Beyond the DORA metrics: Measuring engineering excellence — Thoughtworks Technology Podcast
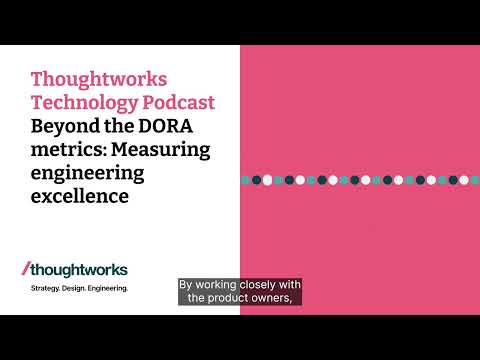
[music] Welcome, everyone, to the ThoughtWorks Technology podcast. I'm joined by my co host, Scott Shaw. Scott, do you want to introduce yourself? Sure. My name is Scott Shaw. I'm the head of technology for Asia Pacific Region in ThoughtWorks. I've been one of the regular hosts of this podcast as well. Wonderful. Thanks, Scott.
Today we are joined by two of our colleagues, Dinker Charak and Sachin Dharmapurikar. Dinker, do you want to quickly introduce yourself? Sure. I'm a product strategist at ThoughtWorks. I'm very interested in building products that matter, and metrics is a very important aspect of that, and a favorite subject of mine. Happy to be here. -Sachin? -Thank you.
I'm Sachin Dharmapurikar. I am product manager for engineering effectiveness solution. As you can see, it has metrics at its heart, and I have been working on EEBO, which is the topic for today for a couple of years. Super excited to be here. Thank you very much both for joining us.
It's indeed a pleasure to have you both. Like you said, Sachin, we'll be talking today about EEBO metrics. For our listeners, can you tell us what EEBO metrics are? It's an acronym, a little mouthful, but it will start making sense when we explain. EEBO stands for engineering excellence to business outcomes. I have been developer all my life, and we are really good at measuring engineering excellence metrics. These could be like test coverage, four key DORA metrics, you name it.
There are like hosts of metrics which we do, but rarely we talk about juxtapositioning of engineering excellence and business outcomes together. What we realize is that all business outcome metrics and engineering excellence are measured, but they are not put in perspective for the same thing to understand, what is the line of sight between my work and business outcomes? This was our philosophy, and that's why we created this. I've noticed a big uptick in interest in metrics in general. It seems like it's happened maybe since the pandemic.
People are a lot more interested in the value that they're getting from their engineering investments, I guess, but it seems to be something that I'm asked about everywhere I go, and customers and people in the industry want to be able to measure things now. Have you seen the same thing? Yes, we have, and this really gave us also the right push to move forward with this faster. Everyone is interested in metrics, though the only thing that we saw, which had us worried is that people are confusing different purposes for looking at the metrics. Some people are looking at-- they're mixing the purpose of figuring out inefficiencies in a value stream, versus looking at how we can help a developer grow as a developer or any member of the team for that matter, and how to look at the outcome of the team, how to look at the excellence of a team, and how do you look at the business outcome? Somewhere everyone is interested in metrics.
The world is full of metrics now. As part of this research, Sachin and I did some analysis, and we figured out that just for product, there are around 700/800 metrics floating around. Everyone is excited, and one of the things that does happen is that everyone starts creating their own. I guess you're making the point that there are a lot of metrics, and there's quite a bit to choose from, but why did you see the need to create a metrics framework, if I may use that term, of your own? Our goal was not to define a set of metrics. EEBO rarely wants to talk to you about, "Hey, use these 5 metrics or 50 metrics or 800 metrics," which Dinker said.
We are, okay, you follow whatever set of metrics you want to arrive at. You can come through OKRs. You can come through your engineering practices. The goal is the philosophy of what to measure, and how to put this thing in context is what we have a take on. We believe if you are working on an engineering stream of work, then essentially it is supposed to impact business in certain positive way. If that is true, then have you identified the definition of success for your team? In whatever metrics philosophy you are following, have you brought that, and how you have connected that to engineering work, which is happening within your team? That's the only take which we have.
Now, as Dinker was mentioning, although there is an interest, people are also working with some of the older practices, not bringing business views into it. There are a lot of anti patterns. Engineering effectiveness and business outcome metrics framework had to also take two step down approach and give them some sort of indication about what we believe is good metric, what we believe is a bad metric. These are only guidelines, what is our core is focus on creating that line of sight between your engineering work and your business outcomes. The goal of tying engineering work to business outcomes has been a Holy Grail for a long time.
