Advancing AI 2024 @AMD
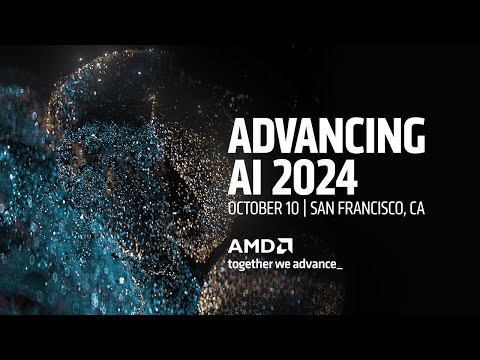
Good morning. How is everyone this morning? All right. Big, big. Welcome to advancing AI 2024. It is so great to be here in San Francisco with all of you.
So many press analysts, customers, partners and lots of developers today. And welcome to everyone who's joining us online from around the world. It's been an incredibly busy year for AMD, with lots of launches across new products in our PC and our embedded portfolio. But today is a special day. Today is all about data center and AI. We have a lot of exciting news and products, and so let's go ahead and get started.
Now at AMD, we believe high performance computing is the fundamental building block for the modern world. And we are really committed to pushing the envelope to help use technology to solve the world's most important challenges. Whether you're talking about the cloud or healthcare or industrial or automotive or comms or PCs and gaming, AMD products today are used by billions of people every day. And for sure, AI is the most exciting application of high performance computing and drives a need for significantly more compute as we go forward. So let's talk a little bit about AI.
Actually, I think we're going to talk a lot about AI today, if that's all right with you guys. Over the next decade AI will enable so many new experiences that will make computing and even more a central part of our lives. If you think about it, AI can help save lives by accelerating medical discoveries. It can revolutionize research. It can create smarter and more efficient cities.
It can enable much more resilient supply chains. And it can really enhance productivity across virtually every single industry. And our goal at AMD is to make AMD the end and AI leader. And to do that, we have four big themes. First, it's about delivering the best high performance, energy efficient compute engines for AI training and inference. And that's including CPUs, GPUs and NPUs.
And you're going to hear me say today there is no one size fits all when it comes to computing. The second is really to create an open, proven and developer friendly software platform. And that's why we're so excited to have so many developers joining us here today.
And it's really about enabling leading AI frameworks and libraries and models so that people can use the technology and really co innovate together. The third piece of AMD strategy. And you're going to see a lot of our partners and customers whether on stage today or you know, throughout the show. It's about co-innovation. Like there's no one company that has every answer. You actually need the entire industry to come together.
And so for us, this partnership is about including the entire ecosystem, including the cloud OEM software, new AI companies. And our goal is to drive an open industry standard AI ecosystem so that everyone can add their innovation on top. And fourth, we also want to provide all the pieces needed for our customers to deliver their total solutions.
And that includes not only at the chip level, but really at the rack, cluster and data center level. So when you put all that together, we are really committed to driving open innovation at scale. And on the silicon side, and you guys know that we spend a lot of time on the hardware. This means driving the bleeding edge of performance in CPUs, GPUs and high performance networking, but all to new industry standards. On the software side, we're going to talk a lot about software today. It's about enabling the industry's highest performance open source AI software stack.
And with our acquisition of ZT systems, we're going to bring all of those elements together to really offer a complete roadmap of AI solutions. So when you look at what business leaders are talking about, you know, when I talk to business leaders today, everyone's focused on number one, how do I use AI as fast as possible? But number two, how do we maximize the impact and ROI for their AI initiatives? Now, when we think about AI, it really is about choosing the right compute for the right application and looking across the portfolio. We see a lot of different pieces, you know, starting first on the CPU side. We have lots of opportunity to really think about, you know, today's AI is really about, you know, CPU capability. And you see that in data analytics and a lot of those types of applications.
So for instance, in enterprise predictive analytics are actually used very often. But you also see in generative AI applications you actually rely on the GPU. So we're starting to see a lot of conversation about agentic AI and these new workloads. And these are the idea that LLMs can actually be tuned to automate very difficult tasks.
And actually reason and help us make decisions by using natural language. So when you look at agentic AI, you actually require significantly more general purpose compute as well as AI compute. So you see CPUs handling things in the data pipelines.
And then you see GPUs for training, fine tuning and inference. So when you look at all of these compute needs, we certainly have the best portfolio in the industry to address end to end AI. So a little bit about our portfolio. We've built our leadership data center compute portfolio over multiple generations, starting with our EPYC CPUs since launching in 2017.
EPYC has become the CPU of choice for the modern data center. The largest cloud providers offer more than 950 EPYC instances and have deployed EPYC widely throughout their infrastructure on their most important services things like office 365, Facebook, Salesforce, SAP, Zoom, Netflix and many more. And on the enterprise side, numerous large customers have also deployed EPYC on prem to power their most important workloads. Today, you're going to hear from all of our server, our largest server OEMs, and they provide over 350 EPYC platforms. If you put all that together, we're very proud to say we exited the second quarter at a record 34% revenue share. On the AI side, we launched MI300 less than a year ago to very strong demand as the large infrastructure providers like Microsoft and Meta deployed MI300 to power their most important AI applications.
Instinct platforms are now available from every major OEM, and numerous cloud providers have also launched public instances, making it easier than ever to use AMD Instinct. Now, customer response has been very, very positive. And you're going to hear from some of those leaders, today, leaders like Cohere, Essential, Luma, Fireworks, Databricks and many others have been able to bring their AI workloads to MI300 very quickly. And you will hear at leadership performance and TCO. So we've got a lot of new news today.
