Alison Gopnik - Transmission vs.Truth, Imitation vs. Innovation: What 4-year-olds can teach AI.
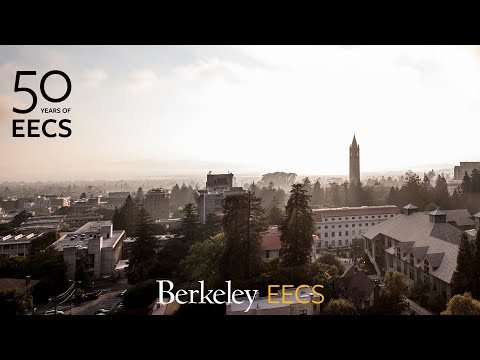
for know one okay um so welcome everyone um and um it's it's a it's it's a real uh pleasure and an honor to introduce our speaker Alison gnik who um alosia here um calls a campus treasure very uh appropriately so Allison has uh has devoted her career to thinking about uh to establishing this the the case that uh that babies are much much smarter than we than we think and more recently to to understanding what we can uh you know what we can learn from babies when we are when we are doing uh designing uh artificial intelligence um she uh she recently I think this year got the ramen heart award which is um which is um uh you know for for her for her life lifetimes work in uh in the foundations of cognitive science um it's um if you will uh it's the touring Award of uh of cognitive science uh so um so when my when my son was two years old uh I read Allison's uh book on the scientist in the crib and um it uh it resonated with and Amplified my own feelings about the subject so so following that I decided to to uh uh you know to to experiment with abandon on on on my son and I'm sure that um when you know later in life when he inevitably blames his parents for ruining his life uh we'll actually have an accomplice in it so thank please welcome and thank you all thank you all so much for uh let's see is this is the probably don't really need it let's see if this is working is it is the this on yes yes no yes okay okay great um I think I can just talk anyway uh so thank you so much for having me um what I'm going to do today is try to get through a number of different ideas and a number of different research uh lines of research work and I'm no no but oh yes now it's red is that right oh it's not M okay yeah okay um and I'm going to sort of speed through at lightning speed and if you want to know more then just get in touch and we can go into more of the details um so what I've been doing as omes said oh sorry and if you want the general picture that I'm going to be talking about here in some more detail this is a new paper that just came out in an old-fashioned peer-reviewed um Journal perspectives in psychological science and it talks it will talk about it talks about the general theoretical ideas and it will give you details about the first couple of studies that I'm going to talk about and then I'm going to talk about a couple of studies that are just in progress at the moment okay um so what I've been doing for the last 20 years is trying to follow this injunction of Turing so in the famous Turing paper the imitation game paper he uh see if I can try to get rid of this in the famous Turing imitation paper he talks about the fact that rather than trying to understand uh instead of trying to produce a program to simulate the adult mind we might be better off trying to produce one which simulates the child and there's this really interesting shift in that paper where he says no actually this is the right Turing test and the reason why he says that is because although the adult mind might tell you something about what a mind could actually do the important thing about children is that they're actually learning to do all these things from their experience in the world and Turing suggested that would really be the test of intelligence and I agree with him and increasingly as AI has relied more and more on machine learning um people in artificial intelligence and computer science have agreed with him so if you want to have a system that can learn to do things from its experience then children are the best system we know of to do that and looking at children figuring out how it is that they can do that can tell us something about how we could have an artificial system that could do that but at the same time trying to design artificial systems that can solve those problems um can help to explain how it is that children and therefore all the rest of us understand and know as much as we do so I'm going to talk about some of the general um I'm going to talk about some of the general morals that come for thinking about AI from thinking about children and here's the first one here's a very common and I don't think even really caricatured view of how people think about AI including people in in AI themselves and this is this view of AI systems as being like intelligent agents like people out there in the world and in particular that these are systems that either or both are geniuses because they're super intelligent or they're Golems because they're super powerful and evil and sort of the picture is that there's this thing called intelligence and you can have a little of it or a lot of it or a whole lot of it and the more you have of it the smarter you're going to be but also the more dangerous and Powerful you're going to be and that's very much the sort of implicit picture of AI and it's been the picture of uh the uh large language models that have recently gotten so much attention in AI um and what I want to argue is that that's a really wrong picture of what intelligence is like in particular if you think especially about children you realize that there's no such thing as general intelligence whether you're thinking about it as natural general intelligence or artificial intelligence instead what you have are many many different kinds of cognitive capacities often quite profoundly different from one another that human beings and children use to make their way through the world and not only are there many different diverse intelligences but uh something that comes from development is that they actually are in ttention with one another they trade off against one another so that it is literally impossible to maximize all of those intelligences at once when you're when you're using one kind of intelligence you're trading off against another kind of intelligence and in particular the three that I'm going to talk about today that tradeoff is very well known and understood in computer science for the first contrast between exploitation and exploration but I'm going to talk about a third kind of intelligence transmission or culture which also has tradeoffs with the other two with exploitation and exploration so as people here I'm sure know um there's this basic tension between a system that exploits that maximizes utility or maximizes reward and a system that explores it goes out and finds out about the universe figures out what the world around it or the environment around it is like um and in the long run having capacities for exploration seems to help you to exploit but in the short run the kind of intelligence that you need to explore can actually be in fact characteristically is intention with the kind of intelligence that you need to exploit and in a whole other talk a whole other set of papers I've argued that childhood is actually Evolution's way of resolving this explore exploit tension and doing something like simulated and kneeling so the idea is you get this early period where you can explore and