I think that everyone talks about business value in engineering, but I think very few people really, when it comes down to it, are you able to pin it down and have a definition? Do you get closer? Are you able to get close to that? Everybody wants it. Everybody wants to do it. There are a couple of things which prevent teams from doing it though, and one of the reason is the culture of the organization. I have never met an organization which does not measure business outcomes.
They measure revenue. They measure conversion rates. They measure the profitability, all these things. Just, those are not done by the same set of people who are closer to the engineering teams. They are shared in a very aggregate manner with very less frequency, or they are told as, "Oh, by the way, this something great happened," or, "Something really bad happened, and we need to buckle up." It is not shared as a progress indicator. The second problem is people declare these goals at a high level in engineering organizations.
For example, we are building an e-commerce platform to reach one billion dollar revenue target in next two years, but they do not break it down into each program. If I am working on let's say the search stream of work, I do not know what it means for me to impact it positively. What will I need to do, or what am I doing today? Am I improving the business goal or not? You told me one billion, it will take two years, I cannot wait for two years to know whether my work is impacting positively or not. It requires that breaking down, and that is another struggle.
Although it's a Holy Grail, everybody wants to do it, people are struggling to find a good path between where they are to reach this Holy Grail. Thanks, Sachin. Our listeners might probably relate to this a lot better if you can give us some examples.
I know that you said that there are no specific metrics that you recommend, but are there examples of good metrics and bad metrics? The framework, and let me use this opportunity to kind of draw that continuum also. As the acronym says, engineering excellence to business outcome, so it has a left-hand side, it has a right-hand side. Left-hand side is engineering excellence. If you think of it, engineering excellence has two parts.
One is excellence in software development, and one is excellence of the software while it's sitting in production. Excellence in software while sitting in production is actually well-covered by DORA. It has been adopted. There has been a lot of benefit for it, so that covers that aspect. How about excellence in software development? If you look at it, there is a focus on, "Hey, we are doing certain activities which reflect the excellence."
In that case, are there any minimal set of metrics that a team could start with? We do have some thought on that. One of them is take debt, the other is build failure rate, and the third one is number of security issues that you report. If you look at these three, these will give you an idea about the excellence of a team in software development. DORA four key metrics take care of excellence of software in production. On the right-hand side business outcomes, it's very difficult to come with three or four set of specific metrics, which makes sense to a wide variety of businesses. However, when we did our research, and we were looking at various business outcomes that teams are using, they fell into four categories.
That is the four categories that we recommend that you should have a few set of metrics at least so that you have a good coverage of the business outcome that you're looking at. These four metrics, the first one is improvement in efficiency and effectiveness. That's the most common one, that everyone is looking at it. The second one is improvement in experience. That is very upcoming, especially, and very common in B2C scenario.
The third one is increase in influence. How is this product going out and increasing its influence in the market? Startups, for example, may not worry about revenue in the early days, but they would really want to know what's the influence their product is creating in their target user. Fourth is market sensing. What information can I glean from these metrics so they can tell me that does my business model hold? This goes back to, we start with the business model, we go out in the market, it teaches us something else, we bring it. To answer your question, are there a specific set of metrics that the team can start with? We would request the team to look at this whole continuum, and we have some suggestions where they could start.
I think this, and that's why we call it a framework, I hope this helps. Prem, I would like to continue that example of search team. If let's say I'm the lead engineer of the search team, and I want to figure out business outcome metrics, the four categories which Dinker mentioned.
The search effectiveness and efficiency would be a simple, "I would like to look at the response time which I'm generating in my code. I would like to understand that how much product I have actually, if there are like five pages on a mobile screen, which I need, five products I need to display on the product, which link people are choosing that is indicating the relevance of my output." These are like efficiency and effectiveness metrics, if I would like to measure. Business outcome-wise, they link directly to financial impact because the funnels are driven through these type of indicators. If you are thinking about future sensing, you can start understanding some of the products which get top-notch priority, example is, let's say you are showing some of the promo products onto your page as the first result, and those are causing people to drop off. Then you can sense that people are not getting interested into these promos, and you can give this feedback back to the business, and they can figure out what are the strategies.
These type of inputs can be extremely useful for them. The third one would be is, you are trying to figure out if I am increasing the influence, my goal is to make 200 million in this first year through product sales and merchandising, but for that, my conversion ratios has to match certain level. By working closely with the product owners, also as business people, I can figure out how long I have been into this journey. I can give you this real example that on one of the projects which I was working, by just having 1% drop into conversion ratio, there was a huge havoc in the business rooms.
