Adrian Aguilera: Digital Mental Interventions for Increased Equity
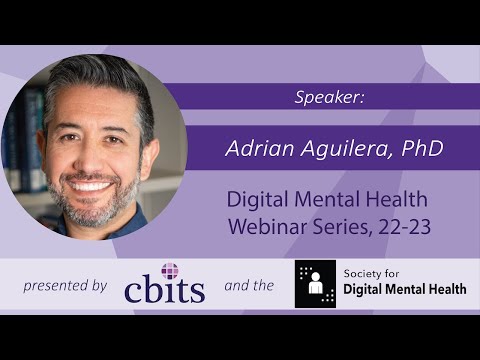
so uh welcome to uh the the uh May edition of the Center for Behavioral intervention Technologies uh webinar uh held in collaboration with the society for digital Mental Health um during the talk uh please go ahead and put questions into the Q a um and I'll go ahead and read them off at the end and today I am really really pleased to uh introduce to you Adrian Aguilera so Adrian is uh I've known him for for many years uh he's an associate professor in the School of Social Work at the University of California in Berkeley and a faculty member at the University of California San Francisco in the department of Psychiatry he directs the digital Health Equity and access lab where he focuses on using mobile technology to improve access and reduce health health and mental health disparities among low-income and vulnerable populations and his work focuses especially on latinx communities Adrian it's a it's a pleasure to have you here and I will turn it over to you great thanks so much David and thanks so much to all of you for joining um we'll go ahead and get started so today I'm going to be talking a little bit about um some of the things we I think we should think about in terms of integrating Equity into digital mental health interventions and then um talk to you a little bit about some of the work that we've been doing in our lab um so first I think it's helpful to get an overview in terms of where uh digital health is in terms of uh what it's targeting and I think one of the reasons there's a lot of potential is that there are a lot of uh apps digital Health interventions targeting mental health there are also a lot targeting diabetes which are two areas that I'll talk about today and one of the reasons that these are two important targets are because they're typically things that need to be managed consistently over time and as we know digital Health provides opportunities to do that um another uh kind of framework that will be helpful to think about as I present today um is this taxonomy of interventions developed by Ricardo Munoz which kind of breaks down the way we can think about uh the relative uh impact of face-to-face intervention compared with technology adjuncts and then we look at uh type 3 which is more self-help but has some guidance to it and then type four is totally automated um so it's important to think about this progression of interventions and different ways to apply technology um there's a little nod to uh David uh David Moore and colleagues and I really think the supportive accountability framework is has been really Central to the way I think about digital health and I think increasingly um uh were I think coming back to this um and really recognizing the role of human support of uh guides um and and thinking about different creative ways to provide support to people doing digital interventions um another uh aspect uh another framework that influences the work that I've done is the efficiency model developed by uh kind of expanding on the support of accountability model um uh by Steven Schuler uh thinking about usability user Center design and then the various aspects that a supporter can help with right so motivation as was is highlighted in the support of accountability accountability model but also thinking about kind of fit some knowledge aspects all in service of increasing improving clinical outcomes so one of the questions that I often ask myself when we're when I'm looking at digital Health interventions is who are you designing for um these are some examples of ads for Apple watch and the Google Fit products and what we can see is that a lot of the target for some of these uh products these digital Health tools they're a lot they're not just digital Health devices I would say but but that is one big aspect of them they target people who are already relatively healthy and I think one of the challenges with doing that is that if we're targeting people who are already healthy and helping them optimize their health we're going to have a relatively small impact on the broader Public Health public Mental Health uh also if we look at language so what are the targets of language but uh for digital health programs and by far English is the biggest uh language represented in digital Health interventions and that really misses opportunities for uh developing in other languages and reaching other populations so an English speaker can go out and look for a product and have a lot of options at their disposal whereas other speakers of other language you know I work a lot with Spanish speakers can go out and they're not going to have as many options so if you're developing interventions thinking about developing in other languages can actually help reach more people and in some ways you even have less competition another challenge uh and I think a limitation of digital Health uh particularly in the private sector thus far and a reason that I think we haven't had quite as much traction as we could is that companies haven't have primarily focused on healthy volunteers um not targeted High burden populations not addressing outcomes such as costs and access to care these are the types of things that are really going to bend the cost curve and they're not Central to the development of uh various digital Health Products so I think until we make that more of a central Focus we're going to have a harder time I'm seeing real impact uh with that we're not targeting for example the Medicaid population as much