Regulation of AI enabled IGT Technologies (Health Canada, US FDA, UK MHRA)
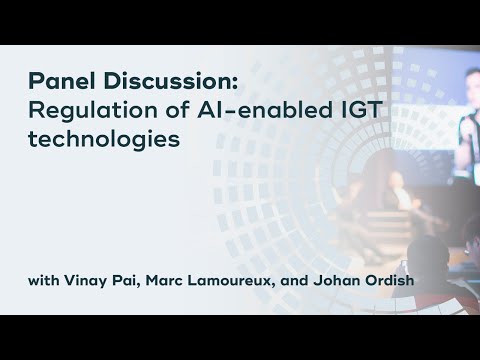
- Alright, good afternoon, everyone. So we're going to get started now. Thank you for being here for this webinar presented by INOVAIT. INOVAIT is a pan-Canadian network led by Sunnybrook Research Institute and supported by the federal Government of Canada with the goal to harness breakthroughs in image-guided therapy and the power of digital systems and artificial intelligence to advance medical imaging technologies.
My name is Ahmed Nasef. I'm the programs manager for training, networking, and outreach at INOVAIT. These webinars are a way to hear firsthand from practitioners, subject matter experts, and key opinion leaders on a wide variety of topics related to the role of big data in the field of image-guided therapy and the associated challenges and fundamentals of integrating AI into health technologies and IGT applications.
We are very delighted today to have leading regulators from Health Canada, the FDA, and the Medicines and Healthcare Products Regulatory Agency in the U.K. who will be discussing approaches and recent efforts in regulating imaging and image-guided therapy technologies that incorporate AI. Our guest speaker and session moderator today is Marc Lamoureux, manager in the Digital Health division, at the Medical Devices Evaluation Bureau at Health Canada. We're also very delighted to have Vinay Pai, digital health specialist at the CDRH at the FDA, and Johan Ordish, the head of software and artificial intelligence in the Innovation Devices division at the Medicines and Healthcare Products Regulatory Agency in the U.K.
Before turning this to Marc to get us started, I'd like to go very quickly over this schedule and some of the ground rules for this webinar. Please know that this session is being recorded. By staying, you are giving us consent to record and archive the video. Later viewing will be available on our website through our YouTube channel, and with French subtitles will be available as well.
We'll start by having brief 10-minute presentations from each speaker, and then we'll have a panel discussion and a Q&A session at the end. If you have any questions, you can post them in the chat or you can use the raise-your-hand feature, if you wish to speak with our guest speakers. I would like to take this opportunity to announce that INOVAIT is now seeking applicants for its Pilot Fund for R&D projects that integrate image-guided therapy with artificial intelligence to advance medical innovation across Canada. I'll be posting the link to this funding competition shortly in the chat. (https://inovait.ca/funding) So without further ado, I'm going to be passing this over to Marc to get us started.
Marc, over to you. - Thank you, Ahmed, and thank you all in attendance for spending some time with us. I'm really happy to be moderating this. And a very special thank you to our guests from the MHRA and the U.S. FDA. So as you heard, I'm accompanied by two colleagues, and I'd like to say sort of two like-minded regulators, so I'm very happy about that.
And I had the pleasure to be speaking with this group about a year ago, but you only got me. But this time, I'm coming with friends. So what we'll do is, to lead us off, I'll pass the mic to Vinay. And as Ahmed had mentioned, we can start this part of the agenda where all three regulators will be providing sort of a brief presentation from their respective jurisdictions. And then, we're gonna come together after the fact, have a little bit of a discussion, and then open the floor to questions from the attendees. So Vinay, over to you.
- Thanks. Can you hear me, Marc? - [Marc] Yes, we can hear you. Thank you. - Thanks so much. I'm gonna share my screen. Can you see the main slide? You should be able to, I think. - [Marc] Yes, we can see your screen.
- Okay, thanks. All right, so I guess there's some pressure to help Marc out here. Perform better than last performance, I guess. But here, I'm gonna present a little bit of an overview of how the FDA looks at AI/ML-enabled medical devices, and it's more of a general overarching view of how things are looked at from our perspective. And first thing I'm gonna provide is a disclaimer that basically this represents an informal communication from me as an individual and doesn't necessarily represent the formal position of the Agency as such.
Setting that aside, I wanted to step back a little bit and mention about the CDRH Digital Health Center of Excellence, or the DHCoE, which we launched in September of 2020, so almost two-plus years back. And the focus of this particular center was to empower us to, when we say us, I mean all the stakeholders in this community of digital health, to first of all, connect with each other, then share knowledge, share technology, share information. And the plan being that, when you do these two steps, you will automatically lead to higher innovation. And so the community has been building up in that space, and as you can see on the right-hand side, some of the accomplishments that this particular center has been striving to achieve and has achieved, and this includes some of the public documents that were released, some authorizations that we have obtained, and also some regulatory frameworks that we've been working on, including the AI/ML Action Plan, as well as what we've done with Health Canada and MHRA on the Good Machine Learning Practices and Principles that Johan and Marc will be going over in the later part of their talks.
One of the greatest benefits AI/ML is that the software has an ability to learn from real-world use and experience, and it's capable to improve its performance based on that learning. And as the AI/ML technologies continue to advance, we see there is a tremendous opportunity to advance healthcare. And the nice thing is data is being more easily available, and that technology that learns from that data is able to help organizations, healthcare professionals, and patients gain better insights into all aspects, like prevention, diagnosis, management of disease, as well as monitoring conditions.
