Luminaries: The future of data and AI in Australian healthcare
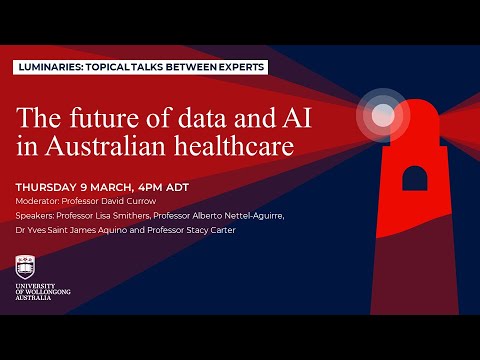
Good afternoon. I'm David Currow, Deputy Vice Chancellor and Vice President of Research and Sustainable Futures at the University of Wollongong. It's a pleasure to welcome each and every one of you here today to our Luminaries webinar series. Luminaries brings together leading University of Wollongong researchers, industry experts and thought leaders for a one hour conversation every fortnight. We will discover how research and collaboration at the University of Wollongong is tackling global challenges. Today we're hearing from a group of exceptional researchers as they discuss the role of data and artificial intelligence in Australian healthcare.
But before we start, I would like to acknowledge Country. On behalf of the university, I would like to acknowledge that Country for Aboriginal peoples is an interconnected set of ancient and sophisticated relationships. The University of Wollongong spreads across many interrelated Aboriginal countries that are bound by the sacred landscape and intimate relationship with that landscape. Since creation from Sydney to the Southern Highlands to the South Coast, from fresh water to bitter water to salt, from city to urban to rural, the University of Wollongong acknowledges the custodianship of the Aboriginal peoples of this place and space that has kept alive the relationships between all living things. The university acknowledges the devastating impact of colonisation on our campuses, footprint and commit ourselves to truth telling, healing and education. Data are now at the center of human lives.
Artificial intelligence built on healthcare data promises a new and transformative kind of health technology. However, this raises big questions. The promised benefits of big data and artificial intelligence be reached and then delivered. And if they are, what are the ethical and social implications of sharing and using big data and employing artificial intelligence based technologies in health decision making? It's my pleasure to introduce you to our researchers today. Professor Lisa Smithers is an epidemiologist whose research mostly encompasses perinatal and pediatric epidemiology.
Much of Lisa's research involves the use of population based datasets and data from large cohort studies. However, she also conducts clinical trials in hospital and community settings. Lisa has a special interest in the application of methods to improve causal inference from observational studies. Professor Alberto Nettel- Aguirre has developed his career working collaboratively in health and medical research and has worked extensively as a biostatistician in a range of projects, namely around pediatric nephrology, neonatology and injury prevention. His expertise and interests cover the correct application of biostatistics and the implementation of statistical learning methods in health and social research.
Alberto is one of the University of Wollongong's representatives at the Australian Data Science Network and leads the Center for Health and Social Analytics within the National Institute for Applied Statistics Research Australia at the University of Wollongong. Professor Stacy Carter is the founding director of the Australian Center for Health Engagement, Evidence and Values in the School of Health and Society at the University of Wollongong. Her training is also in public health and her expertise is in applied ethics and social research methods. Stacy's research program addresses the ethical and social dimensions of four key challenges for health systems using artificial intelligence, detecting disease in populations and individuals, reducing harm and waste, and encouraging vaccination. Dr. Yves Sain James Aquino is a philosopher and physician with expertise in philosophy and ethics in health care.
He is a post-doctoral researcher, research fellow at the Australian Center for Health Engagement, Evidence and Values. His research projects include the ethics of artificial intelligence, the ethics of cosmetic surgery, body image research and social justice in public health. Before we begin, I encourage everyone online today to submit their own questions using the Q&A function. We will try to get through as many questions as possible. However, to kick things off, I'd like to ask the panel about data and artificial intelligence in Australian healthcare.
Where are we today and where could we be in the next ten years? I'd like to kick off with some thoughts. Well, everybody knows I never keep my mouth shut, so I'll just start. Why not? You know, I think it is very important. There's several things
that we need to be thinking of. What we understand by what people think is a AI in health care. You know, things like dictation or voice recognition on medical note is already AI, CAT scans all of these things that we use, the tools, are they AI when it comes to using it as a tool for diagnosis or for actual treatment? I think that's the big difference on what the expectations of people are. So where are we? I think we're still at a baby steps in a way. Where could we be in ten years? It will depend a lot on what people decide to do regarding data, data quality gaps, collection, etc..
That's my first go at it. Fantastic. Thank you Alberto. It's interesting you say that. I love it when when I think about this, I actually think back to five or ten years ago. And for those of us who've been thinking about artificial intelligence and healthcare for a while, there was a lot of hubris around five or ten years ago.