then you can put that exploration to use to exploit uh later on in in in life uh yeah I'm trying yeah I was trying to quit Zoom but it was maybe if I just get rid of the Wi-Fi [Laughter] explore let's see let's force quit Zoom excent okay my joke part of the lecture yeah part of my joke about this is when they get the AV right I'm going to start really worrying about the robots until then I'm I'm not like if they can't manage a PowerPoint then they're not gonna they're not really coming to take over and I have noticed that when I the more sophisticated and computationally the audience is the more likely it is that the AV is not going to work so I have never succeeded in giving a talk a Google without a failure so you guys are in good company um okay so so we have these two very different kinds of capacities for exploitation and exploration but there's a third one uh and it's interesting from an evolutionary perspective you could argue that the exploitation kind of intelligence is what any living creature has to have just to be able to live the exploration capacity the capacity to represent the environment and then use that representation and knowledge is what you get when you get a brain that's kind of what brains are for um but this ability to transmit information from One agent to another in an efficient way is something that seems to be very characteristically human it's what gives us our capacities for culture for example so this third capacity is not using representations to do things in the world and it's also not making sure that those representations are accurate it's being able to pass representations on from One agent to another and to be able to acquire representations from other agents um so what I'm going to do is talk a bit more about this tension between uh transmission and exploration um which is a much less wellknown but still an important tension like the exploit explore tension um and what I want to argue oh another thing to say about this is that as long as we've been human we've had Technologies which have assisted Us in having these cognitive capacities so you could argue that technology itself any kind of tool is a way of increasing our capacities for exploitation um things like microscopes and telescopes and the institution of science are technologies that we use to increase our capacity to represent the world accurately um and what I'm going to argue is that we could think about llms as being technologies that enable us to transmit information more effectively and efficiently so this is argument uh this is this is just re this is rephrasing what I said before there's a fundamental difference between the kind of cognitive capacities that enable new discoveries about the external World things like exploration perception causal Discovery induction things that let us go out into the world and find out new things about it and the kinds of capacities that enable this kind of faithful cultural transmission things like imitation or testimony or statistical pattern extraction from information that other people have given you and there's an really interesting developmental cognitive science literature about things like over imitation for example when people imitate everything that they see someone else do even if it's Irrelevant for the actual structure of the task and we all kind of know examples where our tendency to imitate what we see other people doing our tendency to accept information from other people actually plays against our capacity to find the truth or our capacity to be effective agents in the world that's a very common kind of tension um and what I'm what I want to suggest is that the right way to think about large models is as a kind of cultural technology a kind of imitation engine and in the history of humans we've had a number of these kinds of cultural Technologies technologies that enable us to get information from others more effectively You could argue that language is so sort of er cultural technology it's the way that humans in contrast to other animals can easily get information about the world passed on from uh from one person to another um and writing of course expands that capacity so once we had writing then not only were we getting information from our local postmenopausal grandmother and by the way there's a whole other talk about how postmenopausal grandmothers are the key to this uh human cultural technology um not only were you getting inform could you get information from your own granny but you could get information from lots and lots of other Granny's across time and across space and Innovations of printing again just turn up the knob on how many people you can find out you can get information from effectively and we also in parallel started to have institutions that enabled us to search through all that information effectively so you have things like libraries cataloges IND indices things that enable you to take that information from other people and actually make sense out of it and extract the information that you need from it and of course more recently we have things like internet search Wikipedia or examples of kind of computationally based cultural Technologies and that's a very different picture of what these models are doing than the kind of intelligent agent who just you just need a few more turns of Mo's law and it will be the super intelligent robot that will come and Conquer us all um now on the other hand this is not intended to be and is not a kind of reductive story so uh these cultural Technologies aren't just sort of it's not it's not to say well this isn't so important because it's just to cultural technology these cultural Technologies literally have changed the world um arguably they've changed the world more than any of our other Technologies um uh and there's some interesting arguments in the literature about cultural Evolution that um that our capacity to use these Technologies is indeed one of the most distinctive characteristics of human intelligence that we are in this cultural Niche where our capacity to share information pass information on from One agent to another that's really the thing that defines our success that's made us more successful or not depending on your point of view temporarily at least than other animals um and from the time the cultural these cultural technologies have started uh people paying attention to them and thinking about them have pointed out that they have both positive and negative characteristics this is a famous wonderful quote from Socrates about writing and Socrates thought that writing was a Terri idea he thought that if you started relying on written information two things would happen you wouldn't be able to memorize Homer anymore right no one you'd lose your memory uh capacities for memory and you would believe that something was true just because it was written down so the kind of Socratic dialogue right that's crucial for that's crucial for as far as socres is concerned for truth and knowledge doesn't happen if you've got a book right uh and he thought essentially that these were misinformation