Because it was lesser 1% than the older system, and funding people were asking, "Older system was better because the new system has 1% less conversion ratio." This matters a lot. We had to do a bunch of things from engineering team to bump up this and match 1% up. I know it is very critical that engineering teams have some visibility into this. It looks like you're suggesting that engineering excellence metrics and business outcome metrics, which were previously, maybe used by two distinct sets of people.
Now, we surface on a single pane of glass so that everybody can establish a stronger correlation between the two. Is that right? Exactly, Prem. While business is tracking things on some other place through product manager, some abstracted data is coming on to the team, and the product manager is also abstracting out, it's coming to the development team.
What always used to happen is, especially the development team, which is working on a product which touches the customer, maybe the app or the website folks, they actually used to get this data in a meaningful manner. Rather, let's say the platform team, which is like one or two degrees away from that point of interaction. They used to be in complete dark, and sometimes even complain that, "How do we figure out how we are contributing to the business outcomes?" The way EEBO Metrix started was a dashboard in which we had this left-hand side and right-hand side juxtaposition next to each other. There could be a visual correlation. Yes, later on, we actually developed some statistical method. That also has helped establish a statistical correlation between the two.
It's very important that engineering team sees that the investment we are putting in excellence, the effort we are putting in doing things in a certain way, bringing in good practices, they do have a correlation with the business outcomes. One of the things that I've picked up from talking to you guys is that the importance of the multivariate metrics. There's a lot of risk when we start measuring things, that we set a target, and then that people start to change their behavior to influence that one thing that they're being measured against. Does this help? Does the multivariate nature of this approach mean that it's harder to gain? With EEBO, we don't give you a prescription of metrics, that these are the only metrics you should use with EEBO, but you are absolutely spot on when it comes to not choosing metrics which are like singular function, and they are focusing on one thing.
There is a general rule which I recommend anybody is, do not create any metrics which are targeted at an individual, and that fails, and in fact, it hurts you more than benefiting you. The reason why we like the multivariate matrix, example is, four key DORA metrics is a great one. It is, the abstraction level at which it sits allows you to simply not-- you cannot hide behind them. Example is lead time.
Let's say you are measuring to me based on the lead time which we achieve in our team. The simple thing would be is. I would like to start deploying more often.
Like with my existing gates of quality control, and then upgrading the build from one stage to another to production. If my quality gates are not optimal, essentially, I will start experiencing more escape defects to production, failures in production. If that is indicated in meantime to restore or change field percentage, four key metrics goes down as a whole. Your lead time is spiking up, but you know what? Your other metrics are degrading significantly. That's why I like four key DORA metrics a lot. Similarly, you can do that in the rest of the engineering metrics too.
If anybody wants to gain, example is, one of the bad metric which I want to take example is number of comments per day. If you are trying to measure a team or an individual based on number of comments they do per day, that is going to be extremely detrimental because, there is a very nice study from '50s, like almost 70, 80 years old, and that law is still good for us. The name of the law is Goodhart's law, created, by an economist, but it is very applicable to software engineering. Anytime you create a measure, which becomes a target that seems to be a good measure. If you are measuring me by number of comments per day, I will start making more comments.
I will start putting spaces, [chuckles] and commas, and then formatting, and that is not adding any business value. That is not something which is desired by the engineering organization, but I will start shining onto these metrics which are meaningless to me. I would refrain from using such type of metrics, but make sure that the metrics are multivariate, like four key DORA metrics, or technical debt, or business outcome metrics.
I'm happy, Scott, you caught on to that. There's one more layer that we put on top of it. They should be multivariate, and they should be outcome-oriented. I'll give you an example. We were working with a team which had figured out that the code commit frequency is a good way of measuring the team's excellence.
We started talking to them, "What is it you're trying to achieve? Why this one?" It turned out that in their mind, they had correlated code commit frequencies with time to market, because you're writing more code, you're checking more code, and it's going through CICD, and the feature is going faster to the market. They said, "Then why look at this? Why not just measure the time to market and focus on that?" These are the small things that people need to consider when they're looking at metrics on how to differentiate between something which is output-oriented while they should be focused on something which is outcome-oriented. Multivariate is a very important part of that, and focus on outcome metrics is another.
Quick clarification. When we say multivariate, and tell me if this understanding is wrong. I think what you're calling multivariate is something that is constructed using other scaler metrics. Lead time is one, and is a scaler metric, and then meantime to recovery is another. Then now you are suggesting that you combine these two as one concept so that you don't draw the wrong conclusions.
