Learn Data Science in Just 180 Days - AL & Machine Learning | Prompt Engineer | OpenAI & ChatGPT
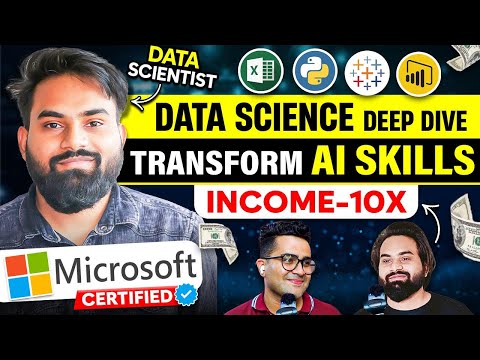
How can prompt engineering help software engineer to increase their productivity? First tell us, what is it? If we've an old blur image but we can convert it into high resolution with the help of AI, so artificial intelligence will mimic it that how can it be seen in high resolution and it'll make it. There're lot of AI tools related to it, nowadays. If I talk about Chat GPT, then they used 570 Giga Bytes of data and 170 billion parameters had been used in it. Which tools & technologies are required to build a model like Chat GPT? Suppose you need to make a similar model for your company that model should only tell you about the company. Then which tools & technologies are required. So, if we talk specially about - I had seen an app that tells age it detects age by looking at the object.
It'll tell you by looking at the object that - if this is a human or an animal so, what is this called - is this AI or has it been build by the combination of ML and AI? So, as I was talking to you off-camera - you told me that, you had taken interviews. And when freshers come to you, then its a very common mistake like even in their resume and the way in which they answer. So, can you describe any scenario - how can they avoid making such mistakes? So, What is Artificial Intelligence? What is Machine Learning? What is Prompt engineering - that is in trend in the market these days? What does a prompt engineer do? How to make a large language model? How was Chat GPT created? What is GPT 3? What is GPT 4? Today, we talked about everything - we tried to understood it through examples that what AI is what Machine Learning is? What is prompt engineering? How to make a language model? We'll talk about that in this podcast - you'll get to learn lot of things - today I've such a guest with me who has 3 years of experience in Data Science and now - he's an AI ML consultant. And their entire experience, project experience - we'll talk about all that in this podcast, so if you like this podcast then do like it, share it, comment down. Sit comfortably, you'll get to learn lot of things. You'll get to learn new things. The condition is that - watch the entire video - don't skip it.
And I've given the chapters in the description that you can follow. Do like this video - Like aim is 3000 - accomplish it for more such interesting podcasts. Let's talk with Prins & get to know AI, ML, Prompt engineer even more properly. Okay! So, Prins - welcome to our youtube channel. Today, I'll get to know from you about machine learning, about AI. And about prompt engineering as well - its a trend these days in youtube - what are prompt engineers - what is Prompt engineering? I'll understand about that & know about your 3 years of experience in AI & Machine learning in data science.
So, I won't take much time - over to you - You can start first with your introduction - your academic background where do you belong to. Please - over to you. Thank you, Ajay! So, I'll start with my introduction. So, my name is Prins Kumar. And I've complet my B. Tech in Electronics and Communication
and then I started my job in Reliance JIO but I wasn't satisfed there, so I started exploring new technologies. That's where my interest evolves in Machine learning & data science. So, then I did few trainings & then joined Prolifics, so right now, I'm working here as an AI engineer. Okay! So, where do you belong to, Prins? I basically belong to UP, Noida. Okay! So, as you told that you're an AI consultant, work around ML so, I want to know the basic because we identify everything as AI.
This is AI tool. If we look at a reel on Instagram - this is made from AI - first I want to understand What is AI? We'll understand with a basic example & what is the difference between Machine Learning & AI? If I talk about AI, then if we design any rule based program or understand something from data then that is basically AI. For example, we give human intelligence to a machine. So that he can decide few things by himself - like decision making, recognize the pattern or to identify the sentiments - in this way. So, this is an artificial intelligence, therefore its not specifically dependent on data
we can make some set of rules or we can even learn from data but if we talk about machine learning then Machine learning is a part of AI. So, the place where data is involved very much then we try to learn from data and we design algorithm in it, basically & build statistical models that tries to identify the data. Find patterns in it or make predictions. Machine learning is more like based on predictive analysis but AI means if we make some set of rules, that can mimic human intelligence then that is basically Artificial Intelligence.