You know, there were there were big tech companies saying that they were going to build learning health systems that could absorb all of the data from a hospital and could do almost everything. There were leading developers who were saying radiologists would be extinct in five years and and we are training them now. But there are these huge claims being made at that time. I feel like that's become a lot more measured now, and I feel like that's really shifted just in the last couple of years, actually.
But I think there's still quite a lot of excitement about the promise of artificial intelligence built on data. You know, that you can't have artificial intelligence without data that's so interconnected. And I'm sure we'll come to that more as we go. But there's a pattern, I guess, that's connected to the difference between the hubristic claims a decade ago and what's happening now that, you know, you see those headlines all the time 'AI beats panel of seven radiologists', you know, similar kind of shape of headline. And that's often based on research that's done by the manufacturers of the systems, research that's based on really quite artificial datasets, you know, synthetic datasets that have been essentially changed to make it easier for the AI to learn to do the thing that it's meant to be doing. And then when you get those AI out into the wild, right out into the real world, and when you have really good quality health and medical research done on their performance, they often don't perform as well as they did in those quite artificial situations.
And I think what's changed recently is that the clinical and public health community has really come to terms with that problem. And they've really started to talk about, okay, what do the standards need to be for AI research in health? And maybe they need to be higher in health than they are in some other places. How can we evaluate real world performance? How can we make sure that these algorithms actually are fair, not just that they work, but that they don't treat some people unfairly relative to other people? How can we make sure that patients and clinicians and the public are involved in setting the agenda and in development? So I feel like we're at quite an exciting point. But like Alberto said, we actually really have to make some commitments at this point to make sure that this technology goes the way that that we want it to. And Yves has been
been talking with quite a lot of experts about this kind of issue, actually. How do we make this transition to the right kind of AI? I agree. And just to echo on that, especially what we've learned, what we have experienced during the pandemic about a lot of parts of the Australian healthcare system. They're really interested in the advantages and potential benefits of artificial intelligence and how it can automate things as well as support workforce, because at the moment Australian healthcare workforce would really need a lot of help, especially when it comes to screening programs. But at the moment what is happening is that Australia tends to import a lot of these systems instead of developing our own. And there are benefits. There are trade offs when it comes to that. But one of the key
challenges when doing that is that these AI systems developed elsewhere are built on data based on the local population and people assume that it's like any other technology where you can copy/paste the technology from one area to the next and have no problems. But as we are finding out, we need to understand how AI systems work in local populations. So I think that's what we have to look out for in the next few years, not just the excitement about the advantages of AI, but how can it work best within the Australian context. Can I pick up potentially, Yves, on your some of the things you're saying there. AI is built on data most of my work has been on big data really more administrative sources of data. And those systems can't work unless we get data quality right in the very first place.
So based on my experience, I think over the last 15 years of using administrative data, I would actually propose that the quality of that data has improved along the way. But I think that it still depends a lot on how data was set up, who set it up, for what purpose and how it's used by people entering that data. So that sort of fits a little bit with what you were saying Yves. My area is perinatal health, and so with administrative data, that perinatal data is collected extremely well across the whole of Australia because we have standardised definitions and fields, we have really good reporting systems for feeding up the quality of that data.
And yet, although administrative data is great for these purposes, it's collected with a health service in mind. Yes, rather than other purposes. So I think that's something we really need to think about. If we think about the potential of AI sitting on routinely collected data. And perhaps just one more thing I wanted to mention here is what I perceive as being a current and potentially a future problem is having data analysts, fully qualified people who can actually do the work of analysing the data.
So most organisations are collecting data of some nature. Every organisation needs people to analyse that data. So who's going to do this work? I think we still don't have enough well qualified data analysts to be able to do this kind of work. So I think there's a gap in the market here, and although UOW is trying to do it, it's really hard to find people who know and understand these systems to be able to just design them. And just very quickly add to that not only who's going to do it, but they have to be well rounded, right? Not only number crunchers, then that's something that we need people and everybody needs to understand. Because a number cruncher without a well-rounded idea of how it's going to impact is not going to be the kind of people we want creating the systems.
Yeah, it's so true. And, you know, as we think about that, these are not biostatisticians. This is not what we've been training for the last century.
This is a new workforce that can deal with very large numbers with new tools. Stacy, I'm really pleased that that people are still training radiologists. I think there's a new axiom in the 21st century, which goes 'artificial intelligence will not replace doctors, but doctors that don't use artificial intelligence will be replaced'. And and so as we we think about the the challenges of the future, how do we prepare a workforce not only, as Lisa says, for the the analytic programs, but as you've pointed out, Yves, how do we prepare a workforce to actually understand and interpret the outputs of these programs? Are we changing curricula fast enough in order to do this? Mm hmm.
