Trendyol Data Science Meetup
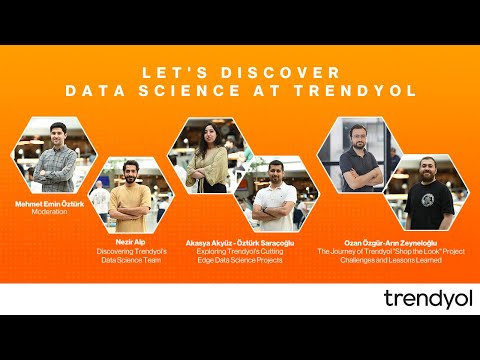
We extend a warm invitation to all of you to join us in our upcoming events. You can stay informed by following the Trendyol group's link page. Let me take a moment to introduce myself.
I am one half of the Emin Trendyol road crew, and I've been a part of this journey for several years now, beginning in November. Let's swiftly outline today's agenda and kickstart our event. Firstly, we will delve into the structure of our team and shed light on how we operate as a unit. Moving forward, we will provide an overview of our general work approach.
Subsequently, we'll delve into our various projects, aiming to offer insights into the intricacies of each endeavor. Furthermore, we'll explore the technical aspects of our scale project, a particularly noteworthy initiative. To cap off the event, we encourage you to participate actively by posing your questions. We will allocate time to address these questions during and after the broadcast.
Our entire session is anticipated to last about one hour, and we greatly appreciate your active engagement in advance. Thank you once again for your presence and enthusiasm. Without further ado, I'd like to extend a warm welcome to our first speaker. Let's get started! Hello everyone, Let's dive into today's agenda. Greetings, my colleagues. A warm welcome to all of you.
Allow me to introduce myself first. I'm Ben, responsible for leading our data team. Today, I'll be guiding you through our team's structure, organization, and business approach. To start, I'd like to shed light on our team's purpose and the methods we employ to achieve it. The primary objective of our team can be broken down into two key components. Firstly, we aim to optimize all aspects of our application, ensuring that algorithms are thoroughly examined and critiqued by the algorithm's owners and then analyzed for customer feedback at the highest level.
For instance, consider a user browsing through our application's home page – when I conduct a search, the algorithms highlight relevant products, thus demonstrating optimization. Our challenge is to replicate such enhancements across various touchpoints. Secondly, our goal is to enhance efficiency through optimization, subsequently bolstering operational quality and delivering elevated customer service. This isn't the sole responsibility of the Data team; rather, our friends from Msi Am, who excel in data analysis, are also integral to this process. Collaboratively, we work to craft applications that offer our customers an exceptional experience. As data scientists, our core task revolves around understanding business or product requirements and finding solutions.
This demands a deep comprehension of how to translate business problems into mathematical challenges. Identifying these challenges, formulating solutions, and implementing them technically are essential aspects of our work. To ensure successful data synthesis, we emphasize thorough problem understanding, solution design, and application of effective methodologies. This holistic approach maximizes the efficacy of our team. Turning to our team structure, we've designed it with product development at its core.
Our application's products and processes interlink, necessitating low interdependencies for seamless product evolution. We've organized our team into eight distinct units, aligned with current trends. Each unit functions independently, yet collaboratively, to maintain a cohesive product development cycle. Creating this organization required relentless effort, learning from errors, and discovering innovative approaches. Now, let me share some insights from this journey and delve into the more enjoyable aspects of it.
In the realm of Data Science, the foremost factor is human resources. The most significant contributor is the human intellect, as it's not possible to rely solely on algorithms or AI for solutions. Customization and adaptability are critical, and we require a skilled and steadfast team to harness these capabilities effectively. Moreover, our team must possess diverse strengths to tackle various challenges adeptly.
Collaboration and sharing innovative perspectives are fundamental to our success. This is why our team consists of individuals with distinct skills and mindsets. We also empower our team members to drive innovation and provide technical support, fostering a dynamic environment. Regular interaction and learning are essential in a rapidly evolving field. Collaboration with the market academy and cross-team engagements are pivotal.
These alliances facilitate shared learning and mutual assistance, which is instrumental in navigating the dynamic landscape. To streamline our processes, we hold focused meetings and discussions. These sessions tackle shared problems, and we brainstorm alternative solutions collaboratively. These sessions also extend to larger organizational meetings where we share knowledge gained or skills honed during the week.