Is that what you mean? Yes. The principle behind is that bunch of things need to fall into place for this metric to perform. That's the principle behind it. Some of these could be actually operational things.
Some of these could be good practices. Some of these could be trained on certain aspects, and you could measure all of them in a different way. Those are the operational metrics or KPIs for those activities, but all of these things need to fall into place.
If you have a metric which performs well when team does well in four or five aspects, you have now made it a well-rounded progress, well-rounded measurement rather than as single variate function, which always leads to gaining. Your point is absolutely right, Prem. When we are talking about multivariate, we are talking about, for one metric, multiple either processes, or tools, or techniques, or even capabilities can impact adversely.
It is not enough that one of the variable will make it good or bad. For lead time the example which I took earlier, if let's say one of the executives will focus razor sharp on only increasing lead time, hypothetically a bad idea. Let's say that they want to do that, and they don't want to care about the rest of the three DORA metrics. They just can't say, "I will do it by just committing more often to production. You will need to have your DevOps pipeline properly configured. You'll need to have ability to promote the build into a proper way.
Do you have enough confidence under the quality of tests which are your quality gates?" If they start failing on this, rest of the DORA metrics will be not ideal. Now, if they want to do that, they can improve lead time, but that's not good for business. That's why we love, any time, any metric you want to pick is for a team focusing on outcome, and actually is a representative of multiple processes, technologies or tools, it'll be better for you. Got it.
It looks like these metrics are meant to be used at a team level as opposed to an individual level. I think you also recommend against using these at the individual level. Is that correct, and why do you do that? We are talking about business outcomes. The best way to organize folks who are working and to measure them is look at set of folks who are working towards those business outcomes, so that becomes your team. It's how the team is working towards achieving those business outcomes, what kind of practices they're introducing, what kind of tools they're using.
It's a very good way to measure the excellence of a team, the outcomes that a team is delivering. However, on the flip side, it's not, EEBO metrics, this framework, this thought process in itself is not the best way, or even it won't make much sense if you try to look at developers or individuals looking at this. This metric in itself, we recommend it for team, not for measuring the individual performance. How do you know what's a good value of a metric? I'm sure there's some noise, there's some natural variation in these things, and is there a way to compare to industry standards, or what should we be striving for there? You're absolutely right. Sometimes we have gone to some customers, and vis-à-vis, we are covered on engineering metrics, by the way. We have seen some of the commercial tools or even open source tools, which have let's say 20 or 30 fancy, colored charts, which are just popping up.
When you do that, if you go and look at each individual metric, they have a reason. Sometimes these metrics are created for point in time problems. I'll give you an example of, if a team is suffering from a lot of defects which are going to production, and their hypothesis was that because of there are too many defects in production, I am losing my productivity on engineering teams.
Many developers' capacity is going in addressing these P1s rather than making valuable features. They created this new metric called-- added one to dashboard called Escape Defect to Production. For next six weeks or eight weeks, they will solely focus only on that metric. What happens is when you add this metric, essentially you are kind of creating an illusion that you are ignoring rest of the things, and people start becoming defensive.
People start only focusing on that. The simple impact is, your developers should become very cautious to put code into production. They will say, "I don't want that on that list of escape defects," and Sachin was responsible for that bug. QAs also will become more defensive, and it will hurt your lead time badly.
Now, when these type of practices you introduce, metrics are also like code. I have never seen people deleting code once it is gone to production. Similarly, once a metrics appears on dashboard, it's extremely difficult for it to get rid of, even though it was only for that one month or two months.
The good metrics, if we want to recommend anybody is, please do not create metrics which are for that point in time paying, but focus on these larger conversations about some good metric which are multivariate, outcome-linked, and you want to have a conversation. Example would be, if your production is having a lot of bugs and your productivity is getting lower, you need to have a conversation around why our change field percentage is too high, or meantime to restore is too high. What can we do to do that? You can have a drill-down metric for transient stages which you are going through, but do not put that into your main dashboard. Otherwise you will have a deluge of metrics that you have multiple metrics, 30 metric, 40 metric, but by looking at it you don't know what to do with it. This, Scott, introduces the third concept that we started formulating as part of EEBO metrics, and that is fully modeling of a metric. When usually people talk about a metric, for example, lead time, they'll say, "Hey, my lead is five days."