As now, there're many tools - if you go for reels then you might see that someone puts a text there - a video was generated. So, that happens through AI - but if we talk about the example of machine learning then as we've to predict the price of real estates - or classify the cancer so, these are example of Machine learning. And Okay! Nowadays - if we've old blur images, but with the help of AI we can convert it into high resolution.
So, it'll mimic artificial intelligence that - how can it look in the resolution and it'll make it for you. Now, there're lot of tools related to AI. There're lot of different technologies as well. *Okay*
I want to understand some basics from you - so when a system is created, as you gave the example now so, which technology is used - if I need to train a machine if I need to train a model - which technology is used there, Prins? Firstly, if we're talking about AI tool then we need to understand that what problem are we trying to solve Okay! If data is involved in that architecture or model, then we need data. Nowadays, Chat GPT is highly involved so, if I talk about that - since its such a big model & its based on natural language processing means the way we talk - as now we're talking so, we need huge amount of data so that it can mimic us. So, If I talk about Chat GPT then, they used 570 Giga Bytes of data and 17.5 billion parameters were used. So, in this way data is very important. Then - competition that - we would need architecture we would need resources, so if we plan such huge model then for that we would need GPU.
Because in Chat GPT - deep learning was used. So, for that you need GPU, then the model can use architect then the place we want to deploy. Where do we need to deploy API - on cloud or on any infrastructure. So, we need all these setup for any AI tool or machine learning tool. Okay! So, I saw an app - that tells the app, it detects the age by looking at the object.
By looking at the object it'll tell you - if its a human or an animal. So, is this AI or is it build by the combination of ML & AI? This is a combination. As you told about telling the age - then firstly there's a part of deep learning in which they detect the object. So to tell the age, first you need to detect the object that - if its a human or as you told if its a dog or any other thing. Then first, object detection is used. Then, we're classifying it again - after that as the object is detected as a man.
There's a man and after that - because already lots of images ae on trend in data that as there's a person - how will he look in the age of 10-15 How will he look at the age of 20-40, so it tries to match it that way. That yes, it comes in this range. So, that won't tell you the exact age but somewhat close. Perfect! I understood your point, so he's trying to tell that - if there's any machine or the system you're trying to tell - it is exposed to different types if data and it is taught. And behind the scene, there're some algorithms that are being used to achieve in making the system the model that has been designed. Now, in the case of Chat GPT - it was trained at the level of GPT 3 - like he gave the example. So, things work in this way.
Okay! So, now Prins tell me - as people are talking a lot about prompt engineering. There's a different designation - prompt engineers. So, how can prompt engineering help software engineer to increase their productivity? First tell us, what is prompt engineering? Prompt engineering is basically instructions - they're set of information that we can say.
Now, if I take the example of Chat GPT, then is a text-to-text based model So, we input an information in the form of text. Then, it gives an output in the form of text again. So, here - the information & text that we pass on to Chat GPT is basically prompt.
We call it prompt in terms of engineering. So, first is - one shot interference in which we asked - basically the give an email, suppose and we asked it to tell us the sentiment of email. Is it positive or negative or neutral? So, it'll tell us. Now if the result aren't accurate - you felt that NO, its giving negative but when you're reading it then the sentiments are positive. So, then we can give the example that - classify this sentiment - this is an example, this is the mail and its content and its sentiment was positive. Then, if we give it an example - its called one shot interference.
And if we're doing without example, then its called zero-shot interference. So, if the results are accurate after giving the example - or after giving more examples our result are accurate, then this is prompt engineering. Basically, to make our model accurate, bringing results according to itself is a technique of prompt engineering. The information we're passing would give us accurate results. And basically, its should be able to tell what we want. As we explain things within us, then until that information is cleared basically, until then we won't understand.
Because Chat GPT is mimicking human intelligence. So, that is able to understand your context or not, then that is why prompt is very necessary. Right! So, asking or prompting in such a way that - the model or system is able to give you accurate answer. I won't say that it should've 100% accuracy but it is achieving 98% or 95 % Yes! I actually forgot to add, as you had asked - how does it help software engineers As I know Java & ultimately that project was moved to another language supposedly. But, if we learn that then it may take lot of time, as going from Java to scala it is somewhat similar but if we'll learn it and then implement, then it may take time. But with the help of prompt engineering
we can tell it to translate this code to scala from Java. So, that gives us results very quickly. But we already understand the code of Java that what its functionality is. So it'll give us the same functionality in Scala. So, it can help in many other ways, like if there's logic in your mind but you aren't able to implement it. So, you can simply give instructions to it that what are you trying to do.