which is experiencing a higher burden of mental illness compared to other populations broadly speaking just some highlights in terms of some disparities in mental health and digital disparities we know that racial and ethnic minorities are less likely to receive Mental Health Services and when they do they're more likely to receive lower quality Care unfortunately this seems to be translating into the digital space as well we also know that folks from diverse backgrounds socioeconomically and from lower levels of Education in addition to racial minorities often have lowered lower levels of digital literacy and have a difficulty using commercially available apps uh the the primary fear is that if marginalized and underserved populations aren't considered during the development of these tools we might actually entrench rather than overcome existing disparities this is a real uh concern when we look at technology adoption across the three major racial ethnic groups in the US we see that um if we move down to smartphones that there's uh roughly uh equal similar ownership of smartphones so there's access there however we do need to consider differences in access to home broadband to desktops to uh to or laptops in how we develop interventions so data intensive interventions may not be as appropriate for for all all users the national academies of science engineering and Medicine uh held a digital Health Equity Workshop some years back but I think the points still hold that technology is really about people and addressing these disparities goes back to placing people first not technology first and I really do think that um at the Advent of digital mobile health um kind of in the early um you know 2000 teens um there was a real uh kind of fetishization of technology and the real focus on what the technology can do and we did Place technology first and still doing some instances but we really need to think about how it interfaces with with people more so and with that Community engagement is essential in improving these interventions and ultimately in improving health um here's a graphic that helps us think about how engaging with communities can lead to impact so on the left hand side we can see kind of the relative power that we provide communities uh and um the bottom the relative impact and you know we can work with communities and help them inform interventions um but really do most of the work ourselves we can take it a step further and involve communities in the development or we can also have communities Drive um project projects and ideas and targets so it's important to think about this and think about how we can really merge our expertise with the expertise that communities have particularly in terms of defining problems there are a couple of Frameworks that are starting to be developed that are um you know thinking broadly about um digital not just at the individual level so this is an adaptation of uh the National Institute of minority and health disparities I believe that's the acronym um so they've had this framework thinking about the various levels uh of domains of influence on health uh from the individual to societal from the biological to the Health Care system and there's recently been uh in integration of the digital environment and I think it's important to think about not only the individual level which is really important to think about in terms of digital literacy access things like that but even thinking about um how these things move up um thinking about infrastructure so as I mentioned previously the availability of broadband for example and even policy we saw a big change in policy at the beginning of covid when restriction HIPAA restrictions were lifted in terms of Telehealth and now we're seeing the impact of policy now that a lot of the kind of coveted emergency policies are ending so these are all impacting the work that we do um uh uh here's another framework that um that's helpful in thinking about how we can design digital Health more equitably with the night towards social justice is the digital Health social justice framework about by um Caroline Figueroa um along with a few of us as well she was a postdoc at Berkeley uh is thinking about some of these factors in development so first of all equitable distribution right who am I uh who's part of my research who is part of the development um thinking about Equitable design uh privacy and data return stereotypes and bias uh am I reifying stereotypes and bias within the the products that I'm developing and then also considering uh structural racism and this is I know it's challenging because we're working at the individual level and it's hard to you know totally change let's say structural racism however it is important to start to acknowledge uh things like structural racism that people face uh on a daily basis and impact their Mental Health all right so that's the broad framework um that I work under kind of some of the things that I think about as I enter this work and now I'm going to go in and give you a few examples of some of our work um over the years the first project that I'm going to talk to you about um started with trying to address some challenges with implementing cognitive behavioral therapy for depression in public sector settings we know that attendance predicts Improvement in CBT for depression however in our setting 23 percent of people dropped out after one session homework completion is quite low with only 23 percent people completing homework um and half half half half of the people that participate finish less than