engines and he was right right um that's exactly true about print that's about writing that's one of the things effects that writing has and I especially like this last uh sentence where he says they seem to talk to you as though they were intelligent but if you ask them anything about what they say from a desire to be instructed they go on telling you just the same thing forever which sounds a lot like chat BT so this is Socrates with you know chat BT cira you know 00 BC which is this this terrible new invention of writing um uh this is even more dramatically true when you think about print and I've gone down this whole rabbit hole that I could spend hours talking about about thinking about the effects of print in the late 18th century there were these tremendous advances in printing technology so you know there's Gutenberg who does the has the basic idea but there were big big Revolutions in printing technology and essentially what they meant was that anybody could print a pamphlet so anybody who wanted to could print something and distribute lots and lots of copies of it and there's a very good argument that that technology was what enabled the enlightenment that enabled all the changes in the in the late 18th century or at least it played a crucial role in those changes and this is actually Benjamin Franklin who you know famously was a printer and a printers apprentice and he's actually printing copies of things like uh Tom Payne's common sense and uh uh the Declaration of Independence and all those kind of uh critical pieces of the American Revolution so that's the good story um the great historian social historian Robert Darton um actually did something very clever which was instead of just reading all the good famous pamphlets he just read all the pamphlets so he read all the pamphlets that were being produced especially in France in the late 18th century with his new technology and you will be shocked and surprised to learn that most of what people do with a new technology like this is produce softcore porn or hardcore porn sometimes um and misinformation so let the mean cake is actually a meme it was actually a misinformation meme that went from one pamphlet uh to another this had consequences right this this it's not it's not like this was just you know a incidental thing arguably the print changes in print technology led to both the American Revolution and the French Revolution um uh I was reading this wonderful biography of Samuel Adams recently and there's a great passage where Samuel Adams is literally making up the Boston Massacre in the print room of the newspaper the night after it happened so this weird ambiguous thing happens and he writes it up and he gets Engravings from Paul Rivier and it becomes the Boston Massacre that's famous in American history okay um so what do you do with this what do you do with these cultural Technologies how do you manage to master the good and bad and I think it's this is not rocket science what happens is that you start getting Norms rules regulations institutions laws that enable you to have the good of the new cultural technology and keep the bad under control things like testimony under oath and editors and fact Checkers and all these things that don't exist before the 18 century when you have these when you when you start having these uh technological changes um and of course that's what we're doing now uh with AI okay so now I'm going to so that's the argument that I want to make about uh large models and many other kinds of AI rather than thinking of them as intelligent agents you should think of them as these powerful cultural imitation engines now I want to shift gears and talk a bit about the other side so if transmission is so important and indeed it is um even young children are learning through imitation and testimony what's missing from something like a large model what kind of intelligence is missing and I want to argue that it's this kind of exploratory model building intelligence that's missing um and as some of the people here will know we I've been part of this DARPA machine Common Sense program and I discovered the main thing about DARPA is make making up acronyms so our acronym is model building exploratory social learning systems and I'm going to talk a little about the model building and exploratory part of that story um so we know from work 20 years of work in developmental psychology that even very young children are constructing abstract causal models from statistics and they're actively learning through exploratory play and some of you have seen this before but still I I think to make this Vivid here is actually a four-year-old who is has gotten one of our the machines we call a blicket detector that we use to figure out children's learning and in this particular case he starts out seeing that yellow makes it Go and red doesn't and then he sees an anomaly and the study was just supposed to be about will he change what he does based on the anomaly but here's the kind of thing that we actually saw oh nothing this one's L up and this one's not so that means let's making this right up I always hear if you have any doubt that children or scientists just look at that facial expression we all we all recognize that we've all had that feeling oh it's because this needs to be like this and this needs to be like that that's why way this is his next suggestion what should we do now oh because the light goes only to here not here oh the bottom of this box has electricity in here but this doesn't have electricity MH um it's it's lighting up so we need to put four so we need to put four on this one to make it light up and two on this one to make it light up okay so I you know aside from being amazingly cute and I feel like we have a moral responsibility to show cute videos if you're a developmental psychologist um there's two really interesting things about what that little boy is doing and that's actually kind of characteristic of what we see when we give children these kinds of problems one thing is that he's exploring the space of possible hypothesis in a way that we actually can demonstrate is much wider than the way that an adult in a similar situation would explore the hypothesis he goes through seven different high level hypothesis about how the system works in the space of these three minutes and the other thing is that he does experiments so for each of those hypotheses he performs interventions on the World to try to extract data from the world and test it against the hypothesis and those capacities that capacity to look through hypothesis and to intervene on the world to get data are the things that seem to be really characteristic of this Explorer intelligence and they're exactly the things that things like large models do not do right um uh so what we wanted to do was to try and and in many many many experiments that we've done we've shown that children if you give them the right kind of data can learn these kind of over hypothesis about how causal system boards work works but it's actually kind of nice when we had these you know that that um