Data and Trust: Machine Learning and the Digital Transformation in #healthcare #ai #ml
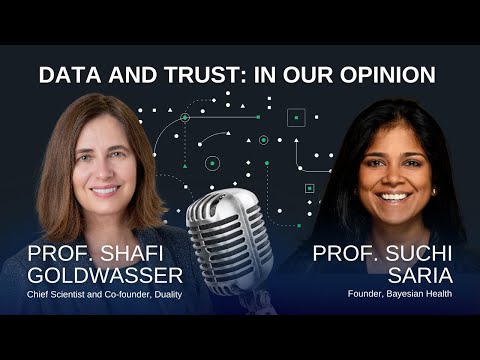
foreign [Music] I'm extremely uh pleased to introduce Sushi Saria who's on our scientific Advisory board but that's really the least of her achievements she's a world famous researcher and professor in healthcare and machine learning and uh thank you for coming today for this interview thank you for having me Shafi it's always fun talking to you wonderful and I think uh I'm sure today is going to be no exception so I um I was hoping that you could start by introducing sort of yourself and how you got interested in healthcare and statistics and machine learning yeah absolutely so um let's see I was very fascinated by computer science at a pretty young age which was atypical for people in India um my older siblings went to it so that's how I got into it and got into robotics at a very young age and uh naturally uh you know the idea of like programming intelligent machines was fascinating so I actually have now been doing almost a machine learning AI research for almost 20 years and um growing up mostly I loved math physics statistics medicine and biology weren't my thing and it was only actually 2008 kind of what felt like was going through uh an early middle life crisis where I was really trying to figure out how can we really take these incredibly powerful tools and start the problem solve problems in areas where there's so much immediate impact and um started collaborating with some colleagues over at Stanford medicine and it was really interesting timing because it was just around the time prior to high-tech act you know nationally there was sort of uh the digitization of electronic health records going from paper records to electronic records which means suddenly there would be this huge amount of messy real world uh important data that was going to become available but um we really in machine like you know people weren't really thinking about it it was like sort of this brand new very valuable uh new capability but we didn't know what to do with it and so as I started to think about well you know traditionally the way we've designed machine learning is not at all for data like that and started to design new machine learning techniques it started become clear like how much harder medicine was and what we needed to do but also how much more desperately we needed it here compared to some of the other domains because there's so many important decisions we can make if only we could figure out a way to make you know Stitch and make sense of this data and overcome some of the noise and bias and messiness and so that's really what got me fascinated took me to Hopkins where I was one of the first faculty between a point between engineering medicine public health and really pushing sort of this Avenue for building techniques for these kinds of data sets how do you build them in a way that is easy to use and then in 2018 I found out a company Bayesian Health that is also now bringing these kinds of state-of-the-art techniques to the bedside to really start to anticipate patients at risk for declining trajectories and providing real-time point-of-care tools that make it very easy for care teams to both identify anticipate and then treat like act in a timely way to prevent deterioration yeah it sort of seems amazing that people didn't work on on medical applications as the first application of AI methods but it's always security I guess vision is highly related to security applications or you know planes and so forth and identifying targets or financial markets So speaking of what you brought up this uh prediction of High um you know patients who are in that should be paid more attention to high-risk patients I remember that you were telling me the first time that I met you about your experience in emergency rooms and maybe you could tell us about the project absolutely so we've worked in a number of uh different areas I'll give you a couple examples um so one that is very exciting is um you know about a month ago results that came out that was uh three manuscripts featured on the cover of nature medicine it was almost an eight-year Journey getting here but it's one of the first instances of machine learning AI deployed at the bedside uh you know and showing the potential for using these kinds of predictive tools and saving lives and what we saw in that work was basically um sort of again this work was done in partnership with Hopkins and Bayesian but basically the system is kind of in real time pulling data you know surveilling patients this is in the in hospitals basically the second the patient comes in the Ed we're starting to read data that is getting recorded we're analyzing these data for patient at risk for various complications um like sepsis which is one of the leading causes of death in hospitals and in sepsis we know if you can identify patients early and in a timely way we know what to do to treat the big part is often we don't get to the patients early enough and so here the idea is we recognize these we you know we're monitoring them um the software monitors them surfaces the information when they sense a patient is at high risk and why that information is presented back in workflow within DMR in a way that's very easy to interact with and now the provider uses it it's almost like a software diagnostic right they're analyzing the information evaluating to see if it makes sense and then they uh initiate a substance treatment if they agree and uh by doing you know