When we look at the type of work that the FDA has been involved in, it's a pretty wide range over the last three-plus years. And at the bottom, you see the Office of Science Technology Policy Blueprint for an AI Bill of Rights. It was released a few months back. And how the activities that our center, the FDA, has been working on alignment with a lot of these Bill of Rights aspects, as we look at safe and effective systems, algorithm discrimination protections, data privacy, and explainability of things, as well as looking at human alternatives, considerations, and fallbacks. Some of the things I would point out are, for example, collaborative community participation related to AI/ML.
And also, we have a list of currently marketed AI/ML devices, which were updated recently, and as well as work we've been doing in the International Medical Device Regulatory Forum for key terms and definitions in machine learning-enabled medical devices. As I mentioned, we have provided a list of AI/ML-enabled medical devices that have been authorized by the FDA. And as of October, it's has been more than 500 devices that have been approved or cleared.
And this list is now available, and you can use the QR code, which is shown on the bottom left, in the middle here, to actually scan and look at this list and find out whether your favorite device is on this list or not. And if it's not, and if it's an AI/ML device, you can always reach out to us and inform us as to why you believe that particular device should be on the list. So this is allowing us to see how explosive the growth has been in this industry and how quickly it's moving forward. And so there's a lot of adventure in this space right now. And so it's very fascinating to see how the devices are evolving. Here are some examples of various devices that have been approved or cleared, and again, the QR codes provide you direct links to the articles that have been provided here.
And basically, what it shows is that AI/ML-enabled devices can support a user, whether by identifying areas of concern in an image or by guiding a nurse's hand during the test, like for example with Caption Guidance on the right-hand side. Another thing these examples have in common is the use of this pathway, which we call the de novo regulatory pathway, which we established in 1997 and streamlined to FDASIA in 2012. It's one of the more modern regulatory pathways we have for medical devices. And the advantage of this pathway is, unlike the substantial equivalence pathway, which is a 510(k) pathway, it moves beyond that alone for providing reasonable assurance of safety and effectiveness for novel devices. It allows us, as an agency, to establish special controls which can help define the performance expectations.
And in the case of this one example that's shown on the right here, have actually enabled us to implement a modification control plan for the device that allows the device to perform updates without FDA review, provided certain performance criteria are met for that particular device. So keep this in mind because the de novo pathway is a pathway that has allowed us to do some of these things. So it might be an approach you might be interested in looking at if you're coming to the FDA for your device. One of the first challenges when looking at new technology and new areas of development is making sure that we're all speaking the same language, we're speaking the same things, and the context are all relevant. And the way this has been done is working through the IMDRF, which is the International Medical Device Regulators Forum. And this is a document that was recently released in May 2022, where we have a definite key terms and definitions for different aspects of machine learning-enabled medical devices are provided here.
And basically, what you can see is that it's the science and engineering of making intelligent machines, especially intelligent computer programs and can be utilizing different techniques, especially based on statistical analysis of data export systems that primarily rely on if-then statements and machine learning. So because I have limited time, I'm gonna go a little bit faster here. So one of the key things in looking at machine learning-enabled medical devices is transparency. And we wanna make sure that when we have changes to the machine learning-enabled medical devices, there are certain aspects related to the change that we should keep in mind, like the cause and effect, the trigger for the particular change, and what kind of domain it's being used and what the effects that come out of this particular change. And that's particularly relevant for the medical device itself. And then, you look at the environment in which it operates and how do you look at the cause and effect and the domain where it's operating in.
So these are some of the things that we need to keep in mind. And being transparent on these fronts helps us make sure that everybody aligns with how this particular medical device is being utilized in the field. And one of the ways that we believe that this will be easy to do is through the approach of something we call the Modifications Controls Plans. As we said in our white paper a couple of years back, which is looking at software pre-specifications as well as the algorithm change protocol.
Basically, the pre-specification tells what you want to do. And the algorithm change protocol is pretty much explaining how you plan to do it. So if you wanna make a change to your, if you're retraining it for performance improvement, for example, then show us how you manage the data, what are the different aspects of training and test data, and how you're collecting that data, and so on so forth, how you plan to retrain the algorithm, what are the performance evaluation that you're planning to perform to make sure that this performance is not deteriorating from the initial pre-submission test data. And then, how do you plan to update your algorithm and the software as it goes forward in the field? So these are some of the aspects.
Obviously, the details, the weeds matter, and it depends on how your particular algorithm change protocol applies to your particular device. So we would always encourage industry to come and talk to us when they're coming in with some device in that space. And the nice thing is, many changes may not necessitate review. This is a diagram that comes out of or the flow chart that comes out of the AI/ML discussion paper that I mentioned before.
And basically, what it's talking about is that, if you have an SPS and an algorithm change protocol already approved, then basically, if your modifications do not require a new 510(k), then you just document the change if you have an approved SPS ACP, and you should be good to go. On the other hand, if there is a modification, then if the modification that you're proposing is within the scope of what you agreed upon initially, then you are fine so long as it doesn't lead to a new intended use. So if it doesn't need a new intended use, then all you have to do is get a focused review of the SPS ACP if it's outside the scope of what you had agreed upon. On the other hand, if you are doing some things where the intended use changes or the modification suggests a new 510(k), then you have to come in as a pre-market review. So this is one of the initial flow charts that we had.