So that includes CPUs, GPUs, DPUs, NICs, and also enterprise Copilot plus PCs. So let's start first with the core of the data center computing the CPU. I've spent a lot of time with CIOs recently, and what everyone is thinking about is how do I modernize the data center? They want a CPU with leadership performance, efficiency, and TCO with Turin. That is exactly what we've delivered. Today, I'm super excited to launch our fifth gen EPYC portfolio. It all starts with our latest Zen five core.
We designed Zen five to be the best in server workloads, and that means delivering an average of 17% higher IPC than Zen four and adding support for full AVX512. Turin is fantastic. It features up to 150 billion transistors across 17 chiplets scales, up to 192 cores and 384 threads. And one of the things that's very special about fifth gen EPYC is we actually thought about it from the architectural standpoint in terms of how do we build the industry's broadest portfolio of CPUs.
That both covers all of the new cloud workloads, as well as all of the important enterprise workloads and things like, you know, building fifth gen EPYC CPU at 5Ghz. That was because there was a new workload. We were seeing the need. When you think about industry leading performance for AI head nodes, that frequency becomes really important. And that's an example of where we've brought in the portfolio with Turin.
Now how do we do that. Turin actually uses our industry leading chiplet technology. This is an area where we've innovated ahead of everybody else in the industry. And that allows us to optimize for both enterprise scale up as well as cloud native, scale out workloads. What we do here is we use a consistent ISA and socket, which is really helpful for developers as well as our customers, and we maintain feature parity, including support for next gen memory and IO.
We've also extended EPYC's confidential compute capabilities with the addition of trusted IO that enables Turin to communicate securely with GPUs, NICs, and storage. All right, I'm going to show you some pretty chips here. Here we have Turin. Now, this version of Turin is actually 128 cores, and it's optimized for scale up workloads. It has 16 four nanometer chiplets. So if you look outside of the arm of the ring, you see the 16 four nanometer chiplets and a six nanometer IO die in the center.
And we've optimized this for the highest performance per core because it's extremely important in enterprise workloads. When you see that, you know, software is often licensed on a per core basis, you want the highest possible performance per core. Okay. Now this is also Turin.
This is the 192 core version of Turin. And this guy is optimized for scale out workloads. And here we use 12 three nanometer compute triplets. And it's the same six nanometer IO die in the center.
And this version of Turin is really optimized for cloud. So applications that benefit from maximum compute per socket. This is what we do. Thank you very much. So with these two configurations, our fifth gen EPYC portfolio is the broadest portfolio in the industry. It scales down to eight cores at extremely high performance per core to up to 192 cores with extremely high performance per socket.
And you can go across a wide range of TDPs. And what that does is it just enables customers to choose the best operating point for their specific needs. Now, Turin is great, but I also want to remind everyone it actually builds on our strong track record of execution. We've built a fifth gen EPYC CPU that runs at 5GHz, delivering industry leading performance for AI head nodes and many applications, but you also see that we've gotten up to highest core count 11X performance. So let's now take a look at Turin performance. We're going to compare many of the things in the next few slides to the competition's top of stack, Emerald Rapids.
When you look at the competition's top of stack, a dual socket fourth gen EPYC server is already 1.7 times faster on Specint Rate 2017, and with fifth gen EPYC, the performance is fantastic. We extend that lead with 2.7 times more performance. Now we know it's a very competitive space, and we fully expect as our competition launches their next generation CPUs and they ship in volume, that Turin will continue to be the leader for the enterprise space. There are many commercial software stacks that are licenses for core, and CIOs want to optimize the cost by running solutions on the fewest possible cores when running these workloads on prem. Again, fourth gen EPYC is already the performance leader, and with fifth gen, we deliver 1.6 times
more performance per core than the competition. That's 60% more performance with no additional licensing cost. Now let's move to relational databases. Now these are also critical workloads for things like transaction processing and analytics. And MySQL is widely deployed in the enterprise in the cloud. This is another area where EPYC already offers leadership.
And now with Turin, that performance capability increases to delivering 3.9 times more than the competition in video transcoding. You can also see again, the fifth gen EPYC significantly extends our lead, and we are now four times faster than the competition. Supercomputing is also another one of those really important workloads, and it has been a place where EPYC has continued to lead.
We're already the world's fastest CPU for complex modeling and simulation software. And with Turin, we extend that lead. Because of the Zen five, IPC increases as well as our full implementation of AVX512. And so we now deliver 3.9 times more performance than the competition. And what that means is that for researchers who are really doing the most difficult simulations in the world, they get to their answers much faster. If they're using AMD.
Now enterprises are also running many, many more of their applications, their AI applications on their CPU. And this is another area where Turin delivers leadership three times faster performance on traditional machine learning, and 3.8 times better performance on TCPx-AI. Which is actually an aggregate benchmark that represents end to end enterprise AI workloads. So when you put all this together, I want to give you the business reason why people are so excited about Turin.
According to IDC, nearly 75% of enterprise customers refresh their server infrastructure every 3 to 5 years. Now, if you look at a typical data center today that you know that an enterprise may have, it might be running something like 1000 Cascade Lake servers. That was the top of the stack in the industry from four years ago.
Fifth gen EPYC can do that same amount of work of 1000 servers in just 131 Turin servers. Now just think about what that means. It's like a huge benefit for CIOs. You can replace seven legacy servers with one single EPYC server, and that significantly reduces the power that you need in your data center.
It lowers TCO by more than 60%. And when you add on top of that, the enterprise software licensing costs, that means an enterprise can break even on their investments in as little as 6 to 12 months. So that's good for CIOs and it's also really good for CFOs who want to optimize their CapEx spend. And on top of that, it also creates space for all of the additional compute that you need, whether you're talking about AI capacity or just adding more general purpose compute capacity.