Well, this is a very complex question, David, but for me in an ideal world, there shouldn't be hard walls or borders between disciplines. And they think there. I mean, there is still a value for general education in general subjects and more technical courses still having some element of social sciences, because as we know, and this is the case for courses like medicine as well, if you don't have any understanding of the social implications of medical or clinical practice, you might not have the tools for empathy to be a more empathetic practitioner. And I think that's true for data science and more technical courses. We need to be able to explain to them, or at least teach them a lot of the social issues that they probably will not be exposed to from studying and then practicing.
So at the moment, that's one of the struggles or challenges that we have discovered in our study, is that there is difficulty in communications between experts. So data scientists have different language versus social scientists versus regulators versus public health experts. And they think we need to empower students to be able to discuss things from experts from another discipline. But I understand that will take a lot of work, but that would be my ideal scenario. And, you know, the other part that we need to be doing and at least we're trying to do here in our Bachelor of Data Science is that it's a full data science, right? Not just data, but science needs to be applied. There needs to be context.
There needs to be a yearning for understanding the context to then be able to apply any technique. It's again, not just techniques for the sake of applying techniques, but what is the context? Do they make sense? We're just getting the data to to be within the context of the problem. And that's how you start realising, you know, that you can get people, you know, to really work in industry or in health. David touched on, you know, the biostatistician tells us of a rare species who kind of tries to straddle the worlds. Right. And I think that's the type of not fully curriculum per se, but formative education that we need to be giving the people that we are creating now so that they can straddle the worlds and be useful. And it really needs to be at all levels of responsibility, doesn't it? I mean, one of the things that we've talked about a lot in the empirical work that we've done with all kinds of stakeholders that I've mentioned before is who should ultimately have responsibility around these systems.
And I think that the final answer usually is everyone's got some responsibility, that the right question is actually which responsibility does this person have? So clinicians need to have the skills to be able to evaluate should I be using this tool with my patients because they have a duty to their patient, know they actually have an ethical responsibility to their patient, to know that the tools that they're using are doing what they think they're doing and they're not going to cause harm. Hospital administrators who procure systems, they need to actually understand what they're buying and not just be sucked in by the hype of the developer, if that is an issue in a particular case. Regulators often really struggling with these systems because they present challenges that weren't really a problem previously when regulators were trying to do with medical devices. So we've got an artificial intelligence system that can adapt constantly, can change itself all of the time based on new data that's presented to it.
That's a much more difficult problem for a regulator to know how to manage, then back in the day when it was really just a physical object that if something went in, then same thing would come out. You know, it was it was a much more predictable beast to try to regulate, whereas now regulators are having to deal with the complexity of potentially changing systems. And then there's the community like we have all discovered GPT in the last year has become part of the public imagination.
And all of us, I think, need to become aware of how AI's working because it's not always obvious that AI's in play, right? AI can be making quiet decisions in the background, so it's really important that everyone is aware of the, the fact that AI is everywhere. And in fact healthcare has been pretty good at being really careful about allowing AI in because healthcare has certain standards. It's helped to hold AI back and to ask, 'Is this good enough yet?' AI is much more prevalent in a lot of other areas of everyday life. So, Stacy, both you and Yves touched on firstly the the inherent biases that can be in artificial intelligence if we don't build it properly and if we don't use the right populations to teach it as as Yves has pointed out. I think that leads very logically to a question around the ethical implications of collecting and using data, because if the data aren't being applied to the population where artificial intelligence is itself being applied, then you know, how do we move forward? So is is there a social license in Australia today for the use of of health data to improve health services and to teach and refine artificial intelligence? It's such a great question, David.
So at the Australian Center for Health Engagement, evidence of values, we kind of specialise in a in a process that takes information to the community and asks them to work together to make recommendations about what should happen. And these are called community juries. So we we ran a series of community juries that were led by Annette Braunack-Mayer, who's a Professor in our center. A year or so ago about sharing data from the health system with private companies for research and development. So it's not always the case that these AI systems are going to be developed by private companies. Sometimes they might be developed inside of health services, and there's often very entrepreneurial and innovative clinicians who notice a problem in their health system that they think that AI could help with. And they have the skills
and they start to develop an application or there can be small spin offs inside universities. So things are things aren't necessarily purely kind of big tech or private, but we talk to people about sharing health data for R&D and private companies. And interestingly, part of this process is people learning. And the more people learn about sharing data and what good can come of sharing data, the more willing people are to support data sharing.
So that's a general kind of pattern that has been seen in a number of these similar processes around the world. So when people understand the benefits, they are supportive, but always with caveats. There's always quite strong conditions around that sharing.
