AI and the Productivity Paradox
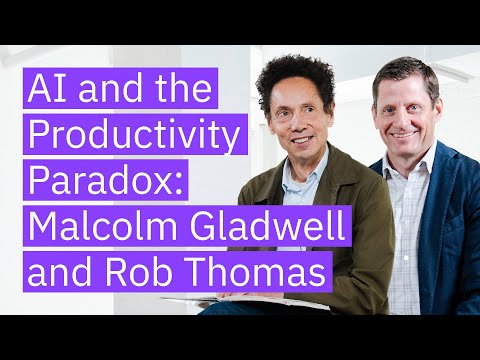
[Applause] [Music] how we doing good Rob this is our our second time we we did one of these in the middle of the P pandemic but not it's all such a blur neither of us can figure out when it was I know it's hard those are like a blurry years you don't know what happened right but um um well it's good to to see you to meet you again um I want I wanted to start by going back you've been in IBM 20 years is that right 25 in July believe it or not so you were you were a kid when you joined I was four so I I want to contrast present day Rob and 25 years ago Rob when you arrive at IBM what do you think your job is going to be at your career is what what do you think the kind of problems you're going to be addressing are well it's kind of surreal because I I joined IBM Consulting and I'm coming out of school and you quickly realize wait the job of a consultant is to tell other companies what to do and I was like I literally know nothing and so you're immediately trying to figure out so how am I going to be relevant given that I know absolutely nothing to advise other companies on what they should be doing and I remember it well like we were sitting in a room when you're a consultant you're waiting for somebody else to find work for you bunch of us sitting in a room and somebody walks in and says we need somebody that knows Vio does anybody know Vio I'd never heard of Vio I don't know if anybody in the room has so everybody's like sitting around looking at their shoes so finally I was like I know it so I raised my hand they're like great we got a project for you next week so I was like all right I have like three days to figure out what Vio is and I hope I can actually figure out how to use it now luckily it wasn't like a programming language I mean it's pretty much a drag and drop capability and so I literally left the office went to a bookstore bought the first three books on Vio I could find spent the whole week in reading the books and showed up and got to work on the project and so it was a bit of a risky move but I think that's kind of you would caution others against doing this well but if you don't take risk you'll never you'll never achieve and so to some extent everybody's making everything up all the time it's like can you learn faster than somebody else MH is what the difference is in almost every part of life and so it was not planned but it was an accident but it kind of forced me to figure out that you're going to have to figure things out you know we we're here to talk about a Ai and I'm curious about the evolution of of your understanding or IBM's understanding of AI at what point in the last 25 years do you begin to think oh this is really going to be at the core of what we think about and work on at this company the um computer scientist John McCarthy he was the he's the person that's credited with coining the phrase artificial intelligence it was like in the 50s and he made an interesting comment he said he said once it works it's no longer called AI MH and that then became it's called like the AI effect which is it seems very difficult very mysterious but once it becomes commonplace it's just no longer what what it is and so if you put that frame on it I think we've always been doing AI at some level I I even think back to when I joined IBM in 99 at that point there was work on rules-based engines analytics all of this was happening so it all depends on how you really Define that term You could argue that you know elements of Statistics probability it's not exactly AI but it certainly feeds into it and so I feel like we've been working on this topic of how do we deliver better insights better automation since IBM was formed if you read about what Thomas Watson Jr did that was all about automating tasks yeah is that AI well probably certainly not by today's definition but it's in the same zip code so from your perspective it feels a lot more like an evolution Than A revolution is that a fair statement yes yeah which I think most great things in techn ology tend to happen that way yeah many of the the revolutions if you will tend to fizzle out but even given that is there I guess what I'm asking is I'm curious about whether there was a a moment in that Evolution when you had to readjust your expectations about what AI was going to be capable of I mean was there you know was there a particular Innovation or a particular problem that was solved that made you think oh this is different than what I thought I would say the moments that caught our attention certainly casprov winning the chess tournament nobody or deep blue beating CASRO I should say nobody really thought that was possible before that and then it was Watson winning Jeopardy these were moments that said maybe there's more here than we even thought was possible and so I I do think there's there's points in time where we realized maybe way more could be done than we had even imagined but I do think it's consistent progress every month and every year versus some simal moment now certainly large language models as of recent have caught everybody's attention because it has a direct consumer application but I would almost think of that as what Netscape was for the for the web browser yeah it brought the internet to everybody but that didn't become the internet per se yeah I have a cousin who worked for IBM for 41 years I saw him this weekend he's in Toronto by the way I said you work for Rob Thomas he went he went like this he goes he said I'm five layers down but so I always whenever