So that's the benefit of the new technology. Now, I really love talking about our technology, but I love more having our partners talk about our technology. So to understand how EPYC delivers the most in the most demanding environments, let's welcome our first guest, a very close AMD partner who runs some of the world's largest and most advanced data centers.
Please welcome Amin Vahdat from Google Cloud. Hello, Amin. It's a pleasure Lisa.
It is so great to have you here. I'm so excited. You know, Google was, really one of the first to adopt AMD at scale.
And we have learned so much from our partnership with you. So can you tell us a little bit about our work together and, all that you're doing in this gen-AI era. It's my real pleasure, Lisa. Thank you for inviting me to join you. Our partnership with AMD goes back a long way. We've been using EPYC CPUs to power our general purpose instances in addition to our high performance and confidential computing solutions.
Just last year, we launched C3D, Google Cloud's fourth gen, EPYC based offering for general purpose workloads like web servers, databases, and analytics. In this new era, C3D is a compelling option for many AI workloads and frankly, the demand for ML compute power is insatiable. I see the rise of generative AI as truly the biggest transformation since the beginning of the internet. We're on the cusp of a change that will redefine industries, amplify human potential, and create unprecedented opportunities. To me, that's what makes partnerships like ours.
So incredibly important. Thank you. We need to constantly evolve our hardware and software architectures to respond to the demand we are seeing from our customers, whether it's raw performance, cost, convenience, or energy efficiency. Lisa, I really look at this. When I think about the work that you're doing, Amin, as you know, pretty amazing. And I know look, beyond AI, we're also doing a lot of work on your internal workloads as well as some of the third party workloads on EPYC. So can you just share a little bit more about what you're seeing and what customers are seeing? Absolutely.
We've been using EPYC CPUs for multiple generations to serve our cloud customers and our internal properties at Google. That adoption has been driven in large part by the gen over gen performance and efficiency gains. We've delivered together.
For example, Snap used EPYC based virtual machines on Google Cloud to reduce their AI inferencing costs by 40% and improve their performance by 15%. So those are some big numbers. 13 months after the introduction of C3D, KeyBank, one of the largest banks in the US, is seeing cost efficiencies modernizing to C3D. Striveworks and Neural Magic are running inference workloads on CPUs, saving money without sacrificing speed. And that's just to name a few thanks to our collaboration. C3D has been one of our most successful VM instance launches to date, with up to 45% faster performance than previous generations.
I, I love hearing those numbers, Amin, it's wonderful to see how great C3D is doing now. Google's, totally leading in AI innovation, and I know you're doing so much. Can you talk a little bit more about, you know, sort of your vision for what's happening in AI and the role EPYC plays? It's going to take innovation on every single front. Releasing new Gemini models, software, CPUs, GPUs and TPUs.
But it's bigger than that. We're rethinking our system and infrastructure designs from the ground up. That's where our AI hyper computer comes in.
It's a supercomputing architecture that's designed to combine performance optimized hardware, open software, leading ML frameworks, and flexible consumption models to maximize the return on AI investments. EPYC CPUs are an important part of that stack, offering a cost effective and seamless option for AI workloads. Sustainability is also a key part of Google's strategy during this transformation, and so I really appreciate AMD's focus on delivering excellent performance per watt and increasing critical metric for all of us.
That's wonderful. We totally agree, Amin, and, you know, it's great to see all the innovation that you're bringing to the market now. We're super excited about launching Turin today.
And it's another area where, frankly, our teams have been partnering closely and you've given us just fantastic feedback. Can you tell us a little bit about your plans? Thank you, Lisa. Turin is a beautiful chip and, we're really looking forward to the continued collaboration.
It was a pleasure joining you today. And, congratulations on all the success. We're looking forward to partnering with you to deliver Turin based VMs early next year.
That's fantastic. Wonderful. Thank you so much, Amin. Super exciting. Now let's turn to data center GPUs and our next generation Instinct accelerators. Last December, we estimated the data center AI accelerator market would grow from 45 billion in 2023 to more than 400 billion in 2027.
And at the time, I remember people asking me, that seems like a really big number. Is that true? Since then, I demand has actually continue to take off and actually exceed expectations. It's clear that the rate of investment is continuing to grow everywhere, driven by more powerful models, larger models, new use cases, and actually just wider adoption of AI use cases. So now, as we look out over the next four years, we now expect the data center AI accelerator TAM will grow at more than 60% annually to $500 billion in 2028. For AMD, this represents just a huge growth opportunity.
We launched our MI300 family last December, and we had leadership performance and very strong customer demand. And I'm very happy to say that the ramp has gone just extremely well. In the last ten months, we've been laser focused on ensuring our customers get up and running as fast as possible with maximum performance right out of the box. And to do that, we've had to significantly drive improvements and continuous improvements across our ROCm software stack. We've integrated new features, we've optimized new libraries, we've enabled new frameworks, and we've significantly expanded the third party ecosystem that supports ROCm.
As a result, if you look today at MI300X performance, we have more than doubled our inferencing performance and significantly improved our trading performance on the most popular models. Today, over 1 million models run seamlessly out of the box on instinct, and that's more than three times the number when we launched in December. And we recently also completed the acquisition of Silo AI, which adds a world class team with tremendous experience training and optimizing LLMS and also delivering customer specific AI solutions. Again, to help our cloud and enterprise customers get to market as fast as possible. The improvements we've made with ROCm are enabling our customers to see great performance with MI300. Now, let me just show you a little bit about what customers are seeing.