video is maybe 10 years old and we'd see that kind of thing and we'd think that looks like it's really crucial but how could we ever study it in a systematic way and what we've been doing now is trying to see if we can use some of the tools of uh of AI to systematically look at this kind of spontaneous open-ended exploration that we see children uh doing so characteristically um and I'm going to quickly go through four different uh studies that we've done and our basic idea is to set up an online environment that is high-dimensional complex and open-ended and then to let both children and assorted AI agents explore that environment and see what they do and then see if they're exploring the environment in a way that's actually giving them an accurate picture of the environment um this is work with Eliza kosoy and with several um bear students so the first thing that we did was to take that blicket detector that you saw that the child was playing with and put it in an online setting so we made this online causal system the blicket detector is a little box that lights up when you put some things on or did not others and the job as that little boy had was to figure out which ones are blickets how does the machine go and to make the machine go yourself um and the in this we've done tons of experiments but in this experiment The crucial thing is that there are different over hypotheses you could have about the causal structure so in particular it could have a kind of conjunctive structure sorry it could have a disjunctive structure where something is oblate or not if it's oblate it will make it go if it isn't it won't make it go that's kind of our first grown-up assumption about how a system like this would work work but it could also work on this conjunctive principle where you need to have combinations of blickets to make it Go just one won't work but there's some combination that will actually be effective and part of what you have to do in this experiment is to figure this out is both figure out the individual blickets figure out what the individual properties of the objects are but also figure out this General principle about how the machine uh works and what we showed in earlier experiments was that kids were actually better at doing this than adults when there was an unlikely over hypothesis so adults just stick with the obvious thing and keep trying it and the kids can find the less obvious thing so what we wanted to do was to just let children explore and the way we did it was with this virtual online blicket detector and perhaps the most interesting thing is we actually had to build the damn Bic detectors 20 years ago because we couldn't get the kids to deal with screens uh the current generation of kids were perfectly happy to do this kind of exploration on a screen so we set up this online blicket detector and you can actually physically move the bcks around um uh here's an example of a child actually playing with a detector and then what we did was we just said figure out how this works um you can do whatever you want just mess around figure out what figure out how it works um and what we discovered was that this we did this with four-year-olds is that most of the children in the course of about they did usually did about 20 trials that took about 10 minutes 20 different things fully dis uated the causal space so they produced the right evidence to decide whether this was a conjunctive or a disjunctive system and to decide which ones were blicket um and most of them got it right most of them figured out which ones were blickets and which ones weren't e and figured out the over hypothesis which when the machine was conjunctive and when the machine was disjunctive soorry each for each kid the machine in fact as it were the ground truth was either conjunctive or disjunctive but the kids didn't know which um and they showed the same kind of competence when it came to intervention so if it was disjunctive they would just put one on if it was conjunctive they would put two so then the next question was could large language models do this and we actually sort of made it easy for the models because we gave them text information that was the information that the children generated so we didn't even ask the models to generate the information themselves we just gave them the same things that the kids had produced you know a red one goes on and a blue one together and it lights up or the red one goes on doesn't go on and it doesn't light up and so forth and we actually gave them the all of the information all the t uh trials that the kids produced and nevertheless they were not good at solving the problem so then when we asked them is this oblate is that oblate is this oblate at least up to uh um up to gbg3 they were it not doing this accurately and we just tried this again with gbg4 and we found the same uh we found the same result um we also looked at um reinforcement learning agents which are closer to actually having the structure of intelligent agents and we found that indeed they could solve the task but they did it after hundreds of thousands of iterations if you know RL you'll know that that's true and that was partly because even with this incredibly simple causal task there's actually a very very large number of potential combinations and permutations that you could have of which sets of of events led to or didn't lead to the machine lighting up and in fact it turned out that when we looked at the end they these uh the these systems were just matching the data they were overfitting and that's why they were produced they produced so many examples that then they just kind of had a catalog of if you do this then here's what the outcome will be um as opposed to the children um okay so that's one example of a kind of exploratory capacity this capacity to go out do experiments figure out causal structure that four-year-olds are showing tremendous competence at and both large language models and um and uh classic reinforcement learning models are are not even in the ballpark of what the children are doing I wanted to just say one thing about GPT 4 which is I think there's an issue I have absolutely no doubt and someone's probably sitting there and doing this on their laptop as we speak um that if you use the right prompts and you had enough reinforcement from Human feedback you could get the system to do something that looked like this um and with gp4 in particular it's very hard to know how to evaluate it because we have no idea what specific uh reinforcement learning it's getting um and what that means for for what it does I think in a way something like rhf turns up the knob on how much this is an imitation engine because now it's really an engine that's being shaped very specifically by the responses of um of other human beings I don't think RH rhf isn't making the system any more exploratory in fact of anything it's making it more A system that is just responding uh and encoding what the information that other human beings have um and maybe we could talk about that a bit more in the discussion okay so let me give