I see my cousin I ask him can you tell me again what you do because it's always changing right I this is a function of working at IBM So eventually he just gives up and says you know we're just solving problems that's all we're doing which I sort of loved as a kind of frame and I was curious what's the coolest problem you ever worked on not biggest not most important but the coolest the one that's just like that sort of makes you smile when you think back on it probably when I was in microelectronics because it was a world I had no exposure to I hadn't studied computer science and we were building a lot of high performance semiconductor technology so just chips that do a really great job of processing something or other and we figured out that there was a market in consumer gaming that was starting to happen and we got to the point where we became the chip inside the Nintendo Wii the Microsoft Xbox Sony PlayStation so we basically had the the entire gaming Market running on IBM chips and so you're the every parent basically is pointing at you and saying you're the culprit probably yeah well they would have found it from anybody but was it was the first time I could explain my job to my kids who were quite young at that time like what I did yeah like it was more tangible for them than saying we solve problems or do you know build Solutions like it it would became very tangible for them and I think that's you know a rewarding part of the job is when you can help your family actually understand what you do most people can't do that it's probably easier for you they can they can see the books yeah um but for for some of us in the the businesses to business world it's not always as obvious so that was like one example where the dots really connected yeah the um there were a couple there's a couple let's talk about a little bit of this in the context of of AI I because I love the frame of problem solving as a way of understanding what the function of the technology is so I know that you guys did something did did some work with um I never know how to pronounce it is it Seeva Seeva Seeva with the football club Seeva in Spain tell me about tell me a little bit about that what problem were they trying to solve and why did they call you in every sports franchise is trying to get an advantage right let's just be that clear everybody's how can I use data analytics insights anything that will make us 1% better on the field uh at some point in the future and Sevilla reached out to us because they had seen some of the we've done some work with the Toronto Raptors in the past and others and their thought was maybe there's something we could do they'd heard all about generative AI that heard about large language models and the problem back to your point on solving problems was we want to do a way better job of assessing talent because really the the lifeblood of a sports franchise is can you continue to cultivate Talent can you find talent that others don't find can you see something in somebody that they don't see in themselves or maybe no other team sees in them and we ended up building something with them called Scout advisor which is built on Watson X which basically just ingest tons and tons of data and we like to think of it as finding you know the the needle and the hay stack of you know here's three players that aren't being considered they're not on the top teams today and I think working with them together we found some pretty good insights that's helped them out and how what was intriguing to me was we're not just talking about uh quantitative data we're also talking about qualitative data but that's the puzzle part of the thing thing that fascinates me how does one incorporate qualitative analysis into that sort of so you're feeding in Scout scouting reports and things like that I gotta real think about how much I can actually disclose but if you think about so quantitative is relatively easy yeah every team collects that you know what's the 40 yard dash I don't think they use that term certainly not in Spain uh that's all quantitative qualitative is what's happening off the field mhm it could be diet it could be habits it could be Behavior you can imagine a range of things that would all feed into an athlete's performance yeah and so relationships there's many different aspects and so it's trying to figure out the right blend of quantitative and qualitative that gives you a unique Insight how transparent is that kind of system I mean is it telling you it it's saying pick this guy not this guy but is it telling you why it prefers this guy to this guy is that I think for anything in the realm of AI you have to answer the why question yeah otherwise you fall into the Trap of the you know the proverbial black box and then wait I made this decision I never understood why it didn't work out so you always have to answer why without a doubt M and how is why answered sources of data the reasoning that went into it and so it's basically just tracing back the chain of how you got to the answer and in the case of what we do in Watson X is we have IBM models we also use some other open source models so it would be which model was used what was the data set that was fed into that model how is it making decisions how is it performing is it robust meaning is it reliable in terms of if you feed it to with the same data set do you get the same answer yeah these are all the you know the technical aspects of understanding the why how quickly do you expect um all professional sports franchises to adopt some kind of are they already there if I