We've now worked with many customers across a wide range of workloads, and our experience has shown that we run out of the box actually very well. So most things run quite well, but with just a little bit of tuning. And MI300X consistently outperforms the competition, which is H100 in inferencing. So for example, using Llama 3.1 405B, which is one of the most newest
and demanding models out there, and MI300 outperforms H100 with the latest optimizations by up to 30% across a wide variety of use cases. And we've seen this across a lot of different customer workloads, using many different models, including Mistral, Stable Diffusion, and many others. So this allows our customers to really leverage the performance advantage to build their own leadership AI solutions.
And you're going to hear some of those key use cases a little bit later on. Now we're always pushing the limits on performance. So let me show you what's next. Thank you. Today I'm very excited to launch MI325x, our next Generation Instinct Accelerator with leadership generative AI performance. MI325 again leads the industry with 256GB of ultrafast HBM 3D memory and six terabytes per second of bandwidth.
When you look at MI325, we offer 1.8 times more memory, 1.3 times more memory bandwidth, 1.3 times more AI performance in both FP16 and FP8 compared to the competition. And when you look at that across some of the key models we're delivering between 20 and 40% better inference performance and latency on things like Llama,, Mistral and Mixtral.
And importantly, one of the things that we wanted to do was really keep a common infrastructure. So MI25 leverages the same industry standard OCP compliant platform design that we used on MI300. And what it does is it makes it very easy for our customers and partners to bring solutions to market.
Now, when you look at the overall platform with 8 GPUs, we deliver significantly more AI compute and memory as well. The 8 GPU version features two terabytes of HBM 3D memory and 48TB per second of aggregate memory bandwidth, enabling our customers to run more models as well as larger models on a single MI25 server. When you put all of that capability together, what you see is that MI25 platform delivers up to 40% more inferencing performance than the H200 on Llama 3.1. And a lot of people also are doing training. And when you look at training performance, we've made very significant progress on optimizing our software stack for training on a growing number of customers. And what you see with MI325, we have excellent training performance.
That's very competitive compared to the competition. So as you can see, we're very excited about MI325. The customer and partner industry has interest has been fantastic.
We are engaged across all of the leading OEMs and ODMs. We're on track to ship production later this quarter with widespread system availability from Dell, HPE, Lenovo, Supermicro, and many other providers, starting in Q1. All right, now, let me invite my next guest to the stage. Oracle Cloud is one of our most strategic cloud partners. They've deployed AMD everywhere including CPUs, GPUs, DPUs across their infrastructure.
And to talk more about our work together, please welcome Senior Vice President, Oracle Cloud Infrastructure Karen Batta. Karan, so wonderful to see you again. Thank you for being such a great partner. And actually you were also at our December event.
So, you know, thank you. You talked a lot about how OCI is adopting EPYC across your platforms and services. Tell us a little bit about what's been happening.
Yeah. Again thank you. Thank you for inviting us again. It's very exciting to be here. You know, since last December, you know, AMD and Oracle have been working together for a very long time since the inception of OCI in 2016. You know, AMD EPYC on our deployed across 162 data centers across the globe that covers our public cloud regions or gov regions or security regions, even our dedicated regions and alloy as well. And we've had tremendous success on our compute platform offering bare metal instances, virtual machines on Genoa-based E5 instances.
And then also we also use, you know, at the base layer of our platform, we also use Pensando DPUs so that we can offload that, that logic. So we can give customers the ability to, to get great performance, instances. Look, we love the work that we do together. And, you know, it's not just about the technology, but it's also about what we're doing, you know, with customers. I know that, you know, you're very active with Turin, you know, can you talk to us a little bit about some of that? Absolutely. You know, one of our largest customers today, today, cloud native customers is Uber.
They're using E5, you know, instances today to actually get a lot of performance efficiency. And they've moved almost all of their trips serving infrastructure on top of AMD running on OCI compute. So that's been incredible. That's pretty good. We also have Redbull powertrains that's developing the next generation of F1 engines for the upcoming seasons. And then additionally on top of that, you know we have our database franchise, you know, which is now powered by AMD CPUs. And customers like PayPal and Banco do Brazil are using Exadata powered by AMD to achieve great things for their database portfolio.
So that's been incredible. You know, we are super proud of the work we're doing together on Exadata. I think that's just an example of how, you know, the partnership has grown over time. So look, the momentum with customers is wonderful.
We love the work that we're doing on compute. There's just a little bit of something called AI right now. So let's switch gears to talk a little bit about AI. You just recently launched our, your, MI300 instances publicly. Can you talk a little bit about that? Yeah.
It's been it's been a great collaboration between the two teams we recently made generally available MI300X. We've had incredible reception internally. Externally. And we're working with customers like, you know, Databricks and Fireworks and Luma AI to run incredible inferencing workloads on top of the AMD GPUs. Additionally, on top, we've seen incredible levels of performance for inference, for running things like Llama 3.1 45B. And so we're seeing great efficiency and performance on top of AMD GPUs.
And we're incredibly excited about the roadmap that you've announced, and we're excited to work together on the future of that roadmap. Yeah. Look, it's, it's really, really cool to see what customers are doing. You know, you talk a little bit about the roadmap.
You talk about the importance of, you know, partnership. So, so what's next on the horizon? I mean, first and foremost, we're very excited about Turin. We're going to be working together to launch E6 instances on OCI compute later, next year. So very excited about that.
So that's our compute family will continue to collaborate on the GPU scale up to capacity for MI300X for our customers across the globe, across all types of regions. And then again, we will continue to collaborate on the DPU architecture as well with you guys. That's fantastic. Karan, thank you so much and thank you for the partnership. Congratulations. Thank you. Thank you. That's great to hear.
Now, let me bring another partner to the stage now to talk about, you know, our partnership for maybe a different angle for more of a user angle. Please join me in welcoming Naveen Rao from Databricks to the stage. How are you, Naveen? Great.
