Digitalize battery development faster, cheaper, better
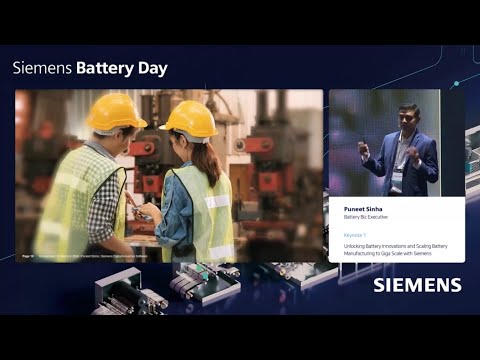
- (speaking in Korean) Korea. This is the only Korean I know, so the rest of the conversation I will have in English. I'm really excited to be here with you to kick off Siemens Battery Day.
The world around us is changing and technology is playing a big role in making it happen. As I look around in this room, it makes me more convinced that this decade is the decade of battery. There is growing demand for battery fueled by transport electrification as well as because of grid energy storage. And it is projected because of that, the production capacity for battery needs to be 14 times by the end of this decade compared to where it started. Additionally, governments around the worlds and their regulations are actively shaping the battery ecosystem. For example, EU has mandated digital battery passport for any battery, which is more than two kilowatt hour by 2027.
So when we look at all of these factors, it is not surprising to see that battery suppliers, machine builders, material developers, automakers, they're investing heavily, more than $300 billion, in batteries. As BJ mentioned, Korea is one of the leaders in battery. You deliver more than 25% of global battery capacity to the world.
However, as you battery suppliers, machine builders, material developers, and auto OEMs look to increase your market share in this highly competitive, increasingly evolving battery market, there are various challenges that you face. I'm just gonna mention few. For mass adoption of electric vehicles, the energy density of battery, that means how much energy you put in one kilogram of battery needs to be pretty much doubled and that requires changes in materials, new innovations for cell and pack. As Korean companies go and set up new plants, not only in Korea but across the world, there will be tremendous new challenge in scaling of those production capacity. That on top of how you reduce your scrap rate, improve your quality, also meet sustainability challenges as well as regulations. When we look at all of these challenges, we need to also keep in mind that battery fundamentally is very different than IC engine.
In battery, you have materials, electronics, electrical, mechanical, software. All of these domains interact very, very strongly and these interactions are either very weak or non-existent in the technologies of past. And because of that, the traditional engineering and manufacturing practices which were actually developed and perfected for IC era are not adequate.
We are seeing that when companies rely on these traditional processes, they encounter lengthy development cycles, very costly processes often leading to over-engineered and overpriced systems. When I go across the world, everywhere I hear that companies are itching to change and bring a new paradigm shift. And that's what we are looking to bring with Siemens, we are looking to change it through what we call digital transformation. What we are focusing on is how battery companies can accelerate innovation. How can they unlock the potential of their large scale manufacturing by combining the digital world, where you can explore new designs, new innovations from material to system level, and connect it seamlessly with the real world, where these innovations needs to be manufactured, needs to be deployed.
And how we do that? We do that with our accelerator portfolio that is based on three key dimensions, digital twin technology, automation solutions, and data and generative AI. How we are delivering this digital transformation is through what we call digital threads. What these are, these digital threads essentially are taking the solution that we have and connecting them, mapping to your business processes. I will not go into details of these digital threads the rest of the day.
We'll give you a chance of listening to our speakers and getting a deeper dive in what these digital threads are, how they're adding value to your processes. What I will focus on is the business value we are bringing to battery ecosystem. And I'm excited to share that Siemens, with this software and automation portfolio, with our accelerator portfolio, has gained exceptional recognition in battery industry. Eight out of top 10 battery suppliers who pretty much account for 85% of battery production capacity today leveraged Siemens solutions. In addition, 19 out of top 20 battery machine builders also use our solutions.