you another example um uh one of the things that I think is important about our our research program is we aren't just sort of saying kids can do this can llms we want to sort of in parallel see what are the capacities that humans have try this out with various kinds of AI agents use that information to do experiment ments with the kids and vice versa um so another question that we had is about categorizing objects based on their causal functions and go back to that point about in cultural Evolution about imitation and Innovation um we know from the cultural Evolution literature that humans are extremely good at imitating what other people do um that's that cultural Niche but of course that wouldn't do you any good unless at some point someone innovated right so if all we did was just imitate what our grandmothers showed us then there wouldn't be any point in imitating because you wouldn't make any progress so cultural Evolution depends on this fine balance between imitation on the one hand and Innovation on the other so the question we wanted to say ask is where do these Innovative capacities come from and we chose a task that involved tool use which is a kind of classic example of this Innovation imitation uh uh parallel so you invent a new tool and it's really useful and then everybody's around around you imitates the tool and that's an important engine of of human progress so what we did was to give kids um examples where they had to sort of figure out a new use for an object that would turn it into a new tool so here's an example you have a tape and scissors and bandage and one job is just figure out what goes with wi which what's highly Associated together and if you have scotch tape everybody says the scissors are highly associated with the Scotch tape if you're a large language model you're going to find lots of sentences that have scotch tape and scissors and many fewer where Scotch tape is associated with Band-Aids but if you raise a causal problem so you say um okay now I've got this torn paper what should I use to fix it um now you don't want the thing that's most associated with the uh the Scotch tape what you want and oh and you don't have any Scotch tape so you explicitly say I have this paper it's torn I don't have any Scotch tape what should I use instead now the Band-Aids are actually the correct uh Choice rather than the scissors um uh so what we did was to have 47 scenarios each with a different set of objects and we asked it multiple times to the llms and to uh children and adults and in each case there was a superficially related object like the scissors um a functional but not related superficially related object and a totally unrelated object and the job of the children and the adults and the models was to say which was the right choice in different kinds of contexts um so what we did was we either asked simply um so you had to evaluate the usefulness of the different kinds of things so we also asked which things go together and when we asked which things go together um all the language models are very good at doing this not quite as good as adults and children but but very good um and GPT 4 is is not significantly different from the children and adults so what we're asking is what goes with what paper does it go more with scissors or does it go more with bandaids but when we asked about the novel use um the large models did much worse and even gp4 didn't do as well as children or adults did and you can see the kind of classic language models that are really just using the statistics of language rather than reinforcement learning from Human feedback are really not doing well on this test on this task at all so this seems to be something that you can't just AB extract from the statistical patterns in the text and the language that you uh that you have or at least it's very difficult to do this um okay so those are examples of things that we children can do examples of exploration and Innovation where the kind of typical uh AI Pro processes and in particular large models are not doing a good job now what I want to do is turn to two new studies that we've done um trying to actually see if we could use the information about what children are doing to design a new RL agent in particular that would do a better job of solving these kinds of tasks um and what we did was again use this idea of just sort of General exploration of a novel environment um so in the first experiment what we did was to try to give ourl agents the same kinds of intrinsic motivations that we see in children um and this is work with yuching du and Lee Diane who's here as well as Eliza kosoy um and this was yuch Ching's um idea I must admit I think my main motivation for this is that actually knowing something about Minecraft gives me some browny points with my grandson um I still still not quite but I've sort of pretended that I actually know what Minecraft is about so what we did was we took a simpler form of Minecraft called crafter and this is a very open-ended environment you find things you use them you make other things you get rewards but the rewards are very much Downstream um and what we did was again just take five to seven year olds tell them play with this the kids are very happy to play with it and then we recorded what they did as they were playing with it um and then we compared that to reinforcement agents that U either just were randomly producing policies or knew the ground truth so that's kind of the other end of the control or who were trained with information gain as a reward so rather than actually trying to get points or get a reward you were the these systems were trained to try and get more information about the environment or else another set that were trained with um uh trying to get more entropy and a third set that we still are just working on that were're trained using empowerment as an intrinsic reward and I won't go into all the details but what we could do is compare the behavior of the kids and the adults to the behavior of these different kinds of reinforcement learning engines and again perhaps not surprisingly we use these different we be meaning yuching and and Lee use these different measures of how much exploration um the various agents and humans did and what we discovered was that the agents including at least some of the children were actually very good at doing a lot of exploration effectively um uh and the agent that had more intrinsic reward did better than the random agent so that was at least a sign that that the adding the intrinsic reward was helping the agents to explore the space effectively although still not as effectively as the uh human adults or children um and it's worth pointing out Lee just sent me this that the adults are taking about adults and children are taking about 20 minutes to do this with about 5,000 moves and the agents are taking a million moves so there's also a bit of a difference um there's also a bit of a difference on that di menion uh the other thing that we found was that when we actually analyzed what the children and adults were doing in terms of