half of the treatment so not high adherence generally speaking or engagement here's another way to look at the data where we see that most people only attend a couple sessions and attendance drops off the model that we've used at the General Hospital in San Francisco is a 16-week treatment so you would think well you would hope that the bar would be highest at 16 weeks but in practice we see that it's kind of all over the place so this is Data before we uh integrated any technology so we wanted to leverage text messaging to improve treatment quality and engagement in the CDT uh intervention for depression um why did we use text messages so what's not on here part of this had to do with when we started so I started developing this work in 2009 um 2010 when techs were one of the things that was more available and in particular for folks from low-income backgrounds so smartphones were taking off but um the smartphone penetration rate was not as high broadly speaking now that's a little bit less of an issue even still techs are still widely accessible they're cost efficient and there's High engagement rates um and I think another thing that we've learned throughout the course of our research is that there's a greater sense of personal connection via the messaging app right it's something that people already use and that's how they use it foreign so the text messaging as an adjunct to CVT um is uh integrated into group cognitive behavioral therapy for depression at the General Hospital in San Francisco um uh the for the trial we focus on Spanish speakers and we use the bright manual for depression which was developed by Ricardo Munoz and Jeannie Miranda at the General Hospital as well there are four modules over 16 weeks um and what we did is we can we compared a control group which was receiving the Standard Group CBT with one receiving that intervention but instead of being assigned homework they would get automated text messages here are some of the patient characteristics comparing the control and texting group um some of the key things to highlight are on the right in particular most people in uh the study had less than a high school education um but almost everybody on the smartphone and but even if most people on the smartphone not everybody use text messaging so we actually had to teach some folks how to do that during the study um this is one of the core elements of the homework for the intervention where people track their mood throughout the week and then answer a question about some other element of the intervention whether it's tracking thoughts tracking activities social interactions Etc and so what we did is we tried to kind of convert that into a texting format and also sent out medication reminders as well as attendance reminders so we sent a mood monitoring text randomly from 8 AM to 9 pm asking people to rate their mood and respond with the message about what they're doing or thinking they would get responses back about 25 percent of the time randomly to keep some engagement going but also to not overburden participants they would receive theme based text messages where we reinforced elements such as mood monitoring cognitive restructuring behavioral activation and improving relationships this is a basic medication reminder that's pretty Broad and was optional and then a group reminder text the day before session when we receive the mood data it's displayed um on the healthy SMS site and we can look at the pink line for an example of how we'd use it so we might contrast let's say on Tuesday this person wrote six estoying Casa I'm at home compared to eight estoy con Amiga and with a friend so we can pull out a variety of factors if we're focusing on thoughts we can explore the thoughts around these two different times we can focus on the behavior of being at home versus being out maybe with a friend or we could focus on the interpersonal nature of being alone versus being with somebody um so really using the data the real world the real world data to bring the CBT Concepts to life so uh our key outcome was looking at attendance um and engagement with the intervention and what we found is that textures attended over twice as many sessions uh six compared to two and a half and more dramatically they attended uh they stayed in treatment longer so time to drop out was um sorry the text kind of uh overlaps there but 13 and a half weeks compared to three weeks so quite a big difference and I think this is where the key to something like text messaging um shows its influence in that people are getting messages whether or not they're showing up so maybe they lose a little bit of motivation and stop attending but they are more likely to come back after an absence of even a couple weeks whereas people who don't get a message once they drop out they're really much less likely to come back because they don't have that constant nudge of or a reminder that this resource is out there so a texting adjunct can decrease treatment attrition which can lead to more people getting better ideally uh we also um uh wanted to use this data to try to um predict outcomes and improve the intervention further and so what we looked at was how can we predict whether or not someone is going to show up to session and we found that a mood the day before session predict whether or not somebody's gonna attend such that a one point increase from someone's Baseline increases the chances of them attending by 32 percent or vice versa lower mood is less likely to attend as well and this is important because we can identify critical points for