I know Marc said 10 minutes. I'm going to go a little bit faster. And finally, looking at AI/ML medical device opportunities and challenges, as you can see, I mean, we pretty much know that there are a lot of opportunities in this space for how we can have earlier disease detection and get more insights into human physiology and other aspects of it. But along with all these opportunities are a huge number of challenges, and I won't go into this particular part because I know Marc and Johan are gonna talk about it and we'll also have a discussion on this topic. I'm fairly certain about it.
So in order to save time for discussion, I'm gonna skip ahead to my last slide, which is basically saying please reach out to us. We are open to discuss and communicate and talk further about any device or any question you might have about AI/ML, and we welcome any inquiries in that space. Thank you. - Thank you, Vinay. That was perfect. And that's really what we wanted to do is just sort of give a little bit of an overview, a little bit of an intro, as sort of what other regulators are doing.
So that's perfect. And I completely agree with you, hopefully, we're gonna get some discussion some of those concepts that you raised. So I'm gonna break the gentleman's code a little bit, and I'm...
I know we should sort of let our guests go first, but I'm gonna butt in line. I'm gonna give you the Health Canada presentation now, and then let Johan from the UK's MHRA bring us over the finish line. So I'm just gonna bring up my screen, and I hope everyone can see that. Can I have either Johan or Vinay let me know if that works? Yes? - [Johan] Still waiting to see it. Oh, yeah, we can see it.
- [Marc] Looks good. Perfect, all right. Sounds good. So I'm gonna move fairly, fairly quickly for my 10-minute slot. And I know I'm repeating myself a little bit from a session that I did with this group about a year ago, but I wanted to provide this so you can compare and contrast with some of our partner regulators. I've included the definition of medical device here on the left, 'cause I wanted to demonstrate that really software alone, that may or may not have a machine learning model associated with it can actually fit this definition.
So again, I always typically start off with that. Our Canadian regulations have essentially three parts. The first part is general licensing, where you can sale, sorry, you can sell or import into Canada.
That's under Part I. We have a Special Access portion, where that's essentially access to custom or unlicensed devices for specific patients, for specific clinical uses. And the third part is our investigational testing, or most people call them clinical trials. On the bottom, I'm showing that the products that we regulate are based on risk.
And in Canada, there are four risk classes and that's sometimes different, depending on which jurisdiction you're looking at. So the Digital Health group, we were created almost five years ago now. Our core function is a pre-market review division, but we were given the mandate to also advance and adapt some of our regulatory approaches to emerging tech, like cybersecurity, AI, and some of the stuff we're gonna be talking about today, And really to help support some of our policies moving forward. So I've chosen to list a few technology types that we are responsible for reviewing. So just a few, diagnostic imaging systems, surgical robotics, radiotherapy, radiosurgical devices, they all come through our Digital Health team. AR/VR devices, just to name a few.
And often, I describe our Digital Health group as what we were actually planned to be when we built the group of five years ago. We were supposed to be the Radiation Emitting and Digital Health group, right? So for those of you listening in, if you've got a radiation-emitting device, it'll likely come through the Digital Health group as well, at least in Canada. Next up, I just simply wanted to share with you three relatively pertinent web postings that are relevant to digital health.
An older one that's been up for years that I won't speak about on the left.. But one that we jointly posted with our friends at the FDA and MHRA, as well as a new one related to electronic health records that contain medical device components or modules. The one I'd like to highlight for the group is really the joint publication on "Good Machine Learning Practice."
You heard Vinay bring it up. I'm sure Johan's gonna bring it up as well. And it highlights very high-level key concepts that we expect manufacturers or innovators to consider, right? Things like clinical data, being representative of the intended patient population, training sets and their independence from their test sets.
Vinay spoke a little bit about transparency. The last principle is actually deployed models that are actually monitored for performance when fielded. So the last point on performance monitoring, I'm gonna speak a little bit about in a minute or two.
And given that this panel has representatives from the FDA and MHRA, this posting is something we'll likely talk about more in this hour. I want to share that Health Canada is planning a relatively technical guidance document on the topic of machine learning-enabled medical devices. We're hoping that this guidance will be posted, fingers-crossed, in the coming weeks. And while I won't necessarily read all the considerations we're planning to touch on, we're intended to communicate our current expectations around things like management of bias. You saw that at the end of Vinay's talk.
Model drift, obviously, the separation between training and test sets, transparency, and that's a big one for us. And you saw it from Vinay, that's also a big one from the FDA. Essentially, we want the end user to understand what the algorithm or the model can and cannot do, right, so that they're fully informed. And we also want you to consider processes and mitigation or sorry, which process are in place to ensure acceptable ongoing performance. And essentially, we wanna make sure that those models aren't drifting.
And I have this, and again, I'm just gonna dive a little bit deeper for the next two or three minutes or so. And one consideration for Health Canada is model drift, right? And what is that? Model drift, some people call it model decay, is simply the degradation of performance over time. And I would say, in the last couple of years or so, we've been regulating a locked algorithm. Essentially, just the model's trained. It's locked. It's deployed.
But drift can actually occur, right? The first way can be, well, actually, there's many different ways drift can occur, but a couple of ways. One of them being data drift, and that's essentially the change in input data, as well as concept drift, and that's essentially the environment changing around the model and those relationships that were originally found aren't necessarily valid anymore. So it doesn't really matter whether drift is occurring due to data drift or concept drift, but machine learning model performance can degrade over time, and that's not something that is very typical for with deterministic-type algorithms, like Microsoft Excel or calculators, right? Past performance may not equal future results, right? You may see that at the bottom of your investment statements, right? And that's upsetting to Beaker here, like scientists like us. And what I wanted to highlight, that even our own Canadian Medical Association published last year in September 2021, and recognizing that model drift is a threat to the performance of these models.