In conclusion, our journey is guided by the pursuit of optimization and innovation. Our team structure reflects our dedication to holistic product development and problem-solving. By fostering diverse expertise and collaboration, we've built a foundation that can readily adapt and innovate in our ever-changing field. Thank you for your attention, and I'm eager to engage in discussions and answer your questions. Let's continue this enriching journey together. OOf course, these are some of the activities we undertake within our project-specific structure, but continuous improvement of our processes is also a priority.
We need to establish a solid infrastructure for this purpose. For instance, dealing with issues such as merging and reception problems in various parts of our application requires effective solutions. We engage in discussions about the types of models to employ and the best approach to take. Our focus extends to other processes as well. For instance, we strategize on how to enhance the user experience. We plan out the flow of our processes, determine the right kind of collaborators, and establish evaluation criteria for interviews.
We iterate and refine our processes, ensuring they align with team needs and objectives. Our aim is to optimize these processes and bring them to their highest potential. Yet, these efforts alone are not sufficient. Continuous self-improvement is essential. For this, we offer training programs that I, as part of the team, participate in. Additionally, we allocate an education budget so each team member can receive education tailored to their needs and thus broaden their skills in various domains.
However, one of our most pivotal aspects is knowledge transfer. This is crucial for efficient functioning. We've developed efficient knowledge-sharing procedures, one of which is peer rotation. Team members who specialize in different areas share their expertise, enhancing our collective capabilities. This approach accelerates the learning curve, enabling our team to excel in various domains and provide different perspectives.
Of course, these are just some foundational insights we've acquired throughout this process. Knowledge transfer is at the core of our team's growth strategy. With that, I conclude my part. Now, my colleague will dive into more enjoyable aspects and provide concrete examples of our projects.
Thank you. Öztürk, over to you. Hello, everyone.
How's everyone doing? Thank you for being here. I'm Öztürk, and I work in the Complex team. As the Data Science team, we are responsible for several unique areas. Let me walk you through some of them. Firstly, our primary focus is the front-end, where our customers interact with our platform.
Think of it as the first point of contact, where user engagement unfolds. It includes personalized product suggestions, campaign recommendations, and curated collections. We aim to deliver tailor-made experiences, enriching users' journeys on Trendyol. Our home page is analogous to a blank canvas that we endeavor to fill with relevant content.
This process involves automatic and manual curation, ensuring that products are strategically positioned and highlighted to captivate users. For instance, when users view a product or conduct a search, we carefully present items that align with their preferences. Our reach extends beyond the front-end. We also have a "Closet" feature, where customers can create curated collections of their favorite products.
This also influences our algorithms, as we factor in users' preferences to generate personalized product recommendations. Additionally, we work extensively on personalizing content and product suggestions. These recommendations cover various aspects, from personal style to product preferences. This dynamic approach aims to enhance customer satisfaction and engagement.
Speaking of content, user-generated content, such as reviews, plays a crucial role. We analyze and categorize reviews to guide customers effectively, ultimately assisting them in making informed purchasing decisions. We strive to streamline the review experience, leveraging the valuable feedback provided by users. Moreover, we focus on optimizing the customer journey in a holistic sense.
For instance, in our Food and Services section, we present hourly meal suggestions to users. Our algorithms prioritize freshness and variety, allowing users to explore diverse cuisines and flavors. Behind the scenes, there's an intricate process involving our Operations team. They determine order sequences and logistics, ensuring prompt delivery and an optimized user experience. These examples illustrate a few of our numerous projects, each contributing to the overall customer journey and satisfaction. Our team's efforts are dedicated to creating seamless and enjoyable experiences across our platform.
Thank you for your attention. Next, let's continue with Ak, who will delve into other exciting aspects of our projects. Ak, the stage is yours.
Thank you, Öztürk. Hello, everyone. I'm Ak, part of the Search and Content teams. Our tasks encompass diverse functions, from search algorithms to content processing. Let me provide you with some insights. Search is a pivotal aspect of our platform.
It starts the moment users begin typing their queries. We focus on enhancing the search experience, ensuring accurate and relevant results. This involves personalizing search outcomes, fine-tuning results, and optimizing the search process for user satisfaction. Our platform also features sponsored products, where we strategically position items to cater to user needs and vendor interests. This process requires careful optimization to maximize the benefit for both parties.