went out and pulled the general managers of the 100 most valuable sports franchises in the world how many of them would be using some kind of AI system to assist in their efforts uh 120% would meaning that everybody's doing it and some think they're doing way more than they probably actually are so everybody's doing it I think what's weird about sports is everybody's so convinced that what they're doing is unique that they generally speaking don't want to work with the third party to do it because they're afraid that that would expose them but in reality I think most are doing 80 to 90% of the same things uh so but without a doubt everybody's doing it yeah yeah the um the other C that I love was there was one about a a shipping line Trion on the Mississippi River um tell me a little bit about that project what problem were they trying to solve think about the the problem that I would say everybody noticed if you go back to 2020 was things are getting H up held up in ports there was actually an article in the paper this morning kind of tracing the history of what happened in 2020 2021 and why ships were basically sitting at Seas for months at a time and at that stage we just we had a massive throughput issue but moving even beyond the pandemic you can see it now with ships getting through like Panama Canal there's like there's like a narrow window where you can get through and if you don't have your paperwork done you don't have the right approvals you're not going through and it may cost you a day or two and that's a lot of money in the shipping industry and the tricon example it's really just about when you're pulling into a port if you have the right paperwork done you can get Goods off the ship very quickly they ship um a lot of food which by definition since it's not packaged food it's fresh food there is an expiration period and so if it takes them an extra two hours certainly multiple hours or a day they have a massive problem because then you're going to deal with spoilage and so it's going to set you back and what we worked with them on is using assistant that we've built in Watson X called orchestrate which basically is just AI doing digital labor so we can replicate nearly any repetitive task and do that with software instead of humans so as you may imagine shipping industry still has a lot of paperwork that goes on and so being able to take forms that normally would be multiple hours of filling it out this isn't right send it back we've basically built that as a digital skill inside of Watson X orchestrate and so now it's done in minutes now did they real did they realize that they could have that kind of efficiency by teaming up with you or is that something you came to them and said guys you could we can do this way better than you think what's the I'd say it's always it's always both sides coming together at a moment that for some reason makes sense mhm cuz it you could say why didn't this happen like 5 years ago like seems so obvious well technology wasn't quite ready then I would say but they knew they had a need because I forget what the precise number is but you know reduction of spoilage has massive impact on their bottom line M and so they knew they had a need we thought we could solve it and the two together um who did you guys go to them though my point did they come to you I recall that this one was an inbound meaning they had reached out to IBM say we'd like to solve this problem I think it went into one of our digital centers if I if I recall it's a literally phone call yeah but so the the other the reverse is more interesting to me because there seems to be a very very large Universe of people who have problems that could be solved this way and they don't realize it what's your is there a shining example of this of some you just can't you just think could benefit so much and isn't benefiting right now maybe I'll answer it slightly differently I'm I'm surprised by how many people can benefit that you wouldn't even logically think of first let me give you an example there's a franchiser of hair salons M Sport Clips is the name my My Sons used to go there for haircuts because they have like TVs and you can watch so so they loved that they got entertained while they would get their haircut I think the last place that you would think is using AI today would be a franchiser of hair salons yeah but just follow it through the biggest part of how they run their business is can I get people to cut hair and this is a high turnover industry because there's a lot of different places you can work if you want to cut hair people actually get injured cutting hair because you're on your feet all day that type of thing and they're using same technology orchestrate as part of their recruiting process how can they automate a lot of people submitting resumés who they speak to how they qualify them for the position and so reason I give that example is the the opportunity for AI which is unlike other Technologies is truly unlimited it will touch every single business it's not the realm of the Fortune 500 or or the Fortune 1,000 this is the fortune any size and I think that may be one thing that people underestimate about AI yeah what about I mean I was thinking about education as as a kind of I mean education is the perennial uh Whipping Boy for you guys are living the 19th century right I'm just curious about if a if a superintendent of a public school system or the president of a university sat down and had lunch with you and said do the University first my costs are out of control my my uh enrollment is down my