It's not just, I'm sure, go for it, share my data. So generally the Australian process people said it has to be for public benefit. You have to be able to show me that this will actually do good in the community.
It has to be done responsibly. There has to be a clear accountability framework. The data have to be secure that would really matter to people. We have to manage these data responsibly. There has to be proper penalties for misuse. You really have to make it hurt if people use these data in a way that they shouldn't so that there's a real reason to prompt them to be careful with these data.
So there's a clear recognition of the value of the public benefit that can come from this use, but also a real knowingness about the value and the importance and the significance of these data for us and the need to be really careful about who uses them. Yeah. I mean, just a very quickly on data and you did touch on it, but is that need for people to feel comfortable about it, right? What are the processes in place for that data not being hacked, the data not getting access from other places, not being used for things that, you know, I may not really be happy. And we're again, with an ad, we're doing some other project. We're still on the analysis session part of it, but that's one of the things about, okay, what do you believe it should be used for? What do you already think it's being used for? Right. That's the other part. A lot of of people give license when they think, oh, it's already being done, so what am I going to do? And a lot of people, you know, at least in some of the focus groups, apart from the surveys, you could see that tension about it's not necessarily consent per say, but what is the safety? Why do you do security? What is the confidentiality? What is the privacy? And those issues have to go hand-in-hand, I would say, with the benefit.
Right? Because some people are like unless you guarantee the safety of it and security and the privacy, I don't know if I really care so much about the benefit. Yves, your thoughts? Because this is an area in which you're you're working all of the time. And. Yeah, thank you so much for that. And in support of what Stacy mentioned as well, based on my conversations with different experts, I think they are aware that a lot of members of the society tend to mistrust the government and some private companies because of the recent examples of data breaches which are quite high profile. And if they don't understand the mechanisms that protect their data and the benefits on sharing their health data, they might not support it.
But I think at the moment it's still unclear who really owns what anyway. And I think for some experts, they believe that patients don't care. They always share very private information on social media anyway. But that's a view that we need to challenge as well, because some comments like some thoughts are not equivalent to health data, right? So some people might share what they eat for lunch, but they're not going to share their, you know, medical conditions or their age. So I think there is that misconception that just because people are freely discussing stuff online on social media, that they're just going to share whatever is in their personal health records. And Lisa, I want to come to you for a moment because you deal with pediatrics, with neonates, newly born bubs right through.
And they don't have a lot of say in how their data are used as far as I can tell. How do we as a community seek a social license in that space to ensure that we're actually using data as as people would want? Wow. That's a very tricky for me to address. But I do agree.
Part of the the baby's data comes from the mother. Right. So in some way, the baby must have some right to the data from the mother as well. Which is it? Which is an interesting thing that's come up with an ethics committee that I've dealt with in the past, that if we're dealing with pediatric outcomes, we do need to know something about where the baby has come from in the circumstances in which they were delivered.
But I think this is more a question for the Ethicist! Well done. Great. So who wants to take that? Because it really is incredibly important as we think about social license, we can talk about people who can gift their data to the greater good. But what about those who don't
have a voice? I'll give Yves a chance as well. So generally for people younger than a certain point in adolescence and depending on what kind of decision you're talking about, that point in adolescence can shift choices about all kinds of things, including what happens to your day to rest with the Guardian, who is usually the parent. So generally it's left to the adult to make the decision about what happens with data. Then at a point in adolescence, depending on what decisions are being made, at least part of the control begins to go to the person themselves.
So and it really depends on the the kind of data that are being shared and the kind of situation that you're in. So, for example, if the purpose is clinical care, then the young person might have control of their information a little earlier than if, for example, the purpose was for research. So things can be different depending on the situation. But often I think this is partly about individuals and it's really important that individuals have control over their data and have an ability to have a say about how the data are used. It's also about the community.
You know, all all of this really, we can call on big ethical concepts like the importance of confidentiality, the importance of respect for autonomy, so that people have the ability to make things go the way they want them to go in their life. And that and they're very important concepts and very widely shared. But really in terms of the way that we practice, a lot of it comes down also to a public conversation and what people are willing to sign up to as the social norms and they don't drop out of nowhere.
You know, they're actually part of a form of engagement really with the community. And as we saw in the community juries, you know, when people understand what's going on, their position will shift. You know, they'll make a different kind of judgment about what's the reasonable thing to do. So I think it's helpful to think of all of these things as a conversation, as needing a conversation, and us needing to engage communities and bring them into these considerations.