So looking at the little graphic on the bottom right, perhaps regulating these models as locked really isn't the best solution and we need to at least monitor performance. I know I'm running out of time, but I just wanted to highlight that this is real. This isn't theoretical, right? There's a couple of publications. One, on the left, from Radiology. The one on the right is a Nature publication.
Essentially showing, and I won't go into details, but essentially showing your Y-axis is model performance and your x-axis is time. So no matter what, these particular models actually degraded over time. The one on the right is an interesting one.
And this is a model that predicted hospital admissions from data collected from an emergency department, actually in the U.K. Johan, I included this example for you. But similar to the one on the left, that green line represents model performance versus time. And what happens in March of 2020? The model starts failing miserably. What happens in March, 2020? Well, we all know, right? COVID hit. The input data changed and the environment around us changed, right? Age of patients changed, admission rates spiked, trauma patients dropped, right? Anyways, so drift does occur.
So as a regulator, I wanted to highlight that that's something that we're doing. I'm simply highlighting here that, in our Canadian legislation, we have the ability to put a term or a condition on an authorization prior to market entrance. And that's what we're tending to do, at least very recently, for some of the higher risk, or what we deem relatively high-risk products that we wanna essentially keep a leash on those products and wanna monitor performance in Canada on Canadian patients. So we ask for, essentially, an annual report or a report on performance, so let's say six months out or a year out.
This is, I think, my last real slide. And we're talking lots with our international partners, right, and about frequent updates to models. On the left, that's the current state of AI regulation, at least in Canada, right? The algorithm is trained, locked, deployed. But if that model is to be retrained, it needs reauthorization, and that leads to weeks, months of delays or approvals, and really delaying patient access to some of these innovations.
So on the right, that's the ideal scenario. Fingers are crossed, right? The algorithm has access to data. It learns and improves and benefits the healthcare system.
The challenge is how do you regulate a product that varies in time. I'll leave it there with a little bit of a cliffhanger, hoping to generate some discussion after. My email address is here. While I can't promise, necessarily, a speedy response, please don't hesitate to contact me and I'll do my best to either answer your question or route it.
So with that, I will pass it off to Johan. - Thank you so much. I'm going to attempt to share my screen. Here we go. Marc, if you could just give me a yell when you can see that, that would be greatly appreciated. - [Marc] We can see it, Johan. Thank you.
- [Johan] Fantastic. Well, thank you so much. It's really a pleasure to be speaking and thank you so much for the invitation, and it's always good to share a stage with international peers and friends, which is exactly what FDA and Health Canada are. I think the UK's view is it makes sense to do this internationally. It streamlines access to market in the different jurisdictions, and also, MHRA doesn't have all the answers. Some of those answers are with our friends in Health Canada and with our mates at FDA as well.
So we can get all this done better if we do it together and also we'll be harmonized as well. So hopefully, some good news there. AI as medical device. Good. Look, here's the honest, broad view I think, 'cause we're sailing the boat as we're building it basically. So the kind of state of the art about how you assess performance of AI's medical devices is not settled yet, but there is a critical need to get these devices to market.
In the U.K., for example, we have the NHS, the National Health Service. We are under the weight of a crippling backlog. The service is not improving.
The need for these devices is there and they can assist, but the method of assessment is not yet settled. So that's an comfortable position to be in, but that's exactly where our regulators should be. We should be looking at the state of the art, considering what innovations can we get across the line to help patients and to help clinicians do their job. So it's uncomfortable, but it is exactly the position where we should be. So in the U.K., we do an assessment
at the very end of 2020, which we renew occasionally. What's missing in our regulation to get the regulation of software and AI right? Broadly, what we thought is, we have the legislative footholds already. Our legislation is broadly right for software and we need to update it more broadly, but we have the tools we need and the general medical device methods remain sound. So software and AI as a medical device is, ultimately, a medical device and those principles remain sound. But really, what was required was clarity, primarily via guidance, about how to meet those broad medical device requirements for software and AI. It was translational but also streamlined processes that work for software and AI.
So for instance, predetermined change control plans or modification control plans, which Vinay talked about earlier. And also, the tools to demonstrate conformity. So principles and processes are not enough. If industry don't have standards to demonstrate conformity, then the rubber will never meet the road and conformity will always be patchy. And also, we want to provide a joined-up offer with other partners of our health systems.
So MHRA are only one regulator amongst the network of many. So we kind of need to work with them and provide a global offer to make our market attractive and to make sure patients have access to these devices. So we've done, hopefully, some significant progress towards this.
So we released a government response, which basically detailed the legislative changes. We did that in June of this year (2022). There is a chapter on software as a medical device. Again, it's legislatively liked.
We don't intend to make a huge amount of changes, but we intend to focus in on business change programme here. Such change programme details, guidance processes, all sorts of other things, experimental work, data science work to hopefully make our regulatory regime for medical devices fit for purpose for software and AI. So here's kind a brief overview of what we're doing in the UK. So we published the roadmap for the change program on the 17th of October. So it's relatively new.
It details much of what we intend to do for legislative change, guidance, development processes, and standard development as well. So there are 11 work packages. The first eight cover the life cycle of software as medical device.
So clarifying what qualifies as software as medical device, how it's classified, pre-market requirements, post-market requirements, details on cybersecurity. There's three work packages on AI in particular. Across that though, there are 33 deliverables. So we're, hopefully, delivering a lot.