Information Gain entropy and empowerment we found that there was a significant correlation between how much what they were doing reflected this kind of reward and how much they actually explored the space effectively so it looked as if among the different children and adults the more they were being driven by Information Gain or by entropy or by empowerment the more effectively they actually explored the space of this open-ended uh the space of this open-ended game um and now let me give me the last uh uh example of a study that we've done so that's one example where we're looking at open-ended exploration and trying to use it as a way of thinking about reinforcement learning agents but also using the reinforcement learning agents as a way of understanding how children can do this kind of uh open-ended exploration so effectively um in this exam in this study what we're doing is looking at a slightly different kind of exploration and this involves um what we call causal curriculum learning so one of the things about humans is that we know when we get a really hard task that in order to solve that hard task we often have to master simpler task to begin with um that's kind of like why you're all here right that's the the rationale for the rationale for uh universities is that if you guys show up and we give you all these simple tasks then you'll be able to go out to the valley and do really hard things um uh one of the things that we know is that giving people a curriculum like this or agents of curriculum like this helps them to solve difficult open-ended problems but of course what you'd really like would be able to generate that curriculum yourself so what you'd really like would be to be able to decide yourself this is the thing that I need to do in order to be able to do this harder thing that I can't do now um so what we did was we used another online environment this is the procgen environment which is essentially a set of different video games and what we did was take kids again sort of five to seven year olds we gave them this difficult task this is a game called leaper and we said you'll get stickers if you solve this and none of the kids could solve it so the kids try this they can't figure out how it works they can't solve it and then we said okay you won't get any stickers but you can decide now which game do you want to play which one do you think you can play that will help you get the stickers in the end and what we discovered was that even five to seveny olds were very systematic about this so we gave them level four which was actually the harder level and then various easier levels and what we discovered um again this is Ana and uh Rosemary and collaborators is that the participants were taking the level of their progress and using that as a cue about what the next level should be that they would choose so if they had not made very much progress then they would choose a simpler level if they were making progress and we could quantify this then they would choose the harder level so they seem to be sensitive to how well am I doing how much progress am I making and used that to determine what the next thing is that they should do in order to ultimately be able to solve the difficult tasks that they couldn't solve to begin with and in fact a large number of them did end up being able to solve the difficult task um uh and then what we did was to actually do the same thing with the agents so now what we did was to actually give the agents um learning progress as a reward as an intrinsic reward so now the agents are getting rewarded not just for solving the task but for how much better they're getting as they try to solve the task and again you know we're still talking about hundreds of thousands of steps for the agents versus you know 10 minutes for the kids but it is still true that giving them that kind of intrinsic reward especially in a context where rewards are sparse greatly improved the way that the agents uh were able to solve this task um okay so let me just conclude the the kind of moral that I want you to take away this is a fantastic Nolla that I highly recommend by Ted Chang which is about thinking about AI growing up um and the moral that I want for this is that this kind of Developmental diversity being able to do different kinds of things having different kinds of intelligences at different times that you can trade off against each other and in particular having a childhood having this period of intense intrinsic motivation to extract information and to to uh to make progress that seems to be one of the things that's really crucial if you're going to go beyond just transmission and cultural technology and have something that really looks like uh at least part of human intelligence okay let me stop there and we have some little [Applause] thanks Alison um maybe I'll I'll ask a quick question before before turning the floor over to others yeah so you know in this um experiment you described about the objects combination of objects lighting things up maybe this is what you were getting at that um when you were comparing humans you know children babies to children to uh to GPT that it was active versus passive learning in the sense that um you know given the transcript of what somebody else has done leaves out their intention and that makes it much harder to to make sense of it yeah I think that's right so you know when the kids are actually doing experiments well one of the next things that we want to do is try and see if suppose we gave took the kids and we just gave them randomly randomly produced data we gave them data from another person s Behavior cloting that's the next experiment um our assumption is they would not do as well as if they were actually generating the hypothesis and generating the experiments um themselves but we don't know whether that's true I the other dimension is just being able to do causal inference from data which is also a challenging as we all know goes beyond us picking out the statistical patterns to sort of say okay this is what the statistical patterns tell you about causal structure I guess also maybe the mind boggles a little bit at thinking about what would the corresponding thing with GP TB if he were trying to make it Active Learning yes right right I mean I have thought about that you know you could imagine a GPT like system that tested out different sentences as hypotheses for example and then you know was trying to get its reinforcement learning um uh humans to give it information about specific hypotheses I don't as far as I know there's nothing that's like that in those C systems so you you've told us how the U uh the children are better scientists and I can understand why on the other hand you have adult scientists who are actually quite good at doing this sort of thing and I get very frustrated I know very many smart people but they don't take risks they don't explore uh so uh and and but otherwise they would really be great scientists so so you see this explore exploit tradeoff very vividly if you're in an institution like science and I think a good