students hate me and my board is revolting help how would you s how would you think about helping someone in that situation I spend some time with universities I like to go back and visit Alma moders where I went to school and so I do that every year MH the the challenge I've Hol of universities is there has to be a will yeah and I'm not sure the incentives are quite right today because bringing in new technology let's say we want to go after we can help you figure out student recruiting or how you automate more of your education everybody suddenly feels threatened at a university hold on that's my job I'm the one that decides that or I'm the one that wants to dictate the course so there has to be a will so I think it's very possible and I do think over the next decade you will see some universities that jump all over this and they will move ahead and you see others that do not because it's very possible where how does when you say there has to be a will um is that the kind is that a kind of thing that you that people at IBM think about like when in this conversation you hypothetical conversation you have with the University president would you give advice on on where the will comes from I don't do that as much in a university context I do that every day in a business context because if you can find the right person in a business that wants to focus on growth or the bottom line or how do you create more productivity yes it's going to create a lot of organizational resistance potentially but you can find somebody that will figure out how to push that through I think for universities I think that's also possible I'm not sure there's there's there's a return on investment for us to do that yeah yeah yeah let's let's define some terms um uh AI years a term I told you like to use what does that mean we just started using this term literally in the last three months and it was a it was what we observed in ter which is most technology you build you say all right what's going to happen in year one year 2 year three and it's you know largely by by a calendar AI years are the idea that what used to be a year is now like a week and that is how fast the technology is moving and to give you an example we had one client we're working with they're using one of our Granite models and the results there we getting were not very good accuracy was not there their performance was not there so I was like scratching my head I was like what is going on what business were they in they were Financial Services the bank so I'm scratching my head like what is going on everybody else is getting this and like these results are horrible and I said to the team which version of the model are you using this was in February like we're using the one from October I was like all right now we know precisely the problem because the model from October is effectively useless now since we're here in February are you serious you you actually useless ABS completely useless yeah that is how fast this is changing and so the minute same use case same data you give them the model from late January instead of October the results are off the charts yeah wait so what exactly happened between October and January the model got way better but dig into that like what do you mean by the we are con we have built large compute infrastructure where we're doing model training and to be clear model training is the realm of probably in the world my guess is five to 10 companies MH and so you build a model you're constantly training it you're doing fine-tuning you're doing more training you're adding data every day every hour it gets better and so how does it do that you're feeding it more data you're feeding it more live examples using things like synthetic data at this point which is we're basically creating data to do the training as well all of this feeds into how useful the model is and so using the October model those were the results in October just a fact that's how good it was then but back to the concept of AI years two weeks is a long time and is that are we had a in a steep part of the model learning curve or do you expect this to continue along this at this Pace I think that is the big question and don't have an answer yet by definition at some point you would think it would have to slow down a bit but it's not obvious that that is on the horizon still speeding up yes how fast can it get we've debated can you actually have better results in the afternoon than you did in the morning really it's nuts yeah I know but that's that's why we came up with this term because I think you also have to think of like Concepts that gets people's attention so you you're basically turning into a bakery you're like the bread from yesterday you know you can have it for 25 cents but I mean you you do preferential pricing you could say We'll charge you X for yesterday's model 2 x for today's model I think that's dangerous um as a merchandising strategy but I get your point yeah but that's crazy and this by the way so this model is the same true for all models you're talking specifically about a model that was created to help some aspect of a financial services so is that kind of model accelerating faster and learning faster than other models for other kinds of problems so this domain was code yeah and so by definition if you're feeling feeding in more data so so more code you get those kind of results um it does depend on the model type yeah there's a lot of code in the world and so we can find that we can create it like I said um there's other aspects where there's probably less inputs available