I would challenge anyone in market to say it's not ambitious enough. It's going to be a real issue trying to deliver it, but that's exactly where we should be pushing our resources to get this done as fast as we can. We're gonna publish our first five before the end of 2022.
We also have some wider work as well, which I'll talk about in a second. So here's how the roadmap was written. So it's legislatively light. We're not doing a lot in legislation. We don't plan to bring forward a whole AI's medical device, separate piece of legislation.
We didn't think there was a point in doing that. We didn't think that would be helpful. We developed it across government. So it's not just an MHRA view. It's working with our health system.
It's working with partner regulators. It's been supported by a wider industry and patient engagement plan that we plan to bring forward shortly and publish. We're also tackling bias directly as well. So we heard loud and clear concerns, especially over COVID, that bias will impact patients directly and we agree.
And AI as medical device has the capacity to reproduce biases that exist or even reproduce new biases that humans don't have. We need to make sure that's a world that doesn't come about. We need to make sure that AI helps rather than hinders. Also, international harmonization is at the heart of our approach.
If we can do it with our partners at FDA and Health Canada, we will do it with our partners at FDA and Health Canada. If we can shove a document out publicly, and say to our partners across there, "What do you think?", that's exactly how we will work and that's what we're already doing. We'll also have a focus on standard development as well. So we'll be producing something with BSI, that's the British Standards Institute, that demonstrates what we're doing in the standard space to make sure we meet that mark.
And also, we recognize, I have no idea what I was going to write for that second bullet. Why did I write that? I have no idea what that means. So I'm just gonna skip that bullet. Apologies.
There are three core challenges that we need to get right for AI, three core challenges it poses though. So the first is the evidence base. It's trying to shore up what that evidence base looks like. We know there are some issues currently about transparency around, say, the limitations of models, where they work, where they don't, the generalizability of those models.
Really, Good Machine Learning Practice Principles is the start but not the end of that work. We need to progress that and build on that work to shore up the evidence base for AI in general. The second kind protocol is AI interpretability. So AI can be a black box. We don't necessarily know exactly how it came to the conclusions it does at some points. That can have two big impacts.
First, it can change the kind of evidence you'll generate as a manufacturer to get to market. So it can potentially push you into the post-market phase a bit further than the pre-market phase. But also, it can impact the performance of the human AI team. So the MHRA are primarily interested in the performance of the human AI team.
So if the performance of the model is 100% sensitive and 100% specific, but the performance of the human AI team is not, then that is still a safety risk to the patient. The patient is still harmed. So we're really interested in the performance of the human AI team and highlighting that opacity might have a key relationship with that performance. And finally, there's a work package on AI adaptivity.
So AI changing across time. So we're working, again internationally, to try get some state of the art developed there. But also, we have some work that will soon be released that involves some data science, which actually mirrors some of the work that Marc presented there, demonstrating the ability to track change across time using machine learning models. So to detect when performance drift has occurred or concept drift or drift detection. So we've deployed a number of machine learning models across data sets related to COVID, data sets related to cardiovascular data, and then also a toy dataset as well to basically demonstrate a toolbox of methods to detect change in AI as a medical device. Again, we need to know that change has occurred, then detect whether that change has improved the performance or reduced performance.
So that's what we're doing. Again, we've all said GMLP was released last year. Again, I think this is the start, right? You can see the ambition.
This is always intended to provoke standards development, to provoke further work. But I don't think the job of Health Canada, I don't think the job the FDA and the MHRA is done here. We need to advance that work at pace to ensure these principles are filled out and supported, both internationally and nationally. But it's the first shot in doing that. Marc, I'm hoping I got you some time back on the schedule. Always happy to take this further in discussion.
- It was perfect. I think we're right on time. A couple of minutes into the panel discussion, and that's a perfect segue.
So thank you, Johan. Thank you, Vinay, for those presentations. Before we get into a little bit of a panel discussion, I'm hoping to probe the attendees a little bit in either asking your questions in the chat. I think we have a raise-hand feature. Ahmed can help me if we don't. But essentially, just get your questions in ahead of time, and that way we will be able to gauge whether or not we want to move away from panel discussion and take all your questions or keep discussing amongst ourselves.
Ahmed, can you just recap how to ask a question. - [Ahmed] Yes, people can either post the question in the chat or they can use the raise-your-hand feature, which should be accessible. - Perfect, thank you so much.
So I was given the task to moderate and I think I'm gonna use my moderator's prerogative a little bit. And I've prepared a few questions for our guests. I have no problem weighing in as well, so I'm gonna have an equal opportunity I hope. But I had the chance to draft these. So I'm hoping to get a little discussion going amongst the three of us. They're relatively broad questions, but I'm hoping to hear kind of how they either align or contrast with the Canadian perspective.
So maybe, first off, and maybe what I'll do is I'll pose this to to Johan first, and then Vinay can probably chime in and I can as well, and this is starting off in first gear. So in your opinion, right, what is the biggest challenge in regulation of AI or machine learning in healthcare and why? - So I think reasonable people could disagree, and I'm hoping both of you'll be reasonable, and you might even disagree to make sure it's interesting for the audience. So I think that the one I would hit on is generalizability, so knowing whether your training and test set fits the real-world application. Other issues, such as bias, could be viewed as issues of generalizability, at least strictly if you construe it in terms of performance.