But Yves, what about you? You've you've done a lot of medical ethics training over the years, what do you think about young people and their data? I totally agree it does depend on the age. So the younger the patient or the health consumer is usually it is the guardian who has the control over what to do with the data. But at the moment there are also conceptual and philosophical conversations about what data are we talking about? Because there is a difference between personal data and sensitive data. I won't get into the definition, but so those kinds of classification of sensitivity, that will also impact on whether the responsibility is on the patient or the guardian. But just letting you know that this is an ongoing conversation. It's not just about social license and people agreeing about sharing their data, but what happens once they share that data? Where does it go to this? It go to a private company? Does it go to the government? Will it be commercialised or monetised, or will it just be used for research? So I think these are massive conversations that you can't really explain quickly.
In an ideal scenario, if you're encountering a patient for the first time and you're collecting information, that's usually at least in the clinical context, that's where you explain where the data might go. But not everyone has the, you know, luxury of time to explain. Here I am collecting raw data now and this is where it might go and this might be the secondary use. It's not just for your clinician, but eventually your data might be used for other types of research and then expanding the benefits. So these are really giant conversations that can't be encapsulated in a very short clinical encounter, unfortunately.
So we've got a couple of questions from from people who are watching today. And I really do like the first of these, 'How do we stop artificial intelligence, exaggerating pre-existing biases in data collection?' There's a good, solid ethical question for you. Well before the ethicist says something. Well, it impacts a lot and Yves and I are working on how do we actually try to get the word algorithmic bias to be thrown out of the vocabulary.
Right. Because, A, the algorithm itself is not biased. B, it is the generative process of the data that could have the social or racial or whatever bias in it, right? So one thing is how do we create collection methods that can do away with what we know are usually biased variables, variable settings.
Second is how do we train our data science tools in a way that we could again do away with some of these and still get the correct signal? Right? Because so far and that's the part where, as I said in the beginning, I don't really think we're at the point of intelligence. We are at the point of pattern recognition, so all the tools we have right now, the faster pattern recognition, and if the data is loaded with a signal that it has a social bias. The pattern is going to be recognised. So that's about all we
need to work out. The generation of data, the process that generates the data, the process that collects it, and then the process that looks at the patterns to see can we do away with some of these things that are way to be seen telling us something that's wrong? I'm so glad, Alberto, that we need to stop calling it artificial intelligence. It's such a shame that that name really caught on, isn't it? Yeah, and it is actually very misleading.
But I'm going to throw straight to Yves because here's a lot of work on both socio legal and data science approaches that mitigate bias, so over to you Yves. So thank you so much, Stacy and Alberto. And one of the issues that I've discussed with the experts say I've spoken to was the problem of algorithmic bias. They mentioned data science mechanisms, so the things or approaches that you can do to de-bias, for example, an existing model or a model that is under development that I think is more of Alberto's expertise. But when people talk about social legal approaches, these are approaches that you can do outside data science, because as we know, when you talk about bias, biases in outputs of models, it's really based on the data that is already biased. And the bias data is really based on the healthcare system.
So whether it's Australia, United States or United Kingdom, we know that the healthcare system has a lot of inequities, whether it's under servicing already marginalised groups or over servicing some marginalised groups. So there is already data asymmetry that exist in the healthcare system. The other issue is in terms of what consists of experts that exist in data science community, but also in areas of decision making, whether it's in policy making or regulation. A lot of efforts coming from, for example, black in AI or womenin AI have criticized these bodies as being exclusively a certain type of person and often the certain type of person are not aware of the impact of technologies on marginalised groups or are not aware of the existing injustices that contribute to the biases. And they are calling for more diversity in the workplace across the board, in any kind of workplace that is involved in the development, research, deployment or regulation of artificial intelligence in healthcare. The other question we have here, and I'm going to paraphrase a little, but at the moment a clinical diagnosis is almost a black box from where the patient sits.
You know, how do people arrive at that diagnosis? Is there an opportunity and indeed a right for patients through AI, artificial intelligence assisted diagnosis, to look into the black box, to actually see some of the workings that are helping clinicians to to make those diagnoses? You you know, this happens if no one goes for it, I just open my mouth. I may be a little bit devil's advocate here, the black box has existed before AI, but most of the time on a 5 to 10 minute meeting with your GP, there's so much and I think you kind of alluded a little bit to this in another sense Yves, you don't have enough time to go through the full discussion of, well, basically all of this is that I can put your diagnosis and I can tell you it's definitely not this because of that. I mean there would have to be a half hour appointment, right? So in that sense, it still kind of exists. Now, I'm absolutely saying it ought to be that everybody has the right to know what's going in, even if it's, again, just with your GP, whether AI-aided or not, I the potential problem we may encounter is different AI or machine learning tools have different levels of explainability of interpretability, so even if there's a desire to, it might be really hard, just as it may be really hard for a GP to actually explain the overall interpretation when you have a pleural effusion or something like that.