which means you probably won't get the same level of iteration yeah but for code that's certainly the cycle times that we're seeing yeah and how do you know that let's stick with this one example of this model you have how do you know that year model is better than big Company B down the street a client asked you why would I go with IBM as opposed to some there's some firm in the valley that says they have a model on this what's your how do you how do you frame your advantage well we Benchmark all of this and I think the most important is metric is price performance not price not performance but the combination of the two and we're super competitive there well for what we just released with what we've done in open source we know that nobody's close to us right now on code now to be clear that will probably change yeah because it's like Lea frog people will jump ahead then we jump back ahead but we're very confident that with everything we've done in the last few months we've taken a huge leap forward here yeah this it's I mean this goes back to the point I was making in the beginning so about the difference between your 20-some self and 99 and yourself today but this time compression is has to be a crazy adjustment so your the concept of what you're working on and how you make decisions internally and things has to undergo this kind of Revolution if you're if you're switching from I mean back in the day a model might be useful for how long years years I think about you know statistical models that sit inside things like SPSS which is a product that a lot of students use around the world I mean those have been the same models for 20 years yeah and they're still very good at what they do and so yes it's a completely it's a completely different moment for how fast this is moving and I think it just raises the bar for everybody whether you're a technology provider like us or you're a bank or an insurance company or or a shipping company to say how do you really change your culture to be way more aggressive than you normally would be does this mean this is a weird question but does this mean a different set of kind of personality or character traits are necessary for a decision maker in Tech now than 25 years ago there's a there's a book I saw recently uh was called The Geek way which talked about how technology companies have started to operate in different ways maybe than many you know traditional companies and more about being data driven more about delegation are you willing to have the smartest person in the room make decision as opposed to the highest paid person in the room I think these are all different aspects that every company is going to face yeah yeah next term talk about open when you use that word open what do you mean I think there's really only one definition of open which is for technology is open source MH and open source means the code is freely available anybody can see it access it contribute to it and what is tell me about why that's an important principle when you take a topic like AI I think it would be really bad for the world if this was in the hands of one or two companies or three or four doesn't matter the number some small number think about like in history sometime early 1900s the Interstate Commerce Commission was created and the whole idea was to protect Farmers from railroads meaning they wanted to allow free trade but they knew that well there's only so many railroad tracks so we need to protect Farmers from the shipping cost that railroads could impose so good idea but over time that got completely overtaken by the railroad Lobby and then they used that to basically just increase prices yeah and it made the lives of farmers way more difficult I think you could play the same analogy through with AI if you allow a handful of companies to have the technology you regulate around the principles of those one or two companies then you've trapped the entire world I think that would be very bad so is there a danger of that happen for sure I mean there's companies in Watson in Washington every week trying to achieve that outcome MH and so the opposite of that is to say it's going to be an open source because nobody can dispute open source yeah because it's right there everybody can see it yeah and so I'm a strong believer that open source will win for AI it has to win it's not just important for business but it's important for humans on the I'm curious about on the sort of list of things you worry about actually let me before let me ask this question very generally what is the list of things you worry about what's your top five business related worries right now top those are your first question we could be here for hours for me to answer I didn't say business related we can leave you know your kids haircuts got it out of the number one is always it's the thing that's probably always been true which is just people MH do we have the right skills are we doing a good job of training our people are our people doing a good job of working with clients like that's number one number two is innovation are we pushing the envelope enough or are we staying ahead number three is which kind of feeds into the Innovation one is risk-taking are we taking enough risk we without risk there is no growth and I think the Trap that every larger company inevitably falls into is is conservatism yeah things are good enough and so it's are we pushing the envelope are we taking enough risk to really have an impact I'd say those are probably the top three that I spend most of my time talk about the last term to Define