We are not only working with these large enterprises to help them continue to be the leaders and drive this future of battery industry, we are also working with the disruptors. When we look at the new startups, new joint ventures that are coming in the battery world, increasingly all of these companies and many more are using Siemens as their preferred technology partners. Today, what I wanna focus on is what is Siemens vision and our capabilities in delivering digital transformation and why these companies are working with us. Digital transformation is not a button that you push and your processes are digitally transformed.
We look at it as a journey and we look at this journey in five stages. As you go from the left hand side to right hand side, your processes will become more and more digitally mature. It starts with configuration. What that is is all the data that you have about your battery cell, pack, your manufacturing systems, your factories, how you bring that data seamlessly together as a single source of truth. Then come this stage of connection.
What happens there is how you can bring all these different domains that I mentioned, chemistry, mechanical, electrical, electronics together to create a comprehensive digital twin so that you can explore, evaluate the behavior of your product, your production, your operation easily. Then comes the stage of automation and this is where you start to bring together the digital world with the real world, how you bring IT and OT together, how you take those workflows and robotize them, how you look to bring logistics. All of these three stages are very critical and essentially what they're doing is setting the foundation for you to take the digital journey so that you have your data of what you are setting it up, what you're engineering, what you're manufacturing is available. Many times, actually most of the times, when we talk to companies, they think of digital transformation ends at that stage. It is important to get to this third stage, but as I said, you're still just getting the data. The power of digital transformation starts coming after that, and that is we take our customers to what we call generative stage.
So here the data that you're collecting, you can start adding AI and become more predictive, understand why certain things are happening, how you can improve your production process, your engineering process, your deployment process. And then the last stage, what we call adaptive, and that's where you don't wait for a problem to happen, you forecast is something bad gonna happen or how you can correct that. So and taking the feedback loop from what is happening with your previous version of your product and how it needs to improve the next iteration of product and production. What I will do today is I will take you through this journey, not by telling you what Siemens does, but by telling you how some of our customers are taking these journeys with us together.
I'm starting with the first stage, as I said, the stage of configuration. I mentioned earlier, battery is very dynamic environment. You not only need collaboration across different domains, chemistry, mechanical, electrical, electronic, software, you also need strong collaboration across all of these experts, across your suppliers, across your end customers. How you do that? To make it more efficient, what we do is Siemens bring a single source of truth across the extended enterprise in a way that you can easily look at the data while making sure your IP is protected. I'll take you to an example of Northvolt, which is a European battery startup company.
They took this journey with us and they're reaping significant benefits. This company, just in a matter of few years, went from 25 people to 6,000 people. And as you can imagine, as you take this growth, there are different silos that we're building in.
They were having trouble having the right data at the right time, working through the different versions, always worrying about the IP protection. As they take over of our PLM platform, that they were able to bring all of this together with battery specific data models and that allowed them, they allowed their engineers to be more efficient. That is resulting almost 20% increase in their overall engineering process and they're on the track to improve the usage of recycled materials in their cell production by 2030. Single source of truth is getting even more critical because as I said, regulations are coming, battery passport is coming in 2027. In order to meet the requirements of battery passport, companies will need traceability across the whole supply chain, about what material is used, where it came from, what is the carbon footprint, performance analysis of battery crosses whole life cycle. By having the single source of growth of your product and production, you achieve that.
With our portfolio, companies are looking at how they can assess the carbon footprint, how can they collect the data that needs to be fed into battery passport. Moving on, second stage, I wanna talk about which stage of connection. For companies, for you to develop high energy density battery, you need to account for multiple factors. You have to look into various chemistries, how those chemistries impact the cell behaviors, performance and safety. You have to look at the optimal thermal management, what are the right algorithms for battery management system and many, many more aspects.
The standard way of doing things, what companies have been doing in past was very testing heavy approach. You build something, you test something, you learn from it, and do it again. For battery, that's a very long and very time consuming process. In order to significantly shorten this cycle, what we are doing is we are bringing comprehensive digital twin that is connecting all of these domains so that you can front load the exploration.