Great. I think that's a wonderfully fulsome answer. We've touched a couple of times in the conversation so far on the quality of the data. What goes in dictates in many ways what comes out at the other end. Are they gaps in the way that health data are collected? And if so, how should we address these as we rely more and more heavily on artificial intelligence moving forward in health? Maybe I'll tackle this one first. I guess in terms of gaps in data, I've got a couple of things to mention about that.
We've seen a massive move in health services towards electronic management, electronic medical records, so the collection of data electronically. If health services aren't already doing that, they're certainly aiming towards that. And I think one of the difficulties as a researcher who sits outside the health system isn't knowing what data is being collected. And I sort of alluded to this in the beginning as well when I mentioned that the collection systems that we have are based on what the health service needs, not necessarily what we might want as researchers or even what the patient might want. So getting a seat at that table to decide what data is going to be collected I found, is extremely difficult.
So if there's a solution to this, I'd like someone to tell me. I haven't figured out how to do it yet. And the other thing that I wanted to touch on in terms of gaps in data collection is that I don't think, at least in my view so far we've done very well at all or got very far in collecting patient reported outcomes. I think if we are intending to have patient centered care, then we need to know and have patient reported outcomes. And if they were part of the system like the the electronic medical record, that kind of information might be collected more frequently, more systematically using some of the systematic tools that we have for patient reported outcomes that would benefit patients because the health services could then potentially use that to improve their care in areas where it's potentially not as good and they can do it evaluation so that just two little snippets of my opinion on where this could go. Fantastic. Lisa.
I mean, patient reported measures, including outcomes and experience, are critical and health systems around the world are starting at last to invest in that space and to respond to the feedback that people are providing. And I think it's that latter bit that will not only improve the quality of those data, but also encourage people to engage in that process. And I couldn't agree more, David, because I think it is about you don't just collect data for the just collection purpose itself.
You have to want to do something with it. You have to be able to do something with it. And that really, I think is the most important part.
Categorically, yes. Stacy, thoughts on this? I'm the first person to be on mute. In fact, do I get a prize? So I just wanted to build on what Lisa said about data and collecting data for a purpose. So I think that needs to pull through to artificial intelligence as well, right? Because it's really easy for artificial intelligence to be built because there are data that are easy to build it on. And because it's an easy application to build with data and we've seen quite a lot of AI developed for that reason I think because data are readily available in that space and developers want to develop AI, that's their job. You know, it's exciting to develop a new app.
It's exciting to think that you might be doing some good by focusing in the health space if you've been working in other spaces before, you know, so it actually often is very altruistically motivated, I think. But just like Lisa said, the data have to be collected for a purpose. I think that AI has to be developed for a purpose, and that purpose needs to be driven by clinicians noticing gaps in health systems, patients saying this isn't working for us, communities saying this is a really important goal for our community.
So it needs to serve that purpose needs to be pulled all the way through I think. And they think some of the frustrations of overseas developers trying to get into Australia. They mentioned that there is lack of integration of data amongst states, not even within the same state, but different in a hospital or health service institution. There is lack of integration and it's sort of it is a roadblock.
It is an obstacle to really take advantage of the potential of health data if every institution has a different form or is not connected. It's a frustration for researchers, but it's also frustration for patients because they feel that if they move to another institution or to another state, they have to say the same thing, when they were promised that once we have digital copies or electronic medical records, that will lessen this burden. But that hasn't really happened yet. So there is frustration for different stakeholders.
The other issue that we are trying to look into, and this is something that Alberto mentioned because a lot of the data sets are collected for purposes of clinical service, there might be some missing information. So we mentioned about bias and one way that people have suggested to combat bias is that we need to increase diversity of datasets, right? We make sure that marginalised groups are represented in the datasets. But the question remains what do we mean by diversity? How do we represent social groups or marginalised groups when that information is not yet collected? So think about the information about race, these are sensitive information, and at the moment, even about gender and sexual identity, these are not collected. So we are trying to develop a project where we examine should we or should we not collect sensitive information to improve the diversity of datasets, hoping that that response can also minimise bias. So there is that ongoing conversation, not only what we're collecting, but what we are not collecting from our citizens, from our from members of the community.
I think it lands back to what Lisa and Stacy were saying, the purpose. So just as we need to get purpose for the data we're getting, we should have a reason for not getting certain data rather than then just blindly ignoring it because people are going 'Oh, we can always link, right? But linkages have already, there's a huge area and linkage error and not, as you were mentioning, Yves, within the state you may not be able to link within different health places. Think about judiciary, education, right? If you really want to be thinking of holistic help on on what you're going to do for your healthcare, that's the other part where we're falling apart.