what we were able to see is that um in similar patients when providers were getting these kinds of data and tracking and treating in a timely way versus uh standard of yeah we were seeing like a significantly uh higher uh you know significant improvements in mortality morbidity let's stay in reductions in utilization and this is just one example I mean there are all sorts of things within the hospital we can monitor for but also there are lots of ideas outside the hospital so just in another example this is looking at patients with Parkinson's where we put uh you know like today part of the big problem with many of these neurologic diseases is patients uh can deteriorate but it's extremely hard to Monitor and measure progression uh typically they come into the doctor's office you know once every nine months or something like that and they run like a very you know like questionnaire where you know how you're feeling and the amount of tremor you have Etc and so the whole idea here was Well turns out you can take a lot of the data that you would have collected and find very close Target markers and actually additional markers on a phone that you can automate that now you have the phone that you're carrying it around with you it can start to measure the degree to which you're moving how quickly you're moving how quickly you're reacting lots of different kinds of signals like that and you can integrate that data to start to measure the same kind of test slightly different because it's more objective and with many more markers more holistic within the patient's everyday environment and you can actually start to track progression very differently so again another example of how machine learning with very messy real world data sets can bring so much new value so it's you know it's interesting I when I hear this besides the fact that it's fascinating and uh very um immediate for all of us uh either because of parents or because of grandparents or because of our future um I'm thinking that um I'm thinking about how do you actually uh compare say what you see in John Hopkins emergency room or what you see in your cohort of patients Parkinson patients who you've sort of um linked up to devices and whose data you analyze how do you compare that to how other emergency room uh uh procedures work and is it better is it worse or a cord or Parkinson patients of a different type of Parkinson or larger cohorts um you know and of course what comes to my mind is that comparison to others requires collaborating with others in some sense or at least achieving benchmarks uh you know means that we we we have to come up with a benchmark and then we have to compare ourselves to it which immediately I think of Duality that I guess one of the things that we are trying to focus on is collaboration in a situation that that is useful and one of the things that came up to me when you were talking is for actually evaluating success you know as comparing two different protocols where the other protocol might not be in your own emergency room yeah absolutely I think more broadly right um I think this is just more true in medicine than ever that like um you know for all sorts of reasons data style load in so many different places and you need the ability to um compute over lots of different kinds of data that may be in the same place that may not be in the same place and and um you know so how do you make um I mean so much of collaboration is hardly starting with uh trust right how do you build trust how do you get people to to you know deeply understand your intention for use of the data how do you make it so that you can uh enforce trust or ensure trust in a way that uh doesn't call for a lot so sometimes like the right software environments is a big part of it right today I think this is such a fast evolving field all the way from now there are new Federated learning approaches where basically you don't move the data you move the computer the data even in any form of Federated learning approaches the idea of like maintaining secure environments and then increasingly all the progress we're seeing on the you know increasing privacy side of privacy sensor machine learning right how do we make it so that we're able to um do leverage this data maximally without needing or requiring that everybody needs to sort of donate the data in a way that has no controls over it and that's something that um I think the more that science advances the more the whole field will benefit because there's just so much need for it today the way we think about it is very much in terms of you know secure enclaves that sit within individual health systems and we have software that runs Within These secure enclaves that we allow us to compare and contrast um you know the performance uh at one side versus a second site or to be able to monitor for deploying across multiple sites but it's definitely I think there are lots of uh uh I mean I think there are still additional controls that can be implemented that makes it even easier and easier to trust that uh you know the data can be used for good without needing to give all the keys away so um another question I guess is uh you know when you there's this Rosy picture right that we're going to have these analytics that will really tell us when we're going to get sick and if we do what to do and how to treat us in the risky times um and yet it's not it's not available right now there's some sort of a timeline and I was wondering uh what is a timeline and what are the barriers that you see to having this be the