Thank you so much for, spending some time with us today. You know, it's, it's been a pleasure working with you and your team. I'd love for you to share just a little bit, you know, about Databricks and what you guys do. Yeah, absolutely.
We're pioneering what we call a data intelligence platform, which combines the best elements of data lakes and data warehouses. The platform enables organizations to unify their data analytics and AI workloads on a single platform. A crucial aspect of our mission is to democratize data and AI. And this democratization is driving innovation across sectors, enabling companies to make data driven decisions and create AI powered solutions. Our team at Mosaic, which is now a part of Databricks, has been pushing the boundaries of what's possible with AI. And we're not just developing models.
We're actually creating entire ecosystems that make AI more accessible, efficient, and powerful for businesses across various industries. Look, I think you guys are doing amazing work. We completely agree that, you know, our goal is to make AI as broadly accessible as possible.
And that's why, you know, partnerships are so important. You've also been very active in using our technology. Can you share a little bit about MI300 and what you've been seeing? Yeah, we've been on this journey for a little while with you, and our collaboration, has been exceptional. We've achieved remarkable results, particularly with large language models.
The large memory capacity and incredible compute capabilities of MI300X have been key to achieve over 50% increase in performance on some of our critical workloads. That's a pretty good number for us. We'll take it. And and that includes things like Llama and other proprietary models that we're working on.
MI30 GPUs are proving to be a powerhouse in the AI computation and efficiency, area. And we're excited about the possibilities that opens up for our customers going forward. Yeah. Well, Thank you. Naveen, look, it's great to hear about, the performance on MI300. Now. You've also been very active in giving us feedback on the ROCm stack, and we so appreciate it.
You know, ROCm plays such an important role in helping, you know, people use, MI300 and our my roadmap. You have a lot of AI expertise as well. Can you talk a little bit about ROCm and what you've seen? Absolutely. Yeah. We've been working with ROCm since, the Mosaic ML days, which was, you know, even, late 2022, I believe. On instinct MI250. And it published those results on the ease of transition from other platforms using ROCm and even scaling across many GPUs to observe very closely that ROCm is capabilities have expanded significantly in the last year.
It now supports a wide range of features and functions for AI workloads, and the performance improvements have been substantial. Many of our models and workflows that were originally developed for other environments can now run seamlessly on AMD hardware, with no modification. Working with AMD, the AMD team has been an absolute pleasure. Together, we're optimizing at multiple levels on the software stack and for AMD GPUs, which translates to better performance and efficiency for our customers. I liked what you said. No modification.
Did you say that? I did, you can say that again. No modification. We are actually thrilled with what our teams have been able to accomplish. I mean, we love working with teams like yours because, you know, it's like a very, very fast iteration and innovation cycle. What's next on the horizon? Yeah, I'm incredibly excited about the future, actually. We've done a we've done a lot with MI300, but that's really just the beginning.
We're looking forward to the continued optimization efforts, not just for the 300X, but also for the MI325X and, upcoming MI350 series. And we're excited by the compute and memory uplifts we're seeing with these products. Especially new things like FP4 for FP6 data types with MI350.
So on the Databricks side, we're working on new models and techniques that will take full advantage of these hardware advancements, to further improve training and inference efficiency and making advanced AI capabilities more accessible to more organizations. The combination of AMD's cutting edge hardware and software innovations is helping to democratize AI and make it more powerful, efficient, and accessible. So we're not just pushing the boundaries of what's possible with AI. We're working to ensure that these advancements are practically and responsibly applied to solve real world problems.
That's fantastic. Look. Thank you so much again for joining us today. Thank you for the partnership, and we look forward to seeing all the great things you and your team are going to be doing.
Thank you. Thank you. So that gives you a little bit of a flavor of how users are seeing, our Instinct roadmap and our ROCm software stack. Now let's turn to the roadmap. As we announced in June.
We have accelerated and expanded our roadmap to deliver an annual cadence of Instinct GPUs. Today, I'm very excited to give you a preview of our next generation MI350 series. The MI350 series introduces our new CDNA for architecture. It features up to 288GB of HBM 3D memory, and adds support for new FP4 and FP6 data types. And again, what we're thinking about is how can we get this technology to market the fastest? It actually also drops into the same infrastructure as MI300 and MI325, and brings the biggest generational leap in AI performance in our history.
When it launches in the second half of 2025. Looking at the performance, CDNA 4 delivers over seven times more AI compute and, as we said, increases both memory capacity and memory bandwidth. And we've actually designed it for higher efficiency, reducing things like networking overhead so that we can increase overall system performance.
In total, CDNA 4 will deliver a significant 35 times generational increase in AI performance compared to CDNA 3. We continue our memory capacity and bandwidth leadership. We deliver more AI flops across multiple data types. When you look at the MI350 series, the first product in that series is called MI355X, and it delivers 80% more FP16 and FP8 performance and 9.2 petaflops of compute for FP6 and FP4. And when you look at the overall roadmap, we are absolutely committed to continue pushing the envelope.
And we are already deep in development on our MI400 series, which is based on our next CDNA architecture that is planned for 2026. Now, Microsoft has been one of our deepest and most strategic partners across our business, and it's played an incredibly important role in shaping our roadmap. I recently sat down with chairman and CEO Satya Nadella to talk about our collaboration.
Let's take a look. Satya, thank you so much for being here. And thank you for being part of our Advancing AI event today. No, thank you so much, Lisa. It's a real honor and pleasure to be with you. So Satya AI is transforming our industry and Microsoft has truly been leading the way.