Those who have data background will quickly relate to this, and database background modeling of a metric. If you're modeling a metric, you're not just stating what is my measurement today. You also model what is my goal. You also model how long will I measure so I get a very good current baseline? The other one should be that, "Hey, if I am setting a goal for myself for this metric, is it really achievable for me?" That's where industry reference comes into the picture like, "How are other teams which are building something similar in the similar industry, what are the baselines they're monitoring?" Finally, the ownership metrics which Sachin also alluded to, who owns this metric? Who is responsible if the metrics starts performing not that great? Who gets to say that, "Okay, now we have this. What we were monitoring it for, that thing is gone." This fully modeled metric was another concept.
I'll quickly recap multivariate outcome-oriented, and they should be modeled properly. Awesome. Sachin and Denker, this is great, right? Are you using these EEBO metrics at any of your clients, and can you also tell us what kinds of benefits they're seeing from using these metrics? Not only with the clients, we are using it internally also, right? That is a great thing. I want to take two examples. There's a large bank in Europe where the CIO wanted to measure the excellence of the team. The problem statement that CIO had was very interesting.
It said, "If I look at DORA four key metrics for all my teams, all my teams are doing very awesome." The team which is doing worse is actually the platform team, because they do not have that much of lead time, and they're not elite, like check in multiple times a day. But I'm the CIO, I know these teams.
I know actually it's the other way around. The platform team is actually doing very good. What we are doing wrong, and that's where all these concepts came in. "Hey, platform team, you should not look at-- every team does not need to be elite."
For a platform team, let's look at, what are other platform teams in similar domain with similar functionality doing? Let's look at that industry, benchmark, then compare against that, are you doing good or bad against that, right? The other thing they had was that we have multiple teams. They had score, tribes. How do we roll these metrics up so I get a CIO view? Which was easy. Then we stopped the person from saying, "How can I go the other way around so I have a developer view?" We said, ''Organize these metrics around a set of folks who are working towards some outcome." There's another organization in Australia which has adopted it.
What they really love is the transparency it brings and the consistency it brings. Every team is now saying that this is what we mean by lead time. This is how we are computing it. If there are multiple teams working in different locations, you can now get a sense that team is working on this product. Then this technology, so this is their lead time.
It becomes an internal reference for other teams that, "Hey, we need to do this." Then they can talk to each other and say why we are ahead, or we are behind. These client stories do call out, what are the benefits of adopting this framework, other than the obvious one, where they are looking at all these engineering excellence metrics. They're statistically creating a correlation with business outcomes. The teams are getting a sense of how their hard work is directly affecting, and not just the team which works on marketing website, but the platform folks also. Wonderful. This sounds really, really inspiring.
Are there other things that teams can do to get started? Do you recommend something, other tools, techniques that you use? In terms of techniques, we believe any leader who wants to start doing measurement in their organization, they should think about a couple of steps before even they put one metrics onto paper. These steps are, first, go and talk to your team, and make sure that they are aligned with measurement of business outcomes, as well as engineer excellence. Explain your intention.
We are doing this, looking at our overall impact on business outcomes, as well as we are trying to use this as a feedback for ourselves to improve." That's the story. If anybody on the team is starting to feel insecure that, "Oh, by the way, this is a performance review, this is a performance measurement," that may start creating long-term culture impacts, which you are unintended by you. The second step is essentially start modeling the metric, which Dinker was talking about. There are some tools which we are giving to people. On our website, we talk about these fully model metric aspects.
There are some resources available. Third is basically, you start using these measurements manually in any form or shape you want. If you don't have tool, do not wait for tool. Tools are not super important, but putting these into some information, radiation space is extremely important.
Even Excel sheet will work. The last one I would say is, if you want to automate, there are many tools available in the industry. Polaris is one of the tools which we have built for the community. The goal was to just show reference implementation of EEBO, and make sure that people can see the benefit in action.
There are multiple steps you can do. Thanks, Sachin. Any parting thoughts before we close today? The most important thing that I have learned in this journey is that metrics are serious business.
The problem is that the request for a metrics, and the start of work towards a metric trivializes the importance of it. Many people just get up and say, "Hey, can we measure that?" Long-lived teams will tell you that over a period of time it has a cost, it does distract. Because in the beginning not much thought was put into it. Most of the time it digresses the team towards making sure that.
Metrics are important. Think before you ask for one, model them properly, make sure that they are not prone to gaming or creating local optima. Do make sure that they are focused towards the outcome rather than looking on the other side and say, "Who do I blame?" That's from my side. Sachin? That's great advice. I like that.
Awesome. Thank you very much, folks. This was a really invigorating conversation for me. I really enjoyed it. Thank you very much. I really appreciate all of you taking the time, and until next time. Thanks for having us. Thank you.
2024-02-03 01:19