And as we think about that, you know, I'm reminded that there are some European countries where it is illegal to collect race. That's right. At one level, you say fantastic. At another level you say, how do you build artificial intelligence where you can guarantee that that algorithm is going to meet the needs of the entire community and know with it leads to some interesting challenges in areas with which I've been associated in cancer surveillance, in screening, participation and outcomes. You know, in Australia we do not collect any data on someone's cultural or linguistic background with the cervical screening program. And as you've pointed out, Alberto, linkages is not going to to fill that gap perfectly. So
it has some very big implications for people. Another question from one of the people online, and again, to paraphrase, we've talked about artificial intelligence, the need for good data. This question relates even further upstream.
'What about the the investment in the hardware and the actual process of collection? How do we get health systems and indeed other systems, as you've just alluded to, justice, education, community services to invest in in sufficiently robust systems for collecting it in the first place?' Alberto, no one's talking, which means it's absolutely yours. No, I'm trying to be good. Come on.
This is good.. That question in itself had a lot of pieces, right? And so I'm really right now thinking about. I know where to start.
Any help is welcome because...I'm a little blacked out right now. Could you just repeat that? How do we get the the investment in the hardware, the processes, all of those things.
I'll start off if you like...I think there needs to be a value proposition again, that that the end product is so valuable to to health, to patients, the community and to health systems that it becomes a no brainer almost. But the part where I think there's a lot more public than what the wording of the question says is that it's not just an investment on making the tool easier to implement, you know, getting a better stethoscope or better imaging. It is we are in an economy where you need to take away from one source to put in the other source guide, which then it's thinking what is the value that it's really going to bring? Because maybe we go back to the hubris of five years ago where, okay, it's going to give me no error and it's going to give me the perfect diagnosis and the perfect treatment. Okay. If you're going there, sure.
Mobile where you're funding in together, getting better hardware. Just just making a farm for your predictions and for your treatment ideas. Right. But that's the problem, right? At which point are we going to saturate? And again, if we're still depending on that data. And so I guess I'd rather than hardware per say, we need to invest in intelligent data collection, quality, safety and transmission processes.
And kind of integrated and organized systems that that don't overlap, that don't conflict with one another. There was some great work done in the U.S. about ten years ago by Wachter on the effect of digitization on doctors and the way that digitization actually came between doctors and their patients, because it creates what we always talk about autonomous systems. We talk about AI is making
our lives easier, you know, it's kind of like The Jetsons. We're going to be able to delegate all the bits we don't like to the AI, and then we'll just get to kick back and do the fun stuff, whether it's in a professional context or in our personal lives. But actually, the research shows, the socio technical research shows that that really what it tends to do is just delegate different kinds of work to the humans. And that's the work that it takes for the humans to create the inputs that the AI system needs to do what it does. So there's a tendency
actually just to move the work around or create different kinds of work. So I think in thinking about investment, I I don't know, I think you're right, David. It just has to be a really good business proposition to get the investment. But in in strategising that investment there also really has to be an eye to that kind of streamlining that can make it so that the humans aren't just there to serve the system, but the system is actually serving the humans. The kind of system is really important.
I love that phrase that the system is there to serve the humans and we mustn't lose sight of that as we struggle with a world that is going to evolve very quickly, not as quickly as we perhaps thought five years ago, but it will still evolve quickly. Which really leads us to the the ultimate question for the afternoon, which is how can we work with the community, with policymakers, professionals, healthcare workers, researchers to actually realise the potential of big data turned into artificial intelligence? How are we going to, as a community, take those important steps forward if we're going to, to really see the benefits that we hope for? I can start if you like, and then I'll pass to the others because I've done a lot of talking. So we're running a community jury really soon. This is in the AI domain.
We're asking a randomly selected group of Australians. We're going to engage with them all over the country. We're going to give them information about artificial intelligence and how it can be used to detect or diagnose diseases. And we're going to ask them, under what conditions should we use AI for disease detection and diagnosis in Australia? After they've learned they're going to come together in Sydney, they're all going to fly from all over the country and they're going to spend a long weekend together deliberating on what should happen. And to my mind, that's the kind of engagement that we need, that we really actually need to bring the community into this conversation to help to make recommendations to guide policy making.
And in fact, we have very generously we have support from the Royal Australian College of Radiologists, the Royal Australian New Zealand College of Radiologists . Because we're still training them. That's right. And as we are, it turns out there are still radiologists and they probably always will be and they're really keen to support and they want to hear what Australians have to say. We've got a number of
R-Techs which are big groups that connect the health system to the research system in Australia, funded by the National Health and Medical Research Council. We have three of those organisations from all over the country coming and those policymaking bodies. They want to know what Australians have to say about these questions. So for me that's the way forward, that kind of partnership.