You know, can you just tell us a little bit about where are we in the AI cycle? What are you most excited about? Where are you seeing the adoption now? First of all, it's always exciting, Lisa, for both of us and all of the folks, when there's a new platform being born. Right. Because in some sense, I like to say this is probably, a golden age again, for systems, right? Because of all the innovation you're doing, the system software innovation, and of course, the application innovation in AI. And behind it are these things that all of us now call the scaling laws.
It's very much like Moore's Law. Now you have these scaling laws that are really creating, I would say, abundance, of compute power. And in fact, it's interesting to think about it. Right? It's the combination of silicon innovations, system software innovation, algorithm innovation, and even sort of good ways to sort of synthesize data are leading to perhaps 100X improvements, for every 10x, increase in compute power. So there's clearly something afoot.
It's a super exciting thing. And it's no longer I mean, I've never seen Lisa this rate of diffusion, ever before. Right? Which is, you know, having lived through both PC, client server, web, internet, mobile, cloud, I would say one, it builds on all of those previous things. And so therefore the rate of diffusion of this throughout the world is pretty exciting to see. Yeah. No, I think you're absolutely right, Satya. It's been incredible.
The amount of innovation that's happening in the industry. And frankly, it's been incredible, the amount of industry that the amount that Microsoft has brought to the industry, in terms of getting, you know, AI innovation out there. I know that we're personally using many of your AI tools, at AMD. We are so excited about the partnership that we have together.
You know, we've been longstanding partners across all aspects of our business and your business. Can you talk a little bit about the partnership and especially, you know, our work in data center and AI infrastructure has been really accelerating. Absolutely. I mean, to your point about the longstanding partnership, there's not a part of Microsoft that we're not partnered with you. Right. When I look back and think about it, right, we're inventing a completely new PC category with you, yet again, we historically always worked with you when it comes to our gaming consoles.
We started work. In fact, you and I first started working together. When both of us were not CEO when we started, you know, really doing the cloud work.
And so we made progress. And then in the last four years, in fact, it feels like, you know, it's been four years since we even started on really adopting your AI innovation for our AI cloud, as I think of it. And I think, you know, the interesting thing that is not only the silicon pieces that you brought, but even the software work that you have done. How? And because at some level, it's that close feedback loop between emerging workloads, right? In this case, these emerging workloads, which are these training and inference workloads, are unlike anything we've seen in the past. Right. These are synchronous data, parallel workloads, that require a, a very different way to think about the software stack and the silicon stack and the jointly optimizing it here.
We now have in our fleet MI300. It's sort of, you know, I mean, we did all the work to even benchmark the latest GPT models. We are seeing some fantastic results. We have customers coming now have choice in the fleet to be able to sort of really optimize for different, you know, for different considerations. People have latency, COGS, performance.
So it's fantastic to see the progress the two teams have made. And I know it's been a lot of hard work, and that's what it takes, which is to be able to sort of see the new workload and optimize every layer of the stack. Yeah, absolutely. So first of all, I have to say we are so proud of the work that we've done together.
On MI300, getting it into Azure, it was absolutely hard work, as you said, but huge thank you to, you know, your engineering teams, hardware and software. I know that, you know, our teams couldn't be closer and how we really brought that together. So let's talk a little bit about the future.
I mean, the thing about AI is, you know, I always say we're just at the beginning of what we can imagine with AI, and it requires an incredible data center infrastructure, a vision for that. And really optimization on all levels. I think one of the things that's most unique about our partnership is, you know, we've talked about bringing the best of, each other to really form that, you know, vertical, you know, vertically integrated stack. So can you talk a little bit about, the roadmap? You know, we're excited at this event. We're actually talking about our accelerated roadmap with MI350, coming next year and MI400 series coming in 2026.
Much of that has been work that we've done together. So, yeah. Can you talk a little? You know, first of all, we're very excited about your roadmap. Because at the end of the day, if I sort of get back to the core, what we have to deliver is performance per dollar per watt. Because I think that's the constraint, right? Which is if you really want to create abundance, right. The cost per million tokens keeps coming down so that people can really go use what is essentially a commodity input to create higher value output. Right? Because ultimately we will all be tested by one thing and one thing alone, which is that world GDP growth being inflected up because of all this innovation.
And in order to make that happen, we have to just be mindful of the one metric that matters, right? Which is this, performance per dollar per watt. And in that context, I think there is so many parameters, right, which we think about what you are all doing. You know, there's what's the accelerator look like for all of this? What's its memory, access bandwidth. How should we think about the network? Right. So that's a hardware and a systems software, problem. So that's something that collaborating together to create, I think the next set of breakthroughs which create, you know, for every ten, we actually get 100X benefit, that I think is the goal.
And I mean, very excited to see how the teams are coming together. But it's OpenAI, Microsoft, AMD, all working together and saying, how can we accelerate the benefits such that this can diffuse even faster than what we have? So I we are looking forward to your roadmap in 350 and then the next generation after that. And the good news here is, the, the, the overall change has already started. And we build on it. And the fact that now all of our workloads will get continuously optimized around some of your innovation, that's the feedback loop that we've been waiting for.
Thank you. Satya. You know, we are so proud of the deep partnership we have built with Microsoft.
And as you heard from Satya, we see even larger opportunities ahead to jointly optimize our hardware and software roadmaps. Now Meta is another very strategic partner who we are collaborating with across CPUs, GPUs and the broad AI ecosystem. They've developed EPYC and they've, used EPYC and Instinct broadly across their compute infrastructure and share our view that open standards are extremely important.
To hear more about that, please welcome Meta VP of Infrastructure and Engineering Kevin Salvadori to the stage. Hello. Hello. Hi, Lisa. Kevin, thank you so much for joining us today.