And it'll give you the code. Or if you don't have time & you have to write a long code but you know that these things will be involved so, you can simply give instructions and generate the code. Okay! So, software engineers can save lot of time through Chat GPT. Which are the tools & technologies required to build a model like Chat GPT? Suppose, you need to make a similar model for your company so that the model only tell about the company. So, which are the tools & technologies required? If I talk about Chat GPT, then large data has been used in it. Because If I talk about - for example, those 175 billion parameters as I told earlier, that it was trained on.
If we make our own model which is specifically organization based. So, first we would need the data of our organization - the problem that we want to solve if I take the example, Bloom bird - they've recently made a model - Bloom GPT that basically gives results related only to finance. Like, if we want to extract finance related information from an article then what they did was - they used 50% general data and 50% data of finance.
If an organization is specifically making for themselves, then it can use 50% general data 50% of its personal data - then they would need infrastructure. Like GPU - now, to train a model of 1 billion parameter, you would need GPU size of 80 GB. So, how bigger data are you training it on and how many parameters are you using, your GPU size would be according to that. Then, the architecture of large language models - earlier what happened was, basically before large language models, we used NLP our human nature to understand the languages. Then, a port of NLP - LSTM was used. Then, instead of that we used attention mechanism because often If I try to translate a sentence, then actually it translates word by word, so often when we merge them, then the translated word doesn't make any sense.
So, at that time attention mechanism arrived - whatever we're translating, is it making any sense or not then in 2017 or 18, I don't exactly know the time - an article was published on transformers. So, they had used this attention mechanism and decoder - encoder for the first time that first, it encodes your inpur text, then decode it. So, the models like - Chat GPT transformer architecture is used in them. This model architecture is very important. Then, its evaluation that - how accurate is the model that you've trained in which way - so, you need these evaluation techniques - we need infrastructure to deploy it. That, you need to deploy it in cloud or there're other platforms, where all these large language models can be deployed.
Okay! Which language should I know, technology wise? Technology wise - if you're in AI, then basically Python is currently versatile in AI because its easy to learn & you understand it very quickly. Then, if you know 'R' then it'll work fine. After that - Python, R and if I talk about tools then you should know Machine learning, deep learning is very important. And now generative AI is very popular as well, so deep learning will evolve more. Like, in LLM - neural networks are only used. So, right now you need these tools.
OK! Now, you're working in a company around AI, Machine learning, then would you like to give such an example from your project experience, that you might've recently implemented with which audience can relate & understand the importance of AI & ML. There's actually an interesting project, which is going on in the company - which is around generative AI. So, what happens is - company get lots of emails and responses some are specific or related to project. So, we're currently trying to prepare a model. First, if we got an email from a client then we need to understand what the requirements are and on that basis our model generates auto-response that yes, you have this requirement - so our company has worked in this field since many years, with this experience and we can provide these services to you. So, for that - a particular person doesn't have to sit there & give response AI will give the response itself and it'll maintain that whatever the structure / subject is shouldn't be changed of that topic. So, right now work is going up on it. They'll launch it very quickly.
So, now as we were talking off camera - you were telling that you had take interviews. When freshers come to you, then there's a common mistake even in their resume & the way in which they answer. So, can you describe any scenario - how can they avoid making such mistakes? So, the first thing that I had observed was that - lack of preparation. That now, AI & machine learning are very fascinating words.
So, everyone wants to AI & ML. Even if you choose, its important for you to have interest. You can't choose only by the reason that - it has good salary or the name is very fascinating. As I asked most of the people, that if you've chosen - what were the reasons - if the salary is good in it. And what is the second reason? I don't have a 2nd reason. Only the salary is good.
Okay! Due to this - whenever we ask questions related to them, then they don't know because their fascination is salary and not AI or ML. So, if you want to come to this field then, first you need to understand it and prepare for it if you want to come to this field. So, preparation is very necessary & practice. Hands-on like, projects of machine learning so hands-on are very important in it. As I'll tell my example, when I was doing the job in Reliance JIO I decided to leave the job as I wasn't satisfied. So, then I started Python. Firstly, curriculum is very important.