way of thinking about science go back to the sort of idea of our Technologies it's a a technology that allows us to take those exploration capacities that we all use when we're kids and actually institutionalize them in a way that lets you you know explore um distant stars or quirks instead of just exploring the like detectors and the things that are in front of you um and your parents um uh I think that's that's EXA actly a way of thinking about it but of course as we all know from being scientists the trick of being a scientist I think more than anything else is getting that Explorer exploit balance right so you can't just explore the way you code when you were two because you have to go out and get grants and do things like that right um uh so you have to be at once this terrible effective exploiter who can pull money out of DARPA uh you know pull resources out um and be an explo who can try lots and lots of different things and I I genuinely think this is a challenge for institutions like science or universities about how you manage to um enable both of those things uh at the same time but it's exact I mean one of my slogans is it's not that children are little scientists it's that scientists are big children which always gets me a round of applause if I'm talking at you know firy lab or some place like that um thank you so much this is such a cool talk um it's very inspiring to an undergrad like me um uh one thing that you were talking about earlier with how uh children only needed like a small number of samples I think you said 20 right um whereas the the models needed like like Millions um would that correspond to like because fundamentally like like you said they're generalizing right would that correspond to like aam's razor or like a similar mechanism yeah it's a good question I mean one questions there are certainly people in cognitive science who've tried to say that the the're really crucial um the really crucial thing is your prior knowledge and it's certainly true that part of the reason why the kids need so much less information is that they kind of have some general ideas about how machines work or about how video games work and they're not simply trying every possible policy so that's certainly part of the reason why they're using fewer steps but it's also true that they're doing this exploration in a very directed way and I think one of the really fascinating challenges um I couldn't resist this I I went through and said no I won't show this last slide um Alvi my husband was saying come on Allison don't add slides now take them away but um uh there's a f fascinating recent paper that came out in nature human behavior from Charlie woa let me see if I can I'm not sure if I can find the slide um where what they did was actually look at um here it is uh look at uh stochastic optimization across development so what they did was let's see if I can find this yeah so here's the paper what they did was actually try and look at both just how much random stuff do you produce how high a temperature is your uh is your behavior is your exploration and how much directed exploration do you produce and what they discovered so this this here is the the red is the random High temperature exploration which is just do you produce more random stuff but the green is actually directed exploration so it's actually exploration that's designed to solve the particular problem that you have and they did a big uh reinforcement learning task and what they discovered which was joy to my heart is that you really could see something like simulated and kneeling where the children really were both higher temperature were doing a higher temperature search than the adults were but also that they were doing this kind of directed exploration and I think the puzzle about how you do directed exploration you know it's easy to see how you could just add noise to the system to make it uh make it more creative make it have more possibilities but how could you get it to do that in a way that is intelligent and sensible and solves the problems you want to solve I think that's a big the biggest kind of Unsolved uh one of the biggest unsolved problems that's uh that's ahead of us and the kids seem to be doing both of those uh both of those things and I'll just I've mentioned this before but you know it's sort of fascinating when I talk to people who are actually working in things like deep learning all of them agree that temperature setting and something like a kneeling is crucial that you can't it doesn't work unless you have some way of manipulating temperature and then I say okay what's the general proof right like what's the general principle about how you should alter this in order to be able to solve problems and they say you fiddle with it until it works and then you fiddle with it some more so it'll work again so I I think again some there's a great opportunity somewhere for someone to actually try and figure out more about how these two kinds of exploration fit together yeah hi thanks so much um super interesting talk really liked it um I had a a question sort of related to the experiments you were showing with the like the bandages and the the scissors and the tape that series of experiments I thought that was really interesting um so uh to me it seems natural that something like gp4 wouldn't do well on this because it's it's it's a problem that's very grounded in like the physical world right um and so um I was curious if you saw examples of similar sorts of behaviors happening for tasks where maybe it wouldn't be so dependent on physical world like some sort of like simly task where you still see issues where gbd4 is not good at kind of um uh taking an idea out of context I guess yeah yeah I mean that's a good that's a good question um uh we thought these were pretty simple examples so we we thought that they that you know they they those should be accessible these are things that are getting described often I mean they're common objects they're in the text uh um when we did it with gp4 v which is the most recent version that has the vision it does better if you give it visual information um if you give it visual information as well the kids can do it just with text so we we wondered about that too we started out giving them illustrations but they can they can give you the right answer just with text but I think that's a really good question and you know people talk about this grounded embodied character of of human intelligence in contrast to what you see with something like uh GPT something that I think is important to say is that even when you're talking about something like gptv or imagenet or the dolly the kind of visual versions of these things they're about pictures they're about pictures that people have decided to put on the internet they're not about going out into a three-dimensional world and moving around and seeing all the random things that you see when you move around in a threedimensional World in fact you know the the internet pictures are really well curated so you don't get the blurry thing that's the thing that