What are the implications of changing a material on the cell behavior, pack behavior, a safety performance lifecycle? I'll give bring you example of PSA Peugeot, an automaker in Europe. When they were looking at what pack they need to bring for their electric vehicle, these are the problems they were dealing with. It was very tough for them to do the trade offs across these different requirements by adopting our comprehensive digital twin, they could reduce the time it takes to engineer pack by almost 50%.
Comprehensive digital twin is not just about battery cell pack. It also plays a very powerful role when you look at the production facility. I take you to an example of HCM, which is a LMFP cathode active material developer. What they also do is they also develop their own lines. One of the big problem that they were facing is how can they reduce the time and cost it was taking for them to change their lines to and tweak them and redo them and test them for different users.
And especially for them, they have different process. Some of the work cells have cyclical process, some of them are batch process. So it is very, very critical for them that every workstation is designed correctly, otherwise there is a lot of equipment idle time reducing their production efficiency.
What they started doing with us, they leveraged our plant simulation so that they can bring a distribution of their complete line and that they can connect with the MES and SCADA in their factory so they can simulate the output of the line, what are the potential problems, what will be the bottlenecks, energy outputs, and many other things. And this was key for them to bring more than 15% improvement in production efficiency and approximately 5% reduction in the electricity consumption for their full line factory. And they're on their path working with us to more and more automate their production line. One of the big problem I believe, everybody in the battery industry is facing is availability of machines. To my last count, it is taking almost 12 to 18 months of lead time to get the machine that you will need for your large scale manufacturing. Also, when I talk to companies in Korea and across the world, many of them are looking to engineer their own machines.
Yeah, bring new innovations so that they can simplify, consolidate various process in one machine. The typical process, you give a specification to machine builder, machine builder, builder prototype, we test it, install it in the factory to find error. Too long. So to improve that, we come to the stage of automation in our digital transformation journey and with team as we bring integrated workflow from concept to the full commissioning of these machines.
I bring you example of Manz, a German machine builder. This is the issue they were facing. What we started doing with them is they not only bring the digital twin of the machine, they also started doing the PLC code generation and validation against these digital twins so that they don't have to wait for the prototype to be ready to do the controls and validation. And this we call virtual commissioning process, which is a combination of digital twin and automation technology. This is tremendously helpful for them and many other machine builders to reduce the time it takes to build the machine and cost, in their case more than 20% reduction in their machine development cost.
The pinnacle of this stage is bringing IT and OT together. One of the big problems that we see for battery industry is the high scrap rate. And why that remains to be such a big problem? Because 80% of the data that you are generating in your factories is untapped. That is stopping you from leveraging the data to learn what is happening in the production process, how you can learn from it and improve it. Many companies, startups, large enterprise are coming to us to solve that issue with them. I bring you an example of Freyr, a European battery startup company.
They are at a pilot line. They're looking to go to multiple gigafactories. They took this journey with us where they wanted to build a data-driven smart manufacturing facility/ And how they are doing it, they are connecting the OT, all the PLCs and drives and machines with the IT side of the work through MES and SCADA layer back to the PLM. And by bringing this IT/OT convergence, they are able to enforce right manufacturing practices, especially when they have to call the material consumption and controlling the machine parameters. And as they're deploying these even on their pilot factory, they are more convinced than ever that this is the path for them to reduce the production time, to reduce the scrap rate, and they believe they will be able to reduce the scrap rate and have the time that would've taken them otherwise. As I mentioned earlier, all the things as you see that I've talked about is all about setting the foundation, single source of growth, comprehensive digital twin, automation, IT/OT integration.
These are very, very powerful stages, helps you get the right things in place, but are they solving your scrap rate problem? Are they solving your quality problems? There is more we can do and that's where I bring you the stage where I wanna spend more time, the stage of generative. What we are doing at this stage with our customers is once they have the data, the right foundation, we are working with them to bring the power of data analytics, battery specific analytics, and generative AI so they can bring the things like real-time analytics to improve the quality, to reduce the scrap rate. I'll bring you a couple of use cases starting with the use case of this tier one battery supplier. They have IT/OT integration in place, they have the PLM system, but the problem they were struggling is in their production. When they were doing the formation and aging at the end of cell manufacturing, they have to wait 20 days to find out whether they have a bad batch of cell or good batch of cell. And that slows down the process, it's a big bottleneck in their overall throughput.