Thank you for the incredible partnership we built. We are actually so honored to be part of Meta's infrastructure. Can you tell us a little bit about our partnership and how that's evolved? Sure.
Well, we first started partnering together back in 2019. But things really took off in 2020 when we started to design in the Milan CPU, into our server fleet to support our planet level infrastructure apps. So supporting Instagram, Messenger, Facebook, WhatsApp, that's when it really kicked off.
Subsequently, our collaboration, of advanced compute infrastructure has enabled the OS to scale our AI deployments, really meeting with the seemingly insatiable demand for AI services. And Genoa and Turin have been essential for us to optimize our workloads, and we're really excited to now be pairing AMD's compute with AMD's MI accelerators to really help us innovate at scale. So we really see our partnership with AMD as essential for us to scale AI going forward.
No, it's absolutely fantastic. Kevin. You know, thank you. I think one of the things that I've been, you know, super excited about is with each generation of technology, Meta's expanded your deployments. So can you talk a little bit about, you know, sort of what drove those decisions and where we are today? Sure, sure. I can you were right at with every EPYC generation, we've continued to expand our deployments.
And when you serve over 3 billion people every day, which is what we do, performance, reliability and TCO matter. And, you know, we're a demanding customer, but simply just a little demanding, just a little demanding. But simply put, you know, you and your team at AMD have continued to deliver for us.
So, you know, last year we announced Meta's at scale refresh with Bergamo driven by a two and a half times performance uplift, higher rack density and energy efficiency. And that all drove a better TCO for us. And I'm happy to announce to everybody something you already know that we've deployed over 1.5 million EPYC CPUs and Meta's global server fleet. I'd like the sound of that. That's something we've called, you know, at scale deployment.
That's serious scale. Look, we are also, like, so excited about our AI work together. One of the things I've been incredibly impressed by is just how fast you've adopted and ramped MI300 for your production workloads. Can you tell us more about how you're using MI300? I can.
So, as you know, we like to move fast at Meta and the deep collaboration with between our teams from top to bottom, combined with really rigorous optimization of our workloads, has enabled us to get MI300 qualified and deployed into production very, very quickly. And the collective team works to go through whatever challenges came up along the way. It's just been amazing to see how the teams worked really well together, and MI300X in production has been really instrumental in helping us scale our AI infrastructure, particularly powering inference with very high efficiency. And as you know, we're super excited about Llama and its growth.
You know, particularly in July when we launched Llama 405B, be the first Frontier-level open source AI model with 405 billion parameters and all Meta live traffic has been served using MI300X exclusively to do it. It's large memory capacity and TCO advantage. You. It's I mean, it's been a great partnership. And, you know, based on that success, we're continuing to find new areas where Instinct can offer competitive TCO for us. So we're already working on several training workloads. And what we love is culturally, we're really aligned around, you know, from a software perspective around PyTorch, Triton and our Llama models, which has been really key for our engineers to land the products and services we want in production quickly.
And it's just been great to see. You know, I, I really have to say, Kevin, when I think about, you know, Meta I mean, you know, we do so much on the day to day trying to ensure that the infrastructure is good. But one of the things I like to say is, you guys are really good at providing feedback, and I think we're pretty good at maybe listening to some of that feedback.
But look, we're talking about roadmap today. Meta's had substantial input, to our Instant roadmap. And I think that's so necessary when you're talking about all of the innovation on hardware and software. You know, can you share a little bit about that work? Sure, sure. Well, the problems we're trying to solve as we scale and develop these new AI experiences, they're really difficult problems to solve.
And it only makes sense for us to work together on what those problems are and kind of align on, you know, what you can build into future products. And what we love is we're doing that across the full stack. You know, from silicon to systems and hardware to software to applications, from top to bottom. And we've really appreciated the deep engagement of your team and you guys do listen. And we love that. And what that means is we're pretty excited.
The Instinct roadmaps can address more and more use cases and really continue to enhance performance and efficiency as we go forward and scale. And we're already collaborating together on MI350 and then MI400 series platforms. And we think that's ultimately going to be to AMD building better products. And for Meta, it helps us continue to deliver industry leading AI experiences for the world.
So we're really excited about that. Kevin, thank you so much for your partnership. Thank you to your teams for all the hard work that we're doing together. And, we look forward to doing a lot more together in the future. Yeah, thank you Lisa. Thank you.
Thank you. All right. Wonderful. Look, I hope you've heard a little bit from, you know, our customers and partners as to, you know, how we really like to bring co-innovation together, because, yes, it's about our roadmap. But it's also about, you know, how we work together to really optimize across the stack.
So as important as hardware is, and we know that software is absolutely critical to enable performant AI solutions for our customers. So to talk more about the progress and we've made just fantastic progress over the last year on ROCm and our broader software ecosystem. Please welcome SVP of AI, Vamsi Bopanna to the stage. Thank you, Lisa, and good morning everyone.
As you just heard AMD platforms are powering some of the most important AI workloads on the planet. So today I'm excited to tell you about the tremendous progress we are making with ROCm, our AI software stack that's making all of this possible. Two years ago, when we laid out our pervasive AI strategy, we made open software a core pillar underpinning that strategy. We said we would partner deeply with the community and create an open ecosystem that is able to provide a credible alternative for delivering AI innovation at scale. And today, we are there. AI innovators, from the largest corporations to exciting startups are delivering their most demanding workloads on our platforms.