dream come true you know is it two years five years ten years yeah actually I think I think for a long time part of the challenge was we um didn't have we had these aspirations but we didn't have like high quality well-validated and you know tools or systems that really could show that these things are really working I think we're starting to see many more such examples like I want to say in the last couple years there's been enormous acceleration in terms of both the understanding of how to make sense of this data you know how to build tools that are like high quality that providers will trust and so now to me increasingly the difficult part and then you know with interoperability more and more data is now possible to stitch together so that's super exciting where I see lag now is basically you know the the science and the technology is advancing faster than the users like the health system leaders or the users of this technology right it's also new and they're in the midst of covert and they're just emerging and the world is moving forward so fast ahead of them in front of them and so it's like very bringing everybody else along to see one what is possible how do we figure out how to implement these tools how do we build trust with these tools how do we understand like how they're using data in a way that's beneficial not harmful and then some and then all the tooling we need so sometimes trust is built through education but other times trust is built through the right kind of tool sets right so awareness that there are now compute platforms that make it very easy to do things in a very secure way up until four years ago so here's something really like funny like four or five years ago very few health systems adopted the cloud right people were very skeptical and cynical of moving you know they relied on on-prem servers they didn't want to go on the cloud and I think that's like absolutely not the case today like most systems now have migrated the cloud because they realize it's actually more secure than working in an on-prem environment and much much easier to manage and so in the same vein it's you know uh so so much is happening so fast and it's creating awareness and building trust and um you know understanding like what's possible and and you know making it happen it's interesting that you mentioned uh covet is an inhibitor in terms of time obviously a lot of things stop working which kind of shocks me how how fragile systems were but uh it also seems to me to have been also an accelerator at least predictive models for predicting who is going to be a patient is going to be suffering uh from coven more than others and had to use data and had to use it quickly a I know that include in Israel there's you know collect Innovation they have published a few papers on this and I'm sure you've done it work on uh on on coveted data you probably couldn't help it because there you were and the questions were acute yeah it's so I think the landscaper looks very different country to Country so one thing that was super interesting was in the US for instance um you know like for example through Bayesian because we were plugged into some of the health system infrastructure we were able to start monitoring covet patients and start to learn from that kind of data to forecast which patients are at risk for deteriorating and then use that to drive some of the kinds of decisions like um you know is this person safe to discharge is this person going to you know deteriorate rapidly so maybe we want to escalate them and put them on a ventilator for example or to more tightly manage these kinds of patients so I think we saw all the possibilities we were you know there was so much excitement in terms of the analytics Community coming together to start building all sorts of new approaches with this kind of data NIH making all this kind of data available through registries one big challenge a hurdle I saw in the U.S was that we were able to start getting data sets we were able to start learning and implementing these methods but the ability to put it back into workflow so that you could start informing real-time decisions that that didn't exist and the reason is because you know the like the kind of infrastructure bayesians building is kind of relatively new the ability to go back and put real-time machine learning models to influence real-time decisioning is you know it's and so as a result you know we're early in in the you know the number of systems that we're collaborating with but system infrastructure of that sort doesn't yet exist you can kind of do that a little bit through the EMR by putting some simple models in place but those often require you know like clunky workflows you have to spend a lot of time doing educating there's lots of pieces to it that aren't today TurnKey so in some sense covet to me was the perfect example was so much need even lots of researchers you know jumping at the opportunity and demonstrating how we could do things differently but then the translation and the scaling of it struggled because we just didn't have the infrastructure here to get it right um in other ways covet accelerated things a lot because we have you know uh people again like I said didn't want to use the plot they didn't want to use Telehealth like they didn't didn't weren't that excited about digital Technologies and during covet there was no other way you had to jump on board and so that meant Health Systems now have to have a digital strategy they're thinking much harder about integrating virtual with physical