the process lifecycle in reaching patients. Roughly $2 billion plus to bring a new drug to market as well.
So the question is how can we increase the probability of success of a drug making it to patients, having that in-patient result that we're all seeking, and also open up the opening intake of the funnel so that we have more drug possibilities to be able to test and test efficiently. How can we personalize these results more for you and for me so that they do react to our individual body makeups, increasing the probability of success, as I mentioned before, and shortening the drug development time. AI, we believe is a key answer in making all this become a reality.
So when I first started in the industry, we were using tools like Jump to look for data correlations and we are doing this ourselves. And frankly, you know, there there's only so much that one human brain can process itself, but how do we take advantage of these magnificent compute tools that we've built and generated ourselves and use that with these vast datasets that were relatively minuscule compared to today's connected factories and connected datasets. So as we look at drug discovery going forward, or even more broadly, materials discovery going forward, drugs being one example of that material discovery. AI is just emerging in its use case to impact various industries. And we already have real-life examples of how it's impacting drug discovery, how it's helping to fill in missing data holes, how it's helping to design clinical trials in clinical trial frameworks in a more efficient and insightful manner. And how do we increase the probability of drug candidate's success.
So one approach is shown on this page, the model-informed drug discovery and development, MID3 process. So a fit for purpose structural and statistical model is the first major requirement in population pharmacometric model development. This is a very complex and a very computationally intensive task that could benefit greatly from supervised machine learning algorithms. An example of of what Merck KGaA has done is shown on the right-hand side of this page where we compare the classical approach with two machine learning methods, you know, genetic algorithms and neural networks in different scenarios based upon simulated pharmacokinetic data. So the neural network classification task achieved were the most accurate results.
These neural network regression tasks were less precise than neural network classification and genetic algorithm methods, but they enabled a computational gain to be attained by using machine learning that was substantial, especially in the case of the neural networks. This is a great example of deploying some of the massive compute capabilities that exist for all of us and applying it to model selection in the pharma space. So we demonstrated that machine learning methods can greatly increase the efficiency of pharmacokinetic population model selections. In the case of large data sets, complex models requiring long run times, and these results really suggest that machine learning approaches can achieve a first fast selection of models that can then be followed by more conventional approaches after that.
Another example would be, you know, the randomized clinical trials that are the core data sources for meta-analysis and relative efficacy analysis. So as of today, unfortunately, there are missing data between large portions of trials registered in clinical trial registries, and there are reported outcomes published in various scientific journals. I'm sure that many people on the call here have experienced something similar where missing data makes combining data stream or even replicating previous experiments difficult, or even impossible. So this missing citation information makes it difficult to identify all relevant publications for evidence, synthesis and decision-making. And there's four, we've used a novel natural language processing based system to establish links between clinical trial data and their published outcomes in the literature. You know, different approaches, leveraging information, retrieval, machine learning with embedding features that were developed systematically and then evaluated.
And the results really show that, you know, shallow machine learning approaches with embeddings provide promising results indicating the value that it can add to overcome the limitations of manual search and analysis, and missing data points that may be present in publications. Another really exciting opportunity for AI-enabled health is the tremendous opportunity that AI offers for the discovery of new drugs and the probability increase for new drug targets to have the desired therapeutic impact to patients. So more so, on the basic approach can be covered not just drugs, but also novel material synthesis and testing in general. And I think these, we use the same common building blocks going forward.
And so as we look at AI-enabled materials discovery with drug discovery and being part of that, but you could think of other applications in the semiconductor and display areas, aerospace, additive manufacturing, and many others. So one example for drug discovery is shown on this page where in an example of the deployment of AI for molecule designs is an Oxford-UK company called Exscientia. And they claim to have the first two small molecules designed with the help of AI to enter clinical trials. Why is this a big deal? Well, I mentioned before, the traditional drug discovery process takes a long time, four, five years plus.
Four to five years probably at a minimum to come up with a drug candidate. And then the clinical trials testing and, you know, comparisons for that. By comparison, Exscientia's AI design model has been able, the design platform has been able to take this drug candidate and in eight months come up with a viable candidate by computationally sorting through, comparing various properties of millions of potential small molecules looking for the 10 to 20 of those possible small molecules out of the millions to actually synthesize and test and then optimize in the lab. So we can cover a vast amount of space upfront in terms of the possibilities down select those to the most promising 10 or 20, and then really focus the resourcing, the lab infrastructure, the people's time, patient's time on testing those ones that have the highest probability of success in the clinical setting. Another example is shown at the bottom in terms of a collaboration with Merck KGaA and Iktos. So often pharmaceutical companies have identified potentially useful compounds that they can then collaborate with companies like Iktos to learn the parameters of those compounds and find potentially more candidates and more application areas for that.