you see as your uh it's your visual input as you're as you're going through the world so I think I think it's even my hypothesis would be that if you had something like video data right if you had a very large amount of real life video data where you're work looking around the real world maybe with a bunch of multimodal tactile data then you're getting closer to to to a data source that could work but you're still going to have this passive versus active problem so I think even if you got to see that the fact that you couldn't go out and actually try the experiment would would would limit the kind of generalization the kind of generalization and extrapolation that you can interesting thank you I had a question about um you know these three uh three modes that you spoke about you know explore exploit uh and conver so can you is is is is it possible to um understand the developmental phases of children go through in terms of the trade-offs between these yeah uh and it's really interesting I uh there was another another slide that I uh I cut out um so from an evolutionary perspective it seems as if the story is you start out exploiting then you get then you get animals that will explore they have brains and then you get humans who have a lot of cultural transmission but from the developmental perspective literally from the time they're born babies are imitating so um you know newborn babies will already imitate facial expressions and gestures that they see other people producing um so I think what actually happens with human babies is that they start out having these very strong transmission and exploration intelligences and again newborn babies are already exploring they're exploring by looking around but they're still exploring and interestingly they don't have the exploit capacities so you know uh one of the things I say is children have babies have one utility function to maximize and they're extremely good at maximizing which is be as cute as you possibly can be and all you have to do is just maximize the cuteness utility function and then all your other utilities will be taken care of because um because you have nurturers and caregivers who are designed to do the exploit piece and actually when when I was you I've been thinking about this there's a whole other kind of intelligence which is this intelligence of care of how do you actually take care of another agent so that it can have the opportunity to explore for example something again that all of us faculty face as an issue and problem all the time how do we how do we enable our students to explore without dictating what it is that they should do on the one hand or not providing them with resources on the other hand um I think that I think really the human solution is you have combination of children who are exploring um adults who are providing them with care um and who are exploiting so that they can survive and then seriously Elders grandmothers who are both providing care and providing cultural transmission so you've got you've got children who are designed to explore and then adults who are designed to exploit and then Elders Grandmom's in particular who are designed to teach and care that's kind of kind of the the uh small version but that's a whole other story a whole other story about the Grandmom's but it's that kind of combination of ear having a system where you have an early period where you get to both explore and imitate and then a later period where you actually put that into um operation and then yet another period where what you do is give resources to the new generation of children who are exploring both informational resources and other resources it's that that's part of what I mean about the developmental diversity it's that whole developmental picture that enables humans to be intelligent rather than that um there's a bunch of 35 year-olds in soda Hall who have reached the peak of you know possible intelligence and are out there designing machines that are going to be even more even have even more of this magical intelligence stuff than than they do yeah dendra yeah yeah uh wonderful uh so this explore exploit it's not necessarily tied to the the 3D World though the child normally exhibits it in uh in the 3D 4D world so you know we both believe in embodiment but the question is yeah is embodiment necessary for for this uh kind of exploration for the child the typical environment is is an embodied environment but can we dink the these Concepts can be theoretically delink but are they empirically linked so I think they are empirically linked but you could certainly and I've been thinking about this with llms here's a a lovely statistic which is that which was very Vivid when I was just visiting my grandchildren in Montreal which is that your average three-year-old produces three questions a minute so something like three 00 questions a day that's the empirical that's what the empirical evidence is so asking questions is a really interesting example of using language to do this kind of active learning and you could imagine a three-year-old who isn't embedded who's just a text three-year-old but who goes around asking questions all the time um and asking questions in a way that will enable them to uh to make the right kind of theoretical uh progress that they need and there's beautiful experiments by Henry Wellman that show for instance you know if you have a two or three-year-old who is constantly asking questions it can feel like okay this is just a way of annoying adults um Henry showed that the kinds of questions they ask and their decision about whether to ask the next question depends on how much information they've gotten in the last linguistic term so they're doing this Information Gain reward uh motivation but they're doing it in a linguistic context so if you if you think you've already got enough information then you don't repeat the question you go on to the next question but if you if the mom has just given you an answer that isn't sufficient that you know like um opium has a dormitive property that's that kind of example that just an empty response then you go back and repeat the question until you get something that actually has given you more information so anyway I think those are examples of things that something like question answering is an example of something that you could imagine being this kind of active learning even in um um even in a non uh uh physically embodied agent but I think in practice of course you know most of the time what's happening is that the agents really are physically embodied and the kids are physically embodied and they're picking things up and experimenting with them and trying them and the the a lot of the languages happening in that um a lot of the languag is happening in that context as well interestingly also happening in um pretend play which is something that children are doing when they're two or three years old so you see children which isn't quite embodied pretend is kind of an interesting thing because it's not quite the same thing as being embodied and it's very linguisti
2023-11-21 17:37