care they're thinking much harder about data as a result because it's generating so much data so we ought to do something with it and then most recently the you know only worsening Staffing crisis is getting people to think about gosh we need better automation augmentation we need to do better in terms of managing you know like you know we need to figure out ways to do more with less active approaches of throw more humans that the problem isn't going to be adequate enough yeah so in some sense you're saying that there's probably not in some sense you're saying there's progress in the tools which is tremendous there's a lot more data out there that's being generated continuously uh some of it is all data that's been digitized some of it is new data that's been collected by new sensors and and devices you know thinking of from the perspective of someone who's worried about sort of privacy and cryptography uh throughout my career and also in Duality I guess um I agree with you that the thing to do is to make this sort of platform level so in other words have it all out there uh to with easy to use and then have some kind of uh hope that the world of health of a world of doctors uh sort of jumps on the and understands the benefit of the tools so the way I see it is that privacy is a is something that people naturally immediately say well what about my record is you know what about uh my data it might be worth something and I don't want to give it away if this could be done in International's level and a national level or even within a hospital there are different departments I understand for example kidney analysis is offered in different departments in the same hospital they might be even competing with each other for Market but they would help each other by you know pulling together the data um and you mentioned Federated learning which is in a sense a way to use the data without giving it all out because you might have incentives you might have regulations and so forth and we're trying to circumvent that by being able to work on it in an encrypted form as you know um could you see how Duality can help this uh this um Revolution or Evolution yeah absolutely actually I think it would be really exciting for you to summarize I think I don't know how many people in the audience kind of know what Duality does and I think it's fascinating and I think it's really laying down the infrastructure of what I think is so crucial so it'd be actually kind of fun for you to describe uh you know and briefly what Duality is doing right so we so I will divide it into sort of three product areas a one of them is how to in fact get different data centers uh to collaborate collaborate but not just by giving each other the data but essentially decide on a set of Statistics say or um Diagnostics if we use language of medicine then they won't they all agree they're gonna want to run but they want to run on the combination of the data and the combination of the data could be just more of the same or it could be that these different uh collaborators have uh different in some sense features for the same let's say client so they enrich the data and what we do essentially is that we have this cryptographic tools and one of the main tools is called homomorphic encryption that allows you each Center individually to encrypt their data but even though the data remains encrypted then there's a way to compute whatever algorithm diagnostic tool and so forth you want a so that at the end the results are available but you can't see the individual data if the results themselves are revealing that's a different thing but if very often the results are statistics or procedures and they don't necessarily have to reveal anything about individual data or they won't reveal so that's one set of tools and here the important thing is to come up with a strong enough set of tools so that they address all the kind of Diagnostics and new machine learning tools that are out there or being created and for this we have actually a very strong data science team I think any company these days that wants to specialize in collaboration with privacy has to understand the data science you know as well as the cryptography so that's one type of product the other type is where um you have already an algorithm or a diagnostic tool available and you now want to use it and you are a patient or a doctor that has access to the easel but they don't want to reveal the patients you know data but they can so we enable that to be done so without name and specific data still you can run the model and give the patient the result oh the agnostics was all the recommendation and the third type is where you actually want to hide your model so you might be a hospital or a clinic or a startup that has fantastic insights you'd like to provide them and charge for them but you don't want to share those insights so it's a way for you to sort of allow people to use your model without revealing the details of the model and again it's all using uh this either encryption method where you can encrypt and you can encrypt either the model or your encrypted data and yet run um you know more abstractly it's like run a program on an input without knowing what the program details are or the input but you can figure out what the output is or there's something called a multi-party computation which is another way to do it rather than encrypting everything into putting in the cloud and then working on it you can uh actively engage so if we were thinking about several collaborators they'll be sending