And it doesn't stop just with companies by any means. Merck KGaA is a founding member of two AI enablement consortium, one based in Toronto and one based in Israel. So on the left-hand side, the University of Toronto is focused on accelerating the discovery of materials and molecules needed for a sustainable future. We were, like I mentioned, a family member of this consortium that was kicked off last year and focused on AI for drug discovery.
This consortium is bringing together multiple disciplines, multiple scientific disciplines, science engineering, data analytics is a big piece as you can imagine. And the combination of these capabilities, both from a what companies need for drug discovery as well as training of students for next generation skillsets is proving to be very valuable. And the right-hand side is a second example of a consortium approach called AION Labs.
This was founded in Israel over in 2021. And with startup call for proposals that were launched over the course of 2022. And today, there are three different focus areas for the consortium. Antibody discovery, antibody optimization and clinical trial readiness. And you can see some of the logos that are on the page, big pharma getting together, collaborate on these building block technologies supported by the Israeli government from a funding perspective as well as venture capital funding as well.
So this is an example of collaborating in a pre-competitive space, forming these startups underneath this consortium entity and then being able to take advantage to be able to internalize these capabilities if and when the technology develops to a necessary point. So one final piece of AI-enabled help that I think is critical to talk about would be, digital medicine and AI in general, obviously, new fields and we've all seen in the news questions about AI ethics and how do we deploy new technologies like this in a transparent and an ethical way going forward. So, you know, while ethical consideration of areas like, you know, transparency, autonomy, justice and so forth, it's not isolated to digital approaches to patient care. The digital AI aspects that I was describing before really puts a sharper or a brighter spotlight to these areas in a digitalized world.
And with some of these unique digital-based considerations, is arising as well. So at Merck KGaA, we were a pioneer in this field with the creation of a Digital Ethics Advisor Panel and a Code of Digital Ethics nearly 10 years ago that really guides all that we do in this area. So this structure provides both internal experts along with external experts, the opportunity to guide program framing in the early stages and a consistent oversight of what may be, you know, previously unseen questions as we deploy AI-enabled health solutions in a much broader manner going forward.
So we'll pivot and move to the third area, translational medicine for the talk here. And this is another example of bioconvergence, but in a different vein, a different way of thinking about it. Translational medicine is and will play an increasing role in the personalization of medicine tailored to each of us, as I was describing before, based upon our unique genetic makeups and environments that we live in. So this approach uses data previously collected from clinical practices to inform and to strengthen novel preclinical scientific hypotheses.
So in essence, you can think about this as a forward and reverse insights feedback loop from bench to bedside and then back to the bench. And the ability to harness these multi-dimensional biologic as well as therapeutic data spanning from experimental to real world settings is increasing exponentially. And so the opportunity, the transformative nature that this provides pharma R&D now and going forward is really quite remarkable. And I'll give you a couple of examples of what I mean by this. So when we think about transforming data to knowledge, we need to consider advanced analytics as a strategic enabler, you know, of translational medicine.
As we're able to collect ever increasing amounts of data from individual patients and groups of patients, the ability to harness this multi-dimensional biological and clinical data from experimental to real world settings is increasingly important, as I mentioned. And all of this has to be transformed, you know, it's transformed pharmaceutical research and development in recent years with the use of these tools that we were describing at AI and machine learning going forward. So how do we, you know, build this analytical capability in a robust and seamless manner is really one of the key questions. And so one of the options for this is this harnessing this multidimensional data both cross-sectionally and longitudinally, our appreciation of the diversity that's intrinsic and extrinsic factors has significantly increased. And you can see a few examples here, you know, clinically, clinical development, you know, that's more broadly inclusive. Responsible nature for ensuring the safety of our drugs.
An ability to deeply characterize and understand signature of patients on a molecular level. And a few examples of this would be on the box on the left-hand side, the molecular signature of human diversity. So advances in biomarker technologies and data science has increased our ability to characterize human diversity at multiple levels of, you know, like I mentioned before, the intrinsic and external factors ranging from the genome to the exposome. The middle box of the quantification, integration, emulation using emerging translational tools, this data provides such a rich substrate for qualitative integration and emulation using these emerging quantification and transitional tools. And then the bottom, the personalized therapeutics, this whole theme of personalization of medicine, personalization of outcomes is a very significant opportunity in the pharma space as we look at trying to understand the impacts of drugs on individuals and a diverse set of individuals then going forward to decrease the access lag to different drug formulations, having the right dose, the right drug for the right patient at the right time.