Content plays a significant role in our platform's success. User-generated content, particularly reviews, influences purchasing decisions. We analyze and categorize reviews, extracting insights to guide users effectively. Moreover, we enhance user engagement by placing relevant tags on products, helping users identify specific attributes.
Our team also works on advanced visual recognition. We process images to identify objects, scenes, and attributes, which contributes to enhancing the user experience. Furthermore, we've embraced machine learning and AI, with models tailored for our unique platform. We've developed models to handle harmful content detection, ensuring a safe and enjoyable experience for our users. Our projects are diverse, ranging from improving search algorithms to enhancing user-generated content utilization.
This multifaceted approach supports our platform's growth and user engagement. Thank you for your time. Now, I'd like to introduce Ozan, who will share insights about our exciting journey in the Alum Project. Ozan, take it away.
Hello, everyone. I'm Ozan, a member of the Alum team at Saat.com. I'm thrilled to share the journey of our project with you. Our journey begins by identifying products in images across various categories and incorporating them into our platform. Imagine a wedding setup with different products, such as wedding dresses, accessories, and decorations.
Our goal is to locate these items and present them to users in a relevant manner. Our project kickstarted in March 2022 and evolved into multiple phases, with notable achievements along the way. We collaborated extensively across teams to ensure a seamless process. Digital services, technical infrastructure, and filtering tasks were all interconnected, underlining the complexity of our project.
The project's scope expanded as we sought to differentiate similar products in images. This involved separating images into distinct sections, necessitating the application of models tailored for these sections. Furthermore, we delved into fashion segmentation, an essential component to provide users with the best experience.
The challenges spurred us to develop advanced models, enabling us to accurately categorize and present products. We also realized the importance of efficiently managing product information. This included handling product entries, monitoring outgoing models, and managing constant services. These aspects combined to ensure a smooth flow of information and products through our platform.
In essence, our project revolves around finding main products within images, extracting relevant information, and enhancing the user experience. While complex, these steps are integral to ensuring our platform remains reliable and user-centric. Thank you for your attention. I hope you enjoyed learning about our journey. I'll now pass the torch to our next speaker for further insights. Thank you.
Is the product the main concern? One more reason is that when customers view a project, the primary product is not currently displayed. As I mentioned, this is addressed in a distinct context. We only aim to showcase other products. This brings us to one of the key principles our specialists follow – an algorithmic approach that requires two key pieces of information.
Firstly, we have the smart product category. For example, in this instance, sweatpants fall under a product category. Secondly, we have the lens model. Take the example of tracksuit bottoms. This model categorizes them accordingly.
Let's proceed. I'm here to pass the floor to my team mate. Hello everyone, I'll be providing further insights after the previous speaker. I work alongside Ozan.
Let's continue discussing velocity. Our first focus is on data and machine learning. During the data creation phase, we encounter critical aspects. Trendyol boasts an extensive range of products, particularly in the fashion domain.
With a whopping 279 different clothing categories, we aimed to leverage this vast data spectrum for our purposes. Gathering data from 300,000 unique products across 279 categories, each featuring around six images, we amassed a total of 1.77 million images for our training dataset alone. However, with such voluminous data collection, challenges inevitably arise. Pay heed to some of these issues listed below.
Among the challenges, the most significant one pertains to data inconsistency, as seen in the issue of different IDs being associated with identical product images. This stems from the fact that different sellers employ distinct images for the same product, often without being aware of each other's actions. Consequently, our model interprets them as separate products, which affects its performance significantly. This calls for careful attention when structuring the data. Another challenge we face involves team products, which present a lens-specific issue.
As evident in the middle examples, these are essentially the same products, yet our model needs to identify the subtle differences and similarities between them. Furthermore, we grapple with Photoshop products – those with identical images except for slight variations, such as logos. This poses a challenge for our model, impacting its accuracy. Moving on from these challenges, let's delve into an intriguing issue – adversarial machine learning. Before its rise in popularity, adversarial learning faced extensive scrutiny. This issue spans a variety of scenarios, including human identification.