productivity Paradox something I know you've thought a lot about what does that mean so I started thinking hard about this because all I saw and read every day was Was Fear about AI and I studied economics and so I kind of went back to like basic economics and there's been like a macro investing formula I guess I would say it's been around forever that says growth comes from productivity growth plus population growth plus debt growth so if those three things are working you'll get GDP growth and so then you think about that and you say well debt growth we're probably not going back to 0% interest rates so to some extent there's going to be a ceiling on that and then you look at population growth there are shockingly few countries or places in the world that will see population growth over the next 30 to 50 years in fact most places are not even at replacement rates yeah and so I'm like all right so population growth is not going to be there so that that would mean if you just take it to EXT to the extreme the only chance of continued GDP growth is productivity and the best way to solve productivity is AI so that's why I say it's a paradox on one hand Everybody's scared half to death it's going to take over the world take all of our jobs ruin us but in reality maybe it's the other way which is it's the only thing that can save us yeah and if you believe that economic equation which I think has proven quite true over hundreds of years I do think it's probably the only thing that can save us actually looked at the numbers yesterday for a totally random reason on population growth in Europe I me see this a special bonus question we'll see how smart you are which country in Europe Continental Europe has the highest population growth it's small Continental Europe um probably one of the nordics I would guess close Luxembourg okay something is going on in Luxembourg I feel like we all of us need to investigate they're at 1.49 which in the day by the way would be a relatively that's the best performing country is one I mean in the day you'd be countries had routinely had 2 point something you know percent growth in a in a given year um last question you're writing a book now we were talk chat about it backstage um and now I appreciate the Paradox of this book which is in a universe where the model is better in the afternoon than it is in the morning how do you write a book that's like printed on paper and expect it to be useful this is the challenge and I uh I'm an incredible author of useless books meaning most of what I've spent time on in the last decade is stuff that's completely useless like a year after it's written and so when um we were talking about I was like i' would like to do something around AI That's Timeless yeah that would be useful 10 or 20 years from now but then to your point so how do you how is that even remotely possible if the model's better in the afternoon than in the morning so that's the challenge in front of us but the book is around AI value creation so kind of links to this productivity Paradox and how do you actually get sustained value out of AI out of automation out of data science and so the biggest challenge in front of us is can we make this relevant past the day that it's published how are you setting out to do that I think you have to to some extent level it up to bigger Concepts which is kind of why I go to things like macroeconomics population geography as opposed to going into the the weeds of the technology itself if you write about this is how you get better performance out of a model we can agree that will be completely useless two years from now maybe even two months from now yeah and so it will be less in the technical detail and more of what is sustained value creation for AI which if you think on what is hopefully a 10 or a 20 year period it's probably we're kind of substituting AI for Tech technology now I've realized because I think this has always been true for technology it's just now ai is the thing that everybody wants to talk about um but let's see if we can do it time will tell did you have any inkling that the pace that this AI years phenomenon was going to that things the pace of change was going to accelerate so much because you had Moors law right you had a model yeah in the technology world for this kind of exponential increase in so were you were you thinking about that kind of acceler similar kind of acceleration in the I think anybody that said they expected what we're seeing today is probably exaggerating I think it's way faster than anybody expected yeah but technology back to your point of Mo's law has always accelerated through the years so I wouldn't say it's a shock but it is surprising yeah you've had a kind of extraordinary uh privileged position to watch and participate in this revolution right I mean how many other people have been in that have ridden this wave like you have I I do wonder is is this really that much different or does it feel different just because we're here meaning I do think on one level yes so in the time I've been in IBM mobile happened social Network happened blockchain happened AI so a lot has happened but then you go back and say well but if i' had been here between 1970 and '95 there were a lot of things that are pretty fundamental then to so I wonder almost do we do we always exaggerate the time frame that we're inh I don't know yeah but it's a good idea though I think the ending with the phrase I don't know it's a good idea though it's probably a great way to wrap this up thank you so much thank you Mal [Applause]
2024-07-24 11:06