And then transparency is kind of being clear about the limitations. But one of the key limitations is a lack of fit between the training and test data versus the real-world environment, and there is no substitute for just knowing that. So often deploying in silent mode, for example, is one key mitigating, let me start again, one key mitigating way of trying to figure out whether your training and test set does generalize from one hospital to another and whether it fits that population. But there is no good way around that apart from just generating the data. So again, it's gonna be a really hard problem and it's not necessarily a problem that is fully within the regulators remit, right? The ultimate solutions involve the gathering of data. We have a role in opening up those doors and accelerating those measures.
But the ultimate answer involves data, essentially. Yeah, I don't know whether you agree, disagree, or disagree violently. Any of these options, of course, they're available to you. - Yeah, so I can step in.
I mean, think you said generalizability, but went into a lot of different challenges within that scope. So it could be more narrow in focus. My perspective is that, I think, the bigger problem is lack of transparency, and primarily the fact the two aspects of transparency, right? I mean the fact that on one hand the issue of how do you explain how an algorithm came to its decision, right? The glass box that you talked about, Johan. On the other hand, what's the definition of complete transparency, right? And that's not really clear, because different stakeholders have different interpretations of what transparency means.
A common consumer who's using an iPhone or a smartphone may have different expectation of how transparency you should be to really have to explain how many levels in a neural net are there for, how the algorithm decided. Because they may not care for that. On the other hand, even a clinician may not care because they may need to know like, "I'm in the ICU.
I need to treat this patient and I made the decision." How confident can I be that your algorithm did the right thing? Versus a regulatory body would probably want to know more about, okay, how transparent is the way your algorithm constructs data and makes sure that the evidence aligns with the conclusions it's coming to. And I think that's a problem. And I think the other part of the problem is also, from a transparency perspective, how are the output's being used by humans and/or other AI/ML systems when they're talking to each other, right? Especially in decision-making, I think that's a little bit challenging, I suspect. I hope that helps build the debate.
- Yeah, I think, those are all really, really good points. I think the generalizability aspect, at least from the Canadian perspective, is a really, really big one. We are not a large market.
So typical products come in with test sets that are not Canadian. So as a regulator, that's a huge problem. And as a regulator, you always wanna balance that innovation and access to your market versus is this gonna generalize to the Canadian population. So I think the generalizability one, for sure, at least from the Health Canada perspective, is another one. I'm gonna throw another wrench into the mix and it's unlocking the true potential of what machine learning can do in the temporal aspect.
For a regulator, and I touched on this a little bit in the talk, as a regulator, we want, I think, Johan, you used the word state of the art at the top of your presentation, and really, we want the state of the art for our patients. Well, how do we do that? Well, we give them access to the trained model. Well, how do we do that? Well, it's just this constant iteration of regulatory oversight. So this, I don't wanna say continuous learning, but maybe, adaptive or batch learning is really important to us to get right, so that our patients get the most up-to-date model. And you saw that the performance degrades over time. And then- - I think- - Yeah, go ahead.
- I was gonna say that it also goes to what Johan mentioned about building the ship as it sail but then the problem is we are going to make mistakes as we learn, right? And the question is, how do we make sure that the mistakes are not impacting patient health? - Yep. - And hopefully, as international collaborators, we can learn from each of those mistakes and not repeat them. - I think that the starting point from the MHRA perspective, and it'd be interesting to see if you both agree, is that we're ultimately interested in the performance of the model. And data drift is an equal risk, or perhaps even more than the risk of retraining. So currently, the system is orientated towards data drift occurring and manufacturers not having the flexibility to keep their model relevant and performance as well as possible, which is equally a tragedy, right? So we need to get both sides right. We need to make sure change can occur faster, but that change is assured, I guess.
But currently, our systems, at least in the UK, aren't set up to support that and that is a shame. And we need to change that as fast as possible is our view. - Yep, completely agree.
So there's almost a two-sided approach, where the manufacturer must be able to retrain, right, and the system, the framework, the legislation, needs to be able to keep up as well. So completely agree here. I'm gonna move to a second one, 'cause we all spoke about it. We all spoke about Good Machine Learning Practices. So I'm gonna put everyone on the spot a little bit. And I've been asked this question in fora like this.
If you could focus an innovator's attention on a principle, there's 10 principles right there, which one would it be and why would you say that? So maybe, Vinay, I'll start with you just to switch it up a bit. - So my initial answer would've been all of them because all matter and they're so high-level. I mean, seriously, you shouldn't be ignoring any one of them. But I think, if you're asking me to choose, and I think I'd probably choose two of them, because I think the last two, which is I think, depending on how we read them, number wise. A toss-up for me is between users are been provided clear, essential information, and the second one is that deployed models that monitor for performance.
Now, the reason why I chose those two, basically, they put sort of time nicely with the need to be aware of the other principles, right? If you aren't aware of the other principles, then there's no way you can do these two. And the addition of doing these two is, first of all, it goes back to the point of, A, transparency, B, generalizability also. And the fact is that it allows you to keep your users front and center when you're developing your product. As an innovator, you need to make sure that you're not developing a product nobody can use and you want to build clinician adherence to your product. So I think that makes more value in that. And the other aspect to it is, if you're measuring the performance of the product in real world, it allows you to know how far apart your pre-market data is from the post market.
Because once it goes out in the real world, the performance is going to deteriorate, and so the question becomes how much of a device buffer do you have? How much of safety factor do you have in your data or the algorithm to handle the variation that you're gonna see in the real world? And I think those are key factors, I think, which allow you to make sure that you're building a model more robustly. - Thanks, Vinay. Any response, Johan? - Yeah, first of all, Vinay cheated and picked two instead of one.