An example of using this is, is a machine learning approach with a drug called Mavenclad. It's a drug to treat multiple sclerosis and using a machine learning approach in the Mavenclad trials, we were able to identify early predictors of multiple sclerosis disease activity, six months in advance of physical occurrence of physical symptoms being present. So what did these six months of earlier insights enable the doctor and the patient to do? So not only did the outcome itself improve, our understanding of the mechanism of the onset of the disease, or disease activity in MS patients by allowing their the early identification in a clinical setting.
It also allowed for six more months of preventive measures, six more months of therapeutic interventions, six more months of frequent patient monitoring to occur to improve the patient's quality of life, both before the onset of disease activity, as well as the six months for a better life for the patient, a better conditions for the patient over those course of those six months. So this is a, I think a great example of a piece of research that has real-life, high impact importance and a great example of what bioconvergence is all about, which is ultimately to me making the patient's lives better through this multidisciplinary approach and the personalization of the medicine to the individual. I'll spend the last example area for bioconvergence that I'll discuss here today would be bio-inspired computing. So maybe it's the opposite way of thinking about this, most of these other three where how can electronics or how can compute help to enable better clinical trials, better drug formulations, better patient outcomes? The bio-inspired compute is really the opposite. How can compute learn from and be inspired by bio-biology? So if we look at the comparing the human brain to a cutting edge supercomputer, the performance per Watt capabilities is just unbelievably different.
So the human brain uses, roughly 20 Watts to compute to perform a given compute. A supercomputer today would be roughly 65 Megawatts to do a similar job. That's more than 3 million times more than our brain in terms of energy consumption. And it's enough to supply, quite frankly, a small town of probably about 30,000 people with electricity.
So the obvious question is, what can we learn from the human brain? How has the human brain evolved over the millions and millions of years to enable this sort of power efficient compute? And at the time that we have remaining, I'll talk about some of the growing needs for this power efficient compute going forward, and particularly compute at the edge, and some of the work that Merck KGaA is doing in this space. So if we look at power consumption and sustainability, these are some of the grand challenges in front of us. So one example would be, you know, training a single AI model, I've been told, emits about 300 times the amount of CO2 as a roundtrip plane trip between San Francisco and New York, training a single AI model. So we need orders of magnitude improvement in the compute performance per Watt going forward.
And using the brain as our inspiration, we're looking to mimic the architecture of the brain, neuromorphic computing and bring that to AI to consume less power, fault tolerance and robustness, and scalability. So looking forward, it's been reported, by about 2030 information and communication technologies will consume more than 20% of the world's electricity, electrical consumption there, roughly doubling where we're at today. So in other words, development of new materials, development of these technologies to boost computing performance that drives energy efficiency is absolutely critical for our planet going forward. Another example or another information on why this is so critical is we're seeing information move from the cloud to being processed at the edge. So why do we need a edge AI? Well, more and more data is being collected using IoT devices, you know, the need to analyze the data to make decisions about the data locally at the edge as opposed to shifting it to the cloud for that sort of analysis and decision-making. It's projected by 2050, there'll be more than a billion IoT devices.
Quite honestly, I was at a conference a couple of weeks ago, they were projecting greater than 200 billion by 2050. Regardless of what the number ultimately ends up being, it's a big number. And so it simply is not gonna be feasible to move all of that data that's generated by these devices to a central cloud, processing it there, and then send the information back to the edge to act upon it.
So a decentralized data processing capability at the boundary of networks at the edge is really driving a lot of the future going forward. And on the right-hand side, you'll see a few examples of why this is so important. So an Airbus A-350 airplane is in a glorified sensor, the word, a massive set of sensors in the sky, generating about two and a half terabytes of data each day.
Global security, security cameras generate about 2,500 petabytes of data each day. And the growing use of voice assistance, even for very small amounts of time would necessitated a doubling of the data center footprint for companies like Google, if everyone's just using their Android phones for measly three minutes per day. So clearly, the processing of data at the edge and doing so in a very efficient manner is critical going forward for growing of the industry as well as sustainability. And there are some fundamental differences in the operation, the organization, the programming, communication, timing between Von Neumann architectures, which on the left-hand side, which are more of the classical CMOS that we all know from our computers and phones, and everything today versus a Neuromorphic architecture that's shown on the right-hand side.
These differences are substantial and probably would be a discussion in and of itself. So I definitely don't have time to dive into all the details here today, but for the sake of time today, let me just say that, the neuromorphic architecture is on the right-hand side inspired by the brain in its collection of neurons and synopsis that will enable both processing of data and the storage of data in a very different and a very highly energy efficient manner going forward. So going back to the slide that I was showing before about the brain versus supercomputer power efficiency, we have the opportunity to learn from the brain, the millions of years of evolution that the brain has undergone and truly use that as a template for what future computing may look like going forward.