Working with us, because we are working with the data that they're collecting and they were throwing away, as I said, 80% of the data that is coming out of the machines is not utilized in the factories. We are working with that data, and within few hours, whether we're battery specific analytics, this customer was able to find out whether they have a good cell or a bad cell. What is the result? They don't have to wait for 19 additional days to find, do they have a problem? Is it good? Yes. Does it solve the the end problem? No, because just to find problem quickly is good but not good enough. So then we continue working with them so that not only they find the problem, they can also see why that problem is happening. And this is where the data from which material batch they're using, how different machines parameters are, how those things are controlled, all of data come together to correlate the issue that is driving this problem.
In this specific case, they found out the problem was the slitting blade that was driving this bad batch of cells. And then once they found the problem, they now continue to work with our industrial IOT, the data that is coming from PLCs. We were able to set a predictive maintenance schedule, leveraging machine learning. And with that, they could find out what is the slitting blade replacement schedule needs to be so that they don't have that problem. So as you see, building on the foundation, applying generative AI, machine learning, data intelligence, we are delivering real value. And in this case, this process helped them reduce the scrap rate by more than 10% from their baseline.
And because now they can do the production much faster, they have less stopping, they're projecting then for their large gigafactories, this deployment will save them more than $100 million per year. Another use case I wanna emphasize, when we look at gigafactories, everyone is looking to improve the throughput. One of the reasons why throughputs many times is low is downtime of the machines, and many times is because it is tough for operators to report the problems in the timely manner. To our count, more than 70% of the problems go unreported. So how we solve it, we are bringing the power of generative AI connected with the PLM and automation technology. This is the work that we are doing, partnering with with Microsoft.
And I wanna take you to this journey through a descriptive use case. So in this case, there is a factory. This is a pack assembly. The operator found out that there was some problem in one of the robots. They could speak in the native language in the Teamcenter app. Microsoft OpenAI services translate that from the native language in English, also describe the problem leveraging AI in Teamcenter, which is our PLM backbone.
That problem then immediately can be sent through leveraging the single source of data, the robust data backbone that I talked earlier, to engineers who may not be sitting at the same location. In this case they were sitting in Germany. The engineers who are sitting there, they immediately get to know the problem. And they're not only getting to know the problem leveraging AI, they're also trying to find other things they need to know about that machine. In this case, the problem with the PLC code that they have to go, AI again is helping them write the PLC codes, debug the PLC code. So they find the problem easily and they they find the solution quickly.
Once the new PLC code is created, they put it back in our team center backbone and the solution is immediately sent back. Operator on the other side, it has access, gets the code, installs it in the robotic arm and the problem is solved. So as you see, we are not trying to change the behavior of operator, but trying to bring the power of generative AI connected with PLM and automations that they can do the work effectively without changing their behaviors.
I mentioned about three things so far, digital twin, automation, data and AI. When you look at these three dimensions, they all come together in what we call industrial metaverse. Earlier this year, our CEO Roland Bush, he has announced how Siemens is investing and leading the work in industrial metaverse. Industrial metaverse is essentially a virtual representation where you bring the immersive environment connecting real and digital world. Companies, especially in the battery world where everybody has to do things very fast, companies are looking to leverage industrial metaverse so they can learn from their data, find the mistakes quickly, especially when they have to build and scale those factor at different places.
Many companies are working with us in this area in the battery ecosystem, I bring an example of Freyr. As I mentioned earlier, they are trying to take a journey from a pilot scale to multiple gigafactories. Leveraging industrial metaverse that was founded on digital twin, automation technology, data and AI, they were able to create the full view of their gigafactory that they want to install that helps them collaborate with their suppliers as well as with their end customers so that everybody has a skin in the game, everybody can collaborate, make sure the product they are gonna develop will be logistically fine and most efficient.