For instance, one might want to determine whether a person in an image on the left matches the person in an image on the right. This could be useful for multiple purposes – establishing the person's presence across cameras or gauging familiarity through social media. This demonstrates the power of adversarial learning in real-world applications.
Furthermore, this technology proves valuable for visual similarity. If products are visually similar, adversarial learning can distinguish subtle differences, significantly enhancing our platform's efficiency. While I won't delve into overly technical details here, our project incorporates three primary methodologies for individual similarity. If you're interested in the nitty-gritty, we'd be delighted to explore these further and tailor the discussion to your preferences.
Allow me to provide a brief overview. The most fundamental approach is called "Softmax," likely a familiar term if you've encountered machine learning before. Softmax essentially calculates the likelihood of an image belonging to a particular category within the dataset.
This process involves comparing the image against every item in the dataset and calculating its affinity for each category. However, this method faces an issue when dealing with unseen examples, as the results tend to be unreliable. Another prominent method is "Siamese networks," popularly used in Turkish and English variations. This method involves using a reference sample – an image with the same ID – to determine similarity. Positive pairs are images of the same person, while negative pairs feature distinct individuals.
The Siamese network then aims to minimize the distance between positive pairs and maximize it between negative pairs, thus optimizing similarity determination. The third method, "Triplet Loss," involves using anchor, positive, and negative images to train the model. By decreasing the distance between the anchor and the positive while increasing it with the negative, we fine-tune the model's similarity recognition capabilities. In practice, we predominantly utilize the Softmax method, though we've explored variations depending on specific project needs and data characteristics. Let's transition to "trade-off" and "speed." There exists a disparity between theoretical models and practical systems.
In our specific problem domain... They also want to mention it as a challenge. Sure, here's the proofread version of your text: **Trade Training Sequence and Algorithmic Approach** In the context of Trade training sequence, our goal is to make the model perceive different photographs taken from various angles in distinct ways. We aim to enable our model to identify similarities even when presented with diverse visual representations. In essence, this implies our desire for versatility – for instance, using a shoe sample to illustrate, if we show the first product image on the left, we are not limited to only that specific image of the shoe. Our lens model encompasses an entire collection of shoe images.
Our algorithm, which is identical in its functionality, determines whether a given image is the primary product. We initially gather the primary images of this collection. However, on Trendyol, virtually no product has just a single image.
Instead, products often feature multiple images – the second, third, fourth, and so on. Therefore, we capture a range of images from the shoe collection. Consequently, we recognize that the first image we found and the subsequent images all correspond to the same product. Hence, during the training phase, we utilize various images of the same product to carry out our training. The situation during deployment, however, has some nuances.
As Ozan has highlighted, our primary objective is not solely to identify analogs of the primary product, but rather analogs of by-products. To illustrate, consider the initial visuals depicting trousers – these images display a pair of trousers offered as different products. While our model does correctly identify them as trousers, the main focus is on the trousers themselves, with less attention given to the accompanying shoes. As a result, when we extract the shoe group, we acquire images that exhibit a wide array of angles.
Consequently, we observe the distinction between what we refer to as "front" and "profile" images. Notably, for the high-quality images of shoes, the primary product, we search for images displaying a markedly clear quality. Effectively, there is alignment between the two – the primary product with its well-defined image and the auxiliary images that offer varying perspectives.
We shall now proceed. Let's delve into some additional challenges we've encountered. I'll conclude after this segment. A topic I mentioned earlier is related to small objects.
In the first image, an earring is visible on the model. Our target model groups such items together, generating a composite image with a notably low resolution. This is due to the minuscule size of the object. Yet, we also possess a high-quality, low-resolution image of the earring from the front, our reference image. It becomes essential to navigate through a range of images to identify a precise match.
Finally, there's a substantial challenge posed by modern product images, where items are cropped for various reasons. For instance, consider a coat as an example. The requirement is to capture the coat itself. However, the image could also include elements like construction sites or other items.
This can introduce ambiguity as the model could potentially confuse such elements as part of the primary product. In addition, I'd like to extend my gratitude for your attentive participation throughout the presentation. As Ozan and I bring our discussion to a close, we would like to express our sincere appreciation. We invite you to our question and answer session, and preceding that, you can take advantage of the QR code on the screen to explore employment opportunities within the Data Science team. Feel free to engage with us with your inquiries, and we will strive to address them comprehensively. To facilitate this, we welcome interested parties to join us on stage.