So just building on that because obviously, you know, the real patient point of view on integration is crucial. But the other thing that I think we still live in a society where there's providers and customers in a way, and we need to move away from that. You know, we don't need to think that policymakers are going to be the customers of the product that researchers and data scientists have to produce to give to them. We need to be thinking of just as we integrate patients to get their ideas and the population.
We need to be working really, really together from the get go off is this research that's also going to have already a vision of impact on policy and then have policymakers in the group right, rather than saying, okay, well now we created this has know suppliers, we created the data, we created the output, we're going to the policymakers. Here you go, it's in your court to play with it and see what we can do. Right. And that is one of those
things that we'll start getting the circle to actually feedback onto itself and I think make a better a better sense of developing the AI with a purpose and with potential policy implications going forward. Yeah, I guess my my favorite way of working is to work with clinicians or practitioners, health practitioners. And so I really enjoy that because that brings questions to me that are definitely relevant to their situations in their day to day practice and where I feel like the translational impact is most powerful because they have the potential to change those systems from the inside. So that sort of flows along the way with what we're talking about here that is just for me is the most rewarding as a researcher, and I just thoroughly enjoy the challenge of getting to know their fields and how they operate and what the issues are so that I can do my little piece towards making that bigger impact. Yves Thank you so much.
I think Stacy, Lisa and Alberto have said a lot on strategies and I think co-design is very important, but also just making sure that our research doesn't remain just within the university. I think University of Wollongong is good at really sharing what we are finding out into the world and I think the public, we should empower the public to understand the issues and not just as part of our projects but just the public in general. Share your research.
What are your findings? How is it impacting or how would it potentially impact the lives of Australians? I think we have to be more active in sharing our research and not just being siloed in our office. And I think that that's what really inspires me as well, is if there's something that we find in our studies that we can share that with the public. We're not just working with the public, but we're also making sure that they have the information.
They can also access the information that we gather through our empirical studies. It's imperative that this is a whole of community conversation and that we're sharing openly and fully what we find and and how we have found it. It's a bit like, you know, Year 8 maths.
You might get the right answer, but you've got to show the working as well. And I think that's where we have not done well as as researchers in communicating and and working alongside the community. We've only got a couple of minutes left, so it's up to me to ask the fun and very obvious question and to come full circle. Will GPT have any impact on health in Australia? I'm enjoying it so far.
Well, that's got to be worth something. A little anecdote, as many of you here on the panel know, maybe the audience don't we have a new MPH that we're currently starting now and we have a big data specialisation within the MPH, so check it out. I'm just doing a quiet plug there. But with the new MPH, I'm developing a new subject and so there's all of this ChatGPT.
So I'm thinking, all right, what is this thing? So I signed up and I thought, I know I have to create a tutorial, so I'm going to ask ChatGPT what is selection bias? And ChatGPT comes back with this nice stream on selection bias, which is exactly what some of the panelists were saying before. It repeats essentially regurgitates what's out there on the internet. But some of it was actually wrong and I thought, admittedly my students are doing this, so don't listen to ChatGPT, "you're wrong. This is incorrect". And
so it comes back to me in apologise. Yes, I was wrong. This is the correct answer. So here is going to be a lesson for the students in my class. I hope there's none in the audience about the use of ChatGPT. And so I'm trying to get wiser in what it does, how it works, and how I can use it and teach people how to use it. So that's one little
anecdote. That's beautiful. I'm totally with you. If we think about the first time any search engine came out or even nowadays. Three, five different people are looking for the same thing. Three of them get to it first, Right? So knowing what to ask, whether you have a faster because that's really what ChatGPT is, it's a way, way, way, way faster collation.
Right? It's not intelligent yet because the semantics are wrong. Then our exercise is going to be on being intelligent when we ask people to be intelligent, about to ask CPT to produce. Yeah.
It's amazing stuff. Yeah, I think maybe this will improve over time. I'm hoping it does, because I know that my use of it will drop away if I don't see it doing things that are correct. Yep. So that's just my personal view. And I've had a couple of a look at a couple of others as well.
Some of them I haven't been particularly impressed by, but yeah, let's see how. And I think there is an ongoing conversation how different it is from, say, Google search. So we'll find out their ongoing research about how a patient can use it or how a clinician or a public health expert can use it. And there is ongoing
research on that. I look forward to to seeing that. Please join me in thanking Lisa, Alberto, Stacy and Yves for joining us today and giving us a great insight into the brilliant research from across the University of Wollongong. Thank you also to you, our audience, we hope you enjoyed the discussion.
The event was recorded so everyone who registered will receive a link to the recording through email. I'd finally like to thank Jill McGarn and her team for bringing this together and the excellent work they're doing behind the scenes to bring the Luminaries program together. Look forward to seeing you in two weeks time.
Have a great evening. Thank you so much.
2023-03-19 07:16