They can also leverage the industrial metaverse to interrogate the real world. They can look at the problem and extract the digital information about it and find how can they solve that issue. Essentially for them, they are looking at this as a virtual control room for any factory that they create. So they can look into the energy consumption of their factory, how they can optimize it, how the material is gonna flow in this factory. And this is just one of the example. This is a journey.
There is a lot of use cases. For Siemens, what is critical is that when we look at Metaverse, it is not just a high definition rendering movie. What it is is how we deliver the value to customers so that they can go back in time, find what the problem was and fix it, but also go in future and find what they can do better so that those problems don't happen.
And that is the power of the industrial metaverse that we are looking to bring. And that is something that allows us to take to the next step of our digital transformation journey that we call adaptive. Everything I mentioned so far, especially the previous stage, generative stage, very powerful, but what it is doing, it is still waiting for problem to happen.
And what we are allowing companies to do, you find the problem quickly and you find the solution. Where we want to take the industry is you don't wait for problem to happen. As operation is happening, can you forecast when the problem is going to happen so that the machines and systems can take the self-correcting actions? And this is something that can ensure the battery manufacturing, battery deployment in the field can be scaled up in optimal way. And how we do that, we are looking to do that through the power of executable digital twin. That means taking the digital twin and making it run on the edge devices in the cloud, on the factory floors. I'll describe that through a simple use case.
In this case, we are looking at winding machine. Many times, one of the challenges with winding machines, when you are doing it, the foil can break during production. You have to stop the production. It is very tough to measure when the foil is gonna break because you are predominantly measuring speed and torque and these kind of things, right? And of course you have no idea what is happening across the whole meters of these foils. What we are doing is because we can create the digital twin of this operation, this manufacturing step, we know where the manufacturing is happening. We have PLCs.
What we start doing is we start creating the executable digital twin that those executable digital twins are running in the virtual environment or industrial edge in the factory floor, so that continuously you are having two operations in parallel. One is in the real world with PLCs controlling the machines. And then in the virtual world, at the same place you are seeing what is happening. And the power of that is you can start measure or virtually sense a lot of the things that you can't in the real world. And in this case, they can find, okay, at what position the string is getting to a breaking point. And before the problem happens, executable digital twin can not only find the problem and it can find the right way to control it.
That means how you can change the speed or torque of the motor's controlling the winding machine. And it then sends that back through PLCs. So as you see, this is an example of finding the problem before it happens and doing the corrective actions. You can think of the same thing when battery is being utilized in a car or in a container energy storage system. with our digital twin, we can run it in the field in the cloud on the edge. So as battery's performing, we can also see what it should be doing versus where it is.
And by knowing the difference, we can allow companies to recall the product for servicing, avoid some of the very costly and sometimes life endangering events that has happened or can happen both for the vehicles but also for the battery parts that the companies are looking to put for energy storage systems. So this is the journey we are taking. As I mentioned, digital transformation is absolutely necessary if you are trying to innovate fast and trying to bring those innovations to the market fast in a large scale manufacture in a cost effective way. But at the same time, digital transformation is a journey.
There is no one button you can press and the whole thing is completely digital transformed. And I want to leave you with this key takeaway message that as you take this journey, you have to combine the digital world with the real world, and Siemens is driving this process. We would love to work with you as you take this journey. And before I end my speech, I wanna take a step back and look at what is happening in the world. Our next generation is looking at us to improve air quality, reduce pollution, and essentially do something that can reverse the course of climate change. These are big problems, but we owe it to our next generation.
All of us, actually every one of us in this room has a unique opportunity to address this call of action from over next generation by bringing better battery at scale and speed. So let's do it together. And with that, I'm Puneet Sinha. Thank you for your time and I wish you a very, very productive battery day. Thank you.
(audience applauding) (bright music)
2024-07-19 13:33