So that's the first response. But no, I think I would agree that the user information one would be the first one I would hit upon. So the transparency approach.
So I think my biggest challenge I said was generalizability, but regardless of that, I think the way you start is with transparency. So no regulator, including I think all the ones here, expect your model to be perfect. There is no such thing as a perfect training and test set. Everyone has gaps, everyone has even a small amount of bias in there. That the best way to counteract that is with transparency, is knowing the limitations of your model and clearly communicating that to your end user and to regulators, right? So flaws can be forgiven, they can be patched over, they can be solved across time. But if that doesn't start with transparency, then that creates safety risks from the outset and is probably the best way to draw the ire of myself, Marc, or Vinay, if you're a manufacturer for example.
Marc, does that make sense to you? - Yeah, absolutely. I think that's a really good one and thank you for sticking to the rules. You know what? I'm gonna break my own rules in the sense that I'm not able to identify one. I don't think I'm even able to identify two.
But whenever I'm asked about this particular document, one thing that I try to provide advice to manufacturers and innovators on is the need for data and the need for good training data, good test data, and good data management processes. Vinay made a great point about they are relatively linked between them. And for me, reading them, almost all of them are based on data. So again, have good training data, have good test data, and have good data management processes.
And if you have those, it'll be easy to cover the other principles. We have 15 minutes left. We have a few questions. I'm gonna ask maybe in a lightning round, because it's something that has been brought up many times.
We talk about transparency a lot, and while we can talk about it sort of conceptually, a lot of the attendees, I think, are really interested in sort of, well, how can I employ transparency, or how can I do a better job in transparency? So I'm gonna ask sort of what are some of the ways that maybe some of the people in attendance today can promote the adoption of the tools that they're developing and the trust in the AI systems that they're developing, right? So maybe, Johan, I can start with you and go to Vinay. - I think I'm gonna fall back on what the initial evidence says, I guess, which gets clinicians and patients' trust, and it's to be built from their needs and to communicate the limitations of the model and key information based on what they're asking you to provide, I guess. So what I'm saying is transparency, that the way to get transparency right is to not consider your user base to be homogenous, to not consider your user base to be the same as a regulator versus, say, the procurer of that device as well. I think you have different stakeholders that require different forms of transparency, that require different kinds of explanations. So the kind of transparency that I think, Marc, Vinay and myself would be wanting, as a regulator, would be rather more in-depth than your regular clinician. Using that device, and that is good and proper, but that clinician still needs a set of information to help them assess whether that device is correct for their patient and what its key limitations are.
So I think that would be the first. It's not a solution, but it's the first kind of key I think to getting transparency right is to consider who your stakeholders are and what they need to know and what they want to know as well. If it's driven by what they want, then that will be the best way to secure their trust and to make sure your device is trustworthy as well. So no easy answer. It requires hard work and those usability studies are difficult as well. So yeah, hopefully, that's helpful.
- Absolutely. - Yeah, Marc, I don't necessarily have much more to add to what Johan said. I mean, just some things I was thinking about is the fact that, when you're thinking about unlock systems, like where machine learning algorithms are gonna be changing quote unquote, "on the fly", how are we gonna make sure that the stakeholder, the clinicians who are gonna be using the version of the model that has changed from the previous day, they know that the device limitations and labels have changed. And so it goes sort of to the transparency, but it also becomes a question of how much information are you gonna inundate your users with saying, "Hey, my algorithm has changed." This is the intended use now. This is the organ you can look at.
These are the populations you can look at. Next day, you may have a different population. So I mean, that might be a very drastic case, but at some point it's gonna happen that your algorithms are gonna be changing dynamically.
And so the question becomes how well are you gonna keep your intended user up to scope? And I think that's gonna be tricky. - Yep, very good point. And that's the only thing that I could have added. And Vinay, you already did, was the intended use, right? So Johan brought up key information, knowing who your users are, right? And that clear intended use is super, super important. And as regulators, I think we can all agree is that I would much rather have a product well-characterized and those limitations well-characterized and the user knowing what those limitations are rather than the company saying, "You know what? This model is great and it's perfect."
It's not. And and just make sure you communicate that. Let's move to questions. Thank you so much for that discussion.
I think we're well-aligned on a lot on that front. I'm gonna read the first question from Samir, and again, please forgive me. I don't see any hands by the way, so I'm gonna go straight to the chat.
The question from Samir, "We are working with FDA on our 510(k). Is there a place or a document where we can see the testing requirements globally from Health Canada, MHRA, and FDA, so we don't miss out on any testing when down the line we apply for Health Canada or MHRA? Any guidance or input would be helpful. Thanks. - And I see this question is for Health Canada and MHRA. - Yeah, I mean I think that's fair. What I'm gonna ask, and again, I'll take the hit on this one, and again, colleagues jump in if I miss anything. I think testing requirements are very, very specific to what the device is.
So the example I always use is, is again, it comes down to your intended use. Are you intending to detect a clinical pathology or are you intending to rule out a disease? Well, the testing is different for that. So no evidence really is applicable to all. So that's point number one.
I think while there's no one place or one document to support what MHRA's position is on testing and FDA and Health Canada, I hope that the guidance document that we publish in the next few weeks has a little bit more information on testing. One thing that I like to talk to industry about is keep your test set as independent as possible from your training set. And, you know, testing just sort of shows generalizability.