messages to each other where they might be splitting their data and giving each Center only a share of the split and that's already a technical um question of how you split it but you split in such a way that you can work only with a share of the data without and that's not going to give you information about the original data but it will allow you to make progress and make a diagnostic um so you know I was using the term diagnostic this is applicable to a lot of Industries but I do think that the medical in healthcare and and I and I think the personalized Healthcare which is another thing that you're kind of famous for is uh has a lot to benefit from it a lot of sleep a lot of people know about me but nobody knows everything about me so Chevy I was going to say I think what you're describing where basically either multiple centers you know either a company can partner and deploy and provide tooling to multiple centers where at the individual centers they could use um your your dualities platform or homomorphic encryption to be able to run tools learn from that data without actually moving the data and without decrypting the data I think is I think that's that would be super exciting tell us tell me a little bit about if if a company wanted to be able to come and use this approach like what would they have to do today well they would have to contact us and then we would build it for them uh a toolkit that you know essentially will do exactly that um or if they're coming with the tool that they've already developed and they want to sell it to their customers we would make it so that their customers can use it without giving the data in the clear a so at this point you know it's not a one tool serve all but each tool each algorithm which machine learning method um if you want to make it efficient then you will need to design it so that it kind of works at its best capacity depending on how the data looks like and what the tool steps are um so even though you know homomorphic encryption there's something called fully homomorphic encryption so this is sort of a completeness theorem that says that you can run any computation any diagnostic when you really want to run a specific one you want you know efficiency and therefore you need to know the details of what that tool does um having said that uh you know there's a question of course about Real Time versus off offline so when you were just talking about the emergency room you need performance right there and then right uh and probably privacy is not of your utmost concerned because the patient is right there but when you want to build a tool for the emergency room that's when you want to have access to a lot of data and a lot of experimentations elsewhere to be able to run your own you know uh tools on and see what would be the outcome if you use your procedure possibly I think actually benchmarking for medicine is probably the one of the most interesting things which hasn't been done enough so there's always like the Harvard Stanford right there's that I think I've heard that terminology you know there's there's different John Hopkins there are different medical schools they use completely different procedures what is the best what is the best of which patient and how do you come up with a benchmark um and again to me privacy seems very crucial for them where you can contribute your data because you want to find out what's the average you know what's the best thing out there and then you compare yourself to the best yeah I think definitely there's sort of an element of benchmarking where systems are just reporting you know how they're performing and to say the US government or CMS and they're recording that but I think what is happening now a whole lot more is um systems Health Systems payers like many Health candidates are realizing the importance of learning not just sort of saying how am I doing but what can I do to get better at it and to get better at it is where there's like natural opportunities for novel tools that take lots of data and learn from these tools to figure out you know either new ways to identify disease early new ways to optimized treatment new ways to do uh you know matching for trials new ways for forecasting who's going to be responsive or not responsive to treatment so there are so many learning opportunities and I think in scenarios like that what you're describing would be phenomenal right yeah in fact I wasn't thinking about the reporting I was thinking more about the matching how do you match a patient to the best place to treat their own particular case it's like I always think about teachers there's a there's a a good teacher for everyone but they're not the same it really depends on the patient or the student you know and if we had the way to match the right teacher with the right student or the right doctor or the right patient we would do remarkably well so uh even with not going down all the way to personalized medicine but at least to have the you know the right Guru for the right uh uh Center to treat me and I don't you know today how do we do these things Word of Mouth do we Google it do we go to our doctor and they know their Network um I think that there must be better ways to read those conclusions um so I just wanna I I don't want to take much more of your time but you know you use the word trust and I think trust in healthcare is uh is a huge uh it's an old an age-old issue right who do we trust you know who's the doctor that we trust what is the health system that we trust it's um you know what's the