Thank you for your attention. And now, we transition to the Q&A session. Prior to that, displayed on the screen is the QR code through which you can explore career opportunities within our Data Science team. As we anticipate questions during the live session, we shall endeavor to answer them all. We invite those with inquiries to step forward and join us on stage. Additionally, please continue to follow our upcoming events through the Trendyol group link's page.
And that concludes my presentation. Thank you for your time and attention. Sure, here's the proofread version of the transcript: **Event Q&A Session** I think we're ready to proceed with the next question. When comparing pre-made libraries with your final product demand ratio, which do you find more valuable? Do you mostly utilize readily available libraries or focus on algorithm development? Our approach differs somewhat from directly utilizing specific libraries.
As seen in the case of T Robert, we are predominantly engaged in crafting unique solutions tailored exclusively for Trendyol. While we certainly appreciate and employ ready-made libraries when suitable, our solutions are not solely reliant on them. Our methods for solution development and the deployment of libraries align based on the demands of our projects. This approach might set us apart from some other leading companies in the field. Moving on to the next question: Do you have plans to incorporate Arabic language models in your algorithms? Certainly, there are plans in motion to expand our capabilities to include Arabic. As Trendyol continues to establish a robust presence, adapting to new linguistic nuances and patterns becomes essential for seamless service delivery.
Another question touches on project initiation: How do you decide on the projects you pursue? Are these decisions top-down or based on employee contributions and ideas? Our project selection process is heavily influenced by our product-centric focus, as we outlined earlier. We value the input of all team members, collaborating with product teams to gather a variety of ideas. These ideas serve as the basis for our project generation, fostering a collaborative atmosphere and ensuring a comprehensive approach to project initiation. Lastly, have there been instances where you encountered significant challenges or mistakes in your projects? If so, how do you address and recover from them? Indeed, challenges are an inherent part of any undertaking, and we have encountered our fair share.
In one instance, an automation process yielded an unfortunate display error on the website due to image discrepancies. These moments, however, serve as a testament to our team spirit and collaborative ethos. Our cross-functional teams collectively address and rectify such issues, exemplifying our unity and problem-solving prowess. Thank you for addressing these questions. For further inquiries, let's welcome our next participant.
Hello again, and welcome back. Thank you, hello. Here's a piece of feedback: Your creation of the sample algorithm with the learning curve is impressive.
Could you possibly share some insights into your learning and deep learning endeavors? Thank you for your kind words. Indeed, our plans include sharing more about our journey into learning and deep learning through informative articles and posts. Stay tuned, as we plan to provide valuable insights into our progress and experiences in these fields. Now, regarding the technology stack you mentioned during your presentation: What tools and platforms do you use to run algorithms and manage data? Certainly, our technology stack is diverse and tailored to our needs.
We leverage cloud platforms from AWS and Google Cloud for major workloads. Smaller tasks and one-off jobs can be executed using cloud or local machines. Additionally, for specific projects, tools like Hadoop and Spark come into play for effective data management. We adapt our stack to suit each project's requirements. In terms of self-improvement within Data Science, do you have any advice for those looking to enhance their skills in the field? Absolutely.
Practical experience is key – working on various projects, collaborating with different teams, and continuously expanding your skill set. Engaging in competitions can be immensely beneficial. Furthermore, delve into research and stay updated with the latest developments. It's a journey of continuous learning and hands-on practice that shapes expertise in Data Science.
Lastly, for those who are interested in joining the field, could you provide some general recommendations on how to advance their career in Data Science? Certainly. Gain hands-on experience by working on real-world projects. Continuously challenge yourself to tackle diverse problems. Participate in competitions and study solutions to understand different approaches.
Mastering theoretical concepts is equally crucial – knowing the underlying principles enhances problem-solving abilities. Thank you for addressing these questions. Unfortunately, we won't have time for further questions in this session.
However, the recorded video will be available on YouTube. We appreciate your time and insights. Thank you for having us.
It's been a pleasure sharing our experiences and insights. Thank you for your participation. For future events and to stay connected, please follow the Trendyol Group LinkedIn page. Once again, we appreciate your presence and engagement.
Until next time.
2023-08-22 21:31