ROCm is a complete set of libraries, runtime compilers and tools needed to develop and deploy AI workloads. We architected ROCm to be modular and open sourced, to enable rapid contribution by AI communities. It is designed to connect easily to ecosystem components frameworks like PyTorch, model hubs like Hugging Face and over the last year, we've expanded functionality and ROCm at all layers of the stack. At the lower layers, from coverage for platforms, operating systems to higher layers of the stack, where we have expanded support for newer frameworks like Jax, we've implemented powerful new features, algorithms, and optimizations to deliver the best performance for generative AI workloads.
I am so proud of what our teams have accomplished this year. ROCm really delivers for AI developers. We've also been partnering very closely with the open source community. Our deep partnership with PyTorch continues, with over 200,000 tests that run nightly in an automated fashion.
Our CICD pipelines ensure that when developers anywhere in the world commit code to PyTorch, it gets automatically checked that it works well with AMD platforms. That's what it needs. It has enabled us to ship with day zero support for PyTorch, and we have expanded support for key frameworks with significant work on Jax this year, ensuring robust functionality and support for Maxtext.
Work on Megatron-LM has also been super crucial for us for our expanding training engagements. Now, VLLM has rapidly emerged as the open source inference library of choice in our industry. We are delighted with our close collaboration with the UC Berkeley team and the open source community behind VLLM. That's been crucial for delivering the best inference solutions for our customers.
Hardware agnostic languages and compilers like Triton are strategically important for our industry. Triton offers a higher level of programing abstraction with increased productivity and still delivers excellent performance. Last year, we announced that Triton supports AMD GPUs and we delivered on that promise and we've continued our close collaboration with the Triton team to ensure there's expanded functional coverage and excellent performance coming out of Triton for AMD GPUs. We've continued to add coverage for emerging frameworks and technologies, and I'm delighted to share today that SGLang, which is an emerging inference serving framework, now offers AMD GPU support. In fact, I'm delighted that the creators of all of these key open source technologies Triton, VLLM, SGLang, and many more are all here.
Speaking at our developer event. And all this great work is resulting in great support for AI workloads and models on AMD platforms. Hugging Face is the largest and most important model hub in our industry. We announced our collaboration with them in June last year, with the goal that any model that's on Hugging Face should run on AMD, and today I'm delighted to say that over 1 million Hugging Face models now run on AMD. This has been made possible by our close collaboration over the last year, an effort that ensures that all their model architectures are validated on a nightly basis. And it's not just about the number of models.
We've done extensive work to ensure that the most important models are supported on day zero, for example, the Llama 3.1 models came out. Those ran on day zero on AMD. And perhaps even more importantly, several of our partners like Fireworks, offered services immediately thereafter on AMD platforms. And as you just heard from Lisa, we deliver outstanding performance across a diverse set of workloads.
We have been relentlessly focused on performance, from the latest public models to the flagship proprietary models. With each ROCm release, we've delivered significant performance gains. Our latest release, ROCm 6.2, delivers 2.4 times the performance for key inference workloads compared to our 6.0 release from last year.
These gains have been made possible by a number of enhancements improved attention algorithms, graph optimizations, compute libraries, framework optimizations, and many, many more things. Similarly, ROCm 6.2 delivers over 1.8 times improvement in training performance and again, these gains have been made possible by improved attention algorithms like Flash Attention V3 that is supported improved compute communication libraries, parallelization strategies and framework optimizations. It is these huge performance gains that have been key to driving competitiveness and momentum for our Instinct GPUs. But look, model optimization is not the only requirement for AI.
AI production often requires data processing, RAG agentic pipeline development and many, many more things is often significant effort that customers need to put in to realize the value of AI. To solve this last mile of customer AI needs, we acquired Silo AI earlier this year. Silo AI was Europe's largest private research lab and built a stellar reputation, helping customers implement over 200 production AI solutions. In the past few years. With 300 AI experts, including 125 AI PhDs with deep, deep deployment experience. We are thrilled to be able to offer our customers now the ability to implement end to end AI solutions.
This exceptional team has also been behind the development of some of the most important European open source language models, and I'm thrilled to share that those LLMs have been exclusively trained on AMD platforms. Now, I've shared a lot about our software progress, but perhaps the best indicator of our progress is what AI leaders who are using our software and our GPUs are seeing. So it gives me great pleasure to invite on stage four remarkable AI leaders whose work is at the cutting edge of AI to stage. It's an honor to have them here to share their perspective on the future of AI.
So please join me in welcoming these outstanding innovators. Danny Yogatama, CEO of Reka AI. Dima Dzhulgakov, CTO of Fireworks AI. Ashish Vaswani CEO of Essential AI.
And Amit Jain, CEO of Luma AI. It's so great to have you all here with us today. Thank you. Dani, let me start with you. You are an AI trailblazer, having worked on groundbreaking projects like Deep Speech and AlphaStar, and you've actually seen the potential of multimodal AI before many others.
Tell us a little bit about what you're up to at Reka, and some of the exciting work that we've been doing together. Yeah. Sounds great. Yeah. Thanks for the intro. At Reka we provide, multimodal AI that can be deployed anywhere our models understand text, images, video and audio addressing the needs of both consumers and enterprises for developing powerful agentic applications in the cloud, on premises and on devices. We are really, really excited how the models are optimized to run on AMD platforms from high performance cloud GPUs to AI PCs. That's awesome Dani.
Thanks. Now Dima, you are one of the original leaders that built PyTorch. You are also a co-creator of ONNX. You were very well known in the AI ecosystem.
So, you know, tell us a little bit about Fireworks AI and how your open source contributions are shaping your work there. Thanks, Vamsi. So yeah, at Fireworks, we offer a platform for production AI and generative AI with key focus on inference, speed and cost efficiency. So we help companies ranging from startups such as Corsair to enterprises like Uber and DoorDash to basically productionize the latest and greatest open source models acros
2024-10-14 00:54