digital health system that we that we trust and privacy is just one more layer on top of that that is do we trust with all that that our information is going to uh we're still going to own it after um we give it away and um as you said there could be a trusted Enclave uh there could be encryption or as as what you trust the strength of the encryption that physically the data is moved but it's moved and encrypted uh way so I think uh in in you know this issue of trust and online is a much bigger issue than trusting a doctor that you enter into his door you know because there we use these intuitions yes there is no intuition to use when you are using a digital platform uh in terms of trust I think it's a fascinating question yeah I mean I you know I I talk a lot to people in law and in policy and when they talk about computer algorithms that are going to now service substitute let's say to some Court decisions they immediately become very distrustful right as they should but I think you and I kind of trust technology and Trust algorithms as the question is that could have been to other people I think I think the I don't think they're wrong in feeling uh you know being taken aback a bit right because um we've had so many decades or maybe even centuries of experience interacting with humans we don't have the same mental models of computers and algorithms and certainly right now in the Press it's often sensationalized right something goes wrong they pick that and they write a whole story on it humans probably do poor things and make mistakes all day long but those don't make for great stories because you know we expect it so I think there's an element of again education awareness which is the you know like um in so many ways there is opportunity for benefit from having really high quality technological systems that can read the data objectively make sense of it and really both accelerate and optimize the quality of the inferences or decisions that are being made I have to say the flip side of it is also true because because these Technologies there's so much more that's become possible there are groups that are coming in and in a rushed fashion putting out ideas without adequate validation like they're not doing rigorous testing they're not rigorously thinking about the you know whether this biased whether it has any ethical consequences whether it's implemented in a way that's going to get you the right kind of results you're looking for so I do think the emphasis on needing to build trust and the emphasis on needing to build rigorous quality checks is so crucial because we we don't want to lose people's trust from creating huge mistakes and I think the reality is uh I think the right world is one where we have these systems they're rigorously validated tested and uh you know we're able to blend human and machine decision making in order to get the best of both worlds so what's your biggest aspiration or I mean what's your kind of technical or uh aspiration for the next I don't know 10 years so the thing that I'm really excited about is that now the data exists the infrastructure exists like I said it's really it's been like huge amounts of work to get to a place like it was it's so personally rewarding to be able to see that you know to be able to have been able to deploy thousands of providers using our tool adopting them and for us to see meaningful meaningful like you know nearly 20 reductions in mortality from the use of the tool and that's a huge first for the field to be able to show this kind of meaningful benefit like in terms of life saved and so my hope for the next uh what I'm super excited about is I mean there are so many condition areas where we're losing lives today where people are like experiencing like um more mortality morbidity that is completely unnecessary uh let alone the fact that it's just costing our system tons of money so I I mean the way I often measure impact is on the x-axis is you know life saved and then the y-axis is how I'm doing and I want to see that sort of go up over time right like so I want to be able to show that you know as we move past time as we measure our benefit in terms of life saved reduction mortality morbidity we're basically creating benefit and I think we're at a place now where that's very possible like at Bayesian for instance we've expanded in like what took us six to seven years to build now with the platform we're building in so many new clinical areas so much faster it's like how you know designing the first vaccine for kovid was hard right but then you can go into new types of viruses much much much much faster and so in the same vein that's kind of what we're seeing with um you know Technologies like what we're building in Bayesian but you know also I just see that as like where the field is headed as a whole our ability to be able to you know I I think if in 10 years from now the if I would be shocked if every physician in this country was not making at least three to five decisions daily from the use of computers that's like allowing them to optimize patient care and that and that means like you know impact in terms of cutting costs saving lives improving you know improving uh like lifespan which I think is amazing right like it's it's so long do fantastic yeah and I hope that in fact uh we can continue to collaborate and it you know sort of The Duality would facilitate this transition um it's definitely a Rosier healthier future uh thank you so much this is fantastic thank you Shafi for having me and finally [Music]
2023-01-09 15:53