So, artificial neuromorphic systems are really an indispensable in understanding the human brain and in constructing the computational systems from that. So how do we mimic and silicon the brain's neuron function? You know, using materials like phase-change memory, PCM materials that has a unique set of material properties that researchers are exploring today for this. And as the name describes, you know, phase-change memory conducting changes as a function of the number of crystalline pulses. And you can start to see that on the top graphic that's on this page. So after approximately, you know, six pulses, the measured conductance rises sharply and causing essentially, the quasi-neuron to fire in this case. So we are able to use these new material systems in a neuromorphic architecture to mimic the brain on silicon.
It's been demonstrated that the combination of PCM materials with ovonic threshold switches, OTS switches can enable this functionality. An example is shown here with the ALD depositive, GST build, Germanium, Antimony to Tellurium that's shown in the cross-section image here. So GST is a interesting material system where the material changes its phase at roughly 150 Celsius when it switches from amorphous state to a cubic state. And then around 200 Celsius switches to a hexagonal crystal state as well.
And then ultimately this can be reversed by raising it above the melt temperature and then very quickly quenching, and you can see the cycle that's on the right-hand side with the crystallization states changing, and then the amorphous. This is an opportunity to again, mimic the functionality of a neuron and take advantage of the brains compute learnings that we do have. So to jumpstart this, about a year ago, Merck KGaA electronics business sector kicked off a neuromorphic computing incubator based out of our Silicon Valley hub to productize neuromorphic synaptic memory designs using novel and proprietary in-house materials.
So our Silicon Valley site has an advanced 300 millimeter equipment capability, equipment line that's both used for testing of new material systems, circuit designs, as well as full integrated silicon processing capabilities. And we're using this capability, we're leveraging this capability to advance neuromorphic computing and look not just at the material properties and performance themselves, but also more at a system level of how do these material properties, how do these new material stroke geometries ultimately manifest themselves in a performance of an integrated piece of silicon. So while still early, the technology is advancing rapidly and holds a lot of opportunity to bring neuromorphic computing and the power efficiency that we can provide to tangible products in the coming years. And I mentioned in one of my first pages about engaging the ecosystem that a lot of these problem statements are bigger than any one company. And this is an example of how Merck KGaA is using its venture capital group, Merck Ventures, to invest in a couple of companies that are shown here, MemryX and SynSense for the early stage evaluation of combining compute and storing in the same location that MemryX is focusing on, this uses a crossbar array technology and represents, you know, one synapse at each of the crosspoints there with various synaptic weights being applied. The SynSense shown at the bottom is a company that we've invested in the focus on the development and dedicated event-driven neuromorphic processing for real-time vision processing.
And so these processors, ultra low power consumption, ultra low latency can really pave the way for IoT devices now and going into the future for gesture recognition, face or object detection, location and tracking, surveillance and so forth. And one final piece, just 'cause it's a technology that absolutely fascinates me is DNA-based storage. And to me this could be the ultimate example of what bioconvergence can look like. So can we move from silicon space storage, DRAM, NAND, sorts of memory storage capabilities today and over time shift this to DNA. Why is this interesting? Well, simply because the storage capacity, the storage density is enormous.
So, you know, if you look at the density of a single gram of of DNA can store at least 200 petabytes of information, which is almost equivalent to a million external hard drives of one terabyte capacity each. So just an amazing amount of storage capacity. Another way to look at this would be, if you take all the information as all the data that exists in the world today and we're combining it at a one storage location, you could theoretically store this in about a kilogram of DNA. So the opportunities that this presents are enormous. Now, at the same time, the technology challenges are also still enormous. This, you know, it's, it's not ready for productization by any means today, but I would say it's making good progress as researchers devote more and more attention to this going forward.
So I'll leave you with four key takeaways today. Bioconvergence is rapidly disrupting numerous fields and will continue to do so. We're just at the beginning of this inflection point.
Future technology complexity requires a multidisciplinary approach. Digital and data is gonna be the backbone of this going forward and the challenges are numerous. The opportunities are immense and the solutions are gonna require a much deeper understanding and deeper broader collaboration of what we've had today.
So to realize this bioconvergence vision, this personalized healthcare for individual patients, allowing us to gain insights from group data that was previously hidden, it's gonna require much deeper blending of these insights from digital and data, from material science, from engineering, from healthcare. But I firmly believe that Merck KGaA with its industry leading positions in life science, healthcare, and electronics is uniquely positioned to drive this forward. I'd welcome any follow-ups that folks may have, feel free to please reach out to me, my email's at the bottom of this page here. And I look forward to collaborating here going forward more in the future. Thank you so much for your time today and I wish everyone a great 2023.
2023-02-16 13:15