So Johan had mentioned a little bit that lab to the world, in many cases, that performance drops quite a bit. If you are training and testing in Canada, how does it generalize to the other jurisdictions and do you need any prospective data or not? And there are cases, at least from my perspective, that they're not. And robustness testing, again, that's something that I like to talk about as well, is you have a model, it seems to work. Throw some garbage at it and see what it does.
That's something that we'd look to make sure that that model is relatively stable. So again, verbal advice, but I don't think there's a one-stop shop. Johan and Vinay, anything to add there? - I think... - Sorry, go ahead, Johan.
- Thank you That's very kind. I think you're exactly right, Marc. And what you demonstrated there is that we'll be asking exactly the same questions and almost the same way. And so would our approved bodies that do the assessment for class 2A above devices. So that same methodology more or less applies, right? So I think that demonstrates harmonization, so I would definitely support all of that. Build from your intended purpose.
Consider what state of the art is. Make sure you can demonstrate that state of the art and Marc's given you a few tips about how you can do that, in particular for AI and ML, which we'd all expect to see as well. - And the only thing I was gonna add, two things I was gonna add, was one is consider the risk category for the device when you're building some of these tools. And the other thing is, if there's particular SAMD that you're developing, is focused on the population, then you may or may not have that similar population available in the other countries. So the question is whether your algorithm is gonna be behaving correctly in those countries. Something you may wanna keep in mind.
- Yep, good point. Just in the interest of time, I'm gonna move to the question from Mohammed Ismail. My question is somewhat lateral. The question is, "What have the various bodies done to provide more academic and training posts for specialty trainings in the UK or elsewhere to encourage innovation and provide the necessary teaching that allows for accepting innovation within the confinements of regulatory bodies?" So Johan, maybe just because it says UK in there, I'm gonna go to you, and then we can add if necessary. - Fair call.
So that doesn't necessarily sit exactly within the remit of MHRA, but we partner with an organization called Health Education England. So they've released two really, really good reports on how to build confidence in AI from a clinician's perspective. They've done work breaking up clinician's view, I should say healthcare workers in general, into different archetypes to consider what those different archetypes need and what would be best to generate confidence from the healthcare workforce, and also what training is required to get AI right and to get workforce trained appropriately. So that work is being done by our partner organization Health Education England. I would highly recommend you go look at those reports. I'll drop them in the chat as well if anyone's interested, if I can be fast enough before this closes.
So I'll take an action to do that. I can always send them to Ahmed as well. So I highly recommend that read. I can't speak tonight, which is unfortunate cause I'm on a panel, so sorry. Vinay, across to you. - Yeah, the one thing I was gonna add is we do have something called CERSI, which are the Centers for Enhancing Regulatory Science Innovation, which are funded initiatives of several like institutions around the country.
And those are opportunities where we do explore innovative ideas in AI/ML, and so I think that would be one space you may wanna think about. And the projects are, I mean, there's information on the network internet for what kind of projects are funded, and some of the projects include like looking at how AI algorithms can miss on subpopulations and also how do you look account for drifting of the data that the AI algorithm is looking at. So some of those kind of projects are actively ongoing within that space.
So definitely, look at, CERSI has opportunities. So that's C-E-R-S-I, and look at those as potential places where you can get more knowledge on the space. - I think in the interest of time, I'm just gonna move on.
I think those are two really good answers. If there's a question that's specific to Canada, Mohamed, please just send me an email, okay? You have my email at the end of my deck. Helen asks, "What are the expectations for how training and testing data is collected? To what extent do we need to document the source of the data to show that it's validated, for example, the amount of metadata to be collected versus minimizing personal data from a GDPR or HIPAA perspective?" It's an excellent question. Perhaps, maybe what I'll do is I'll let my co-panelists chew on that a little bit. I will speak from very much a regulator, very much from a federal official in the sense that we expect that data is collected appropriately according to other legislation that we don't necessarily administer or regulate on.
So we expect that data to be collected properly. But once you have the data, then we're looking our fence is essentially the safety and the effectiveness of the product and not necessarily the data collection. That's not to say that we won't be interested in what that data is and how it's characterized. But the data collection is, at least from my perspective, from Health Canada's perspective outside of my jurisdiction. - So the only other thing I was gonna add is it depends on I mean what your tool is being utilized.
For example, if you are using a digital tool for a clinical outcome assessment in a pharmaceutical trial, then in those situations you probably wanna be on top of this data, so that if there are missing data you can go back and look at it. So to some extent, it also becomes more about how we can figure out what's going on with the data and how we can troubleshoot what's going wrong with the algorithm. So the more information you keep to help build your model, I think it's better for you as a sponsor or an industry participant that you can debug your algorithm, make sure it's performing in the places it's supposed to perform in those kind things. I think from our perspective, I think it's valuable for you to be more on top of your data as much as possible. - And just to echo some thoughts as well, we have the same expectation that Marc outlined as well that you're consistent with the UK GDPR and wider patient confidentiality rules. But also, just to point out that the key of dependency of knowing where the data's come from to ensure that the model is correctly trained in outputs.
It's kind of garbage in, garbage out. So knowing where that data's come from can give you key indications of where the bias might be in there, for example, how you collected it and so on. So while there is a cost that metadata in terms of personal data, sometimes that cost is often worth paying to have confidence in the performance of that model. So the MHRA, in our own jurisdiction, are working with our data protection regulator to hopefully make those routes clear and to work with other kind of data custodians to make sure that data's available 'caus
2022-11-24 20:53