You don't have curriculum if you don't have path, the same thing was happening with me that I was learning things in bits and pieces because I wanted to know what data science was it included Machine learning, Sequel, R, Python - so, I didn't know that you can choose among Python and R. I was learning both parallelly. And I was learning even though I didn't understand it - whereas if you understood python, then you can continue it. Like, in R there're regression techniques - in Machine Learning or classification so, what I was doing is - I picked any model & worked on it - which I didn't even understand. Because it took some things from here & there, so there needs to be a path for it. Okay! Prins you're telling us very nicely - your experience and I'm able to relate a lot, you might've be able to as well, guys.
You might be able to understand all of it about AI & ML - prompt engineering - large language models Okay! So, tell me - as you talked about the resources, your learnings - so where did your learning skills related to machine learning, AI, Data science - which resources did you follow when you were learning this? Initially, I had simply started. Actually I didn't want to pay, basically and learn everything in free, so I tried a lot I tried youtube, then read articles online, content is available but they aren't structured. There should be a particular path which wasn't available. So, you learn things in bits and pieces but you can't relate to it. And when I was learning, my friends had - I don't know if you've heard or not there's an organization called OdinSchool - in Hyderabad. So, he suggested me to visit it once.
So, I enrolled there. I liked one thing there that - the curriculum was well designed. Like - I haven't checked out for a while but there's a bootcamp of 6 months. In which they teach all the technologies related to data science.
Like, Python, Sequel - they'd even used statistics which is very important - if you go for machine learning if you're making statistical models, then to understand the data. So statistics are very important. That was there as well. There was Machine learning & later - that time it was a bootcamp of 6 months but often Big data is also necessary. So, they had also added the training of PySpark. As I was trying from before, that I should learn simply in this way, what happens is - a curriculum is designed but there we also had placement support. And I was thinking that - because when you learn, you can - but if you visit LinkedIn or if you apply anywhere then you need to struggle a lot for jobs. You don't receive interview calls, so if there's any organization - if anyone feels that its good.
It is providing good training, then enroll there. Give proper time - 6 months or 8 months - whatever you think. And come into ML after a good preparation. Before that, preparation is very important. Okay! You told about your experience very nicely.
I would like to know - in few words - a question popped up in my mind if you need to make a language - a model - a system then what will be your steps? Prins ! For a company - if I talk about Machine learning, then I'm currently working in MLOps firstly, its necessary for me to gather the data. So, there can be various resources of data - like, a flat file, CSP or anything or RDMs can be there, or streaming data - we need to learn to consume it. Then, its pre-processing is very necessary because often it is not cleaned. Pre-processing it, then feature engineering is very important in Machine learning.
That which features are actually contributing in model buildings. After feature engineering comes - model training. So, model training - these days we can't be dependent in specific algorithm. So, we can train the model on different set of languages from where we're getting huge accuracy after that evaluating it - that how accurate is this model. After its evaluation, comes deployment. So, deploying it. Now, if we talk about infrastructure in MLOps, then
after that - we've model registry. The model should be registered first, after deploying. After that, what are the changes in it - so model tracking is also necessary that - whether can changes are taking place in the model or not, or versioning - these are very important. So, this is a proper life cycle of machine learning.
So, what is your final advice to our aspiring data science, who want to learn so, what will be your advice to them that - they should keep these things in mind & don't make these mistakes. Firstly advice, would be - don't join if you only feel fascinating or salary is good whether you've interest in it or not - is very important. firstly, interest - then you need particular path which is very important. You can decide that - where do you get it from. After that - hands on - all the hands-on that you can do, related to project as we do data wrangling - at the time of data pre-processing so, the ore you do it - you'll be that fluent. So, preparation is very necessary.
Okay! So, I hope guys - you got the opportunity to know more about AI, ML, prompt engineering, Chat GPT, we tried to understand prompt engineering properly. And how do we make a language model - how to train it by using different algorithm we got to know it from Prins. So, I believe you might've learned lot of things - if you liked this podcast, then like it, share it, comment. Like aim is Rs. 3000 - accomplish it for more such interesting videos I'll meet you real soon in other interesting podcast. Till then - take care - Thank you so much.
2023-09-09 07:10
Other news