intervention informing Tailoring we can Outreach either be an automated format or be a clinician outrage we also have found that mood ratings in the past week map on to phq-9 which is relatively straightforward but it's also important because this may allow for remote monitoring of larger numbers of people in the age of screening particularly in Primary Care this can be a low burden simple way of monitoring folks and we find it's a big Improvement on uh asking phq-9 questions particularly for folks with lower levels of health literacy or literacy period we often would have to sit with people and ask the phq-9 questions and it could take you know as much as 15 minutes to go through the the questionnaire whereas simple mood rating is pretty straightforward some of the feedback that we got um more from the development from the development stage of the intervention uh this is feedback from English speakers and as you can see a lot of it's related to being self-reflective um being aware these are all things that we really try to um to try to reinforce with clients who are working on improving their mood is to First be aware of how you're feeling so we were really excited to see this um and then we asked Spanish speakers same question as you notice the theme is a little bit different so a lot of people also mention self-awareness but they tended to mention more often that they felt cared for and supported um they said it in many ways um uh they make me feel that there are people that care about my health so even though these are automated messages there's the sense of connection and we know that culturally this makes sense given that um Spanish speakers from Latin America tend to have more of a relational framework where they see themselves in relation to other people in the world and that includes their health and mental health so it's important to recognize that what we develop interacts with what people bring to the table as well in this case culturally in terms of suicidality we instruct patients this is not direct communication but patients still feel a connection there are keywords in the system that can trigger messages to the clinician so that we can go in and see if there's anything concerning in the message so from this line of work I'm using the mood text program generally speaking um we have found that patients who texts attend more and stay longer prior day mood rating predicts attendance mood ratings via texts can approximate phq-9 patients like interacting with automated texts generally and broadly digital literacy issues are real but they're addressable all right um so now I want to shift gears a little bit um and tell you about kind of the the follow-on project from mood text uh which was done in collaboration with my colleague Courtney Lyles who has expertise in diabetes and I think uh from our side we recognize that within primary care a lot of people with depression had chronic illnesses um diabetes being one of the most prominent particularly amongst um uh Spanish speakers and folks from Latin America diabetes is a pandemic you know before the the covid or epidemic I should say um however interventions are siled so we wanted to think about bringing the intervention uh interventions for both together and we honed in on physical activity as a core mechanism and addressing a few things within the development of the intervention that I think many of you will agree on is that mobile Health interventions need to be personalized um they need to be integrated into care and they need to be developed with low-income and vulnerable populations in order to have that broad impact that I was talking about earlier um so we know that both diabetes and depression increa improve with uh increased physical activity we think about it in terms of behavioral activation with depression um but um this is so it's not purely uh behavioral activation in the sense of uh Pleasant activities but it's a good proxy for it so we The Innovation that we applied was a reinforcement learning and I'll talk a little bit more about that but the idea is that we are aim to increase physical activity by first passively sensing steps and then using a reinforcement learning algorithm to personalize messages that can Target specific motivators that are most impacted um this is the Diamante app it's a pretty straightforward app um uh tracking steps and then here are the messages that we sent out burst a feedback message about um how um how many steps somebody walked really relative to their goal and then a motivating message afterwards we use the com beat framework to develop our messages um and the idea behind this model is that a behavior is influenced by capability uh opportunity and motivation and so we developed buckets of messages within the capability motivation and opportunity boxes for motivating Behavior we applied reinforcement learning which is a type of machine learning that focuses on um taking actions to maximize a reward in our case the reward is Step counts and I'll go into it a bit in more detail so here are some of the factors for those of you less familiar with reinforcement learning I'll go through it quickly so first we think about action variables so what is the algorithm going to do contextual variables which are variables related to a participant so um they're characteristics data related to them reward is the outcome so in this case it's steps and then the model is how we predict um that reward so the action variables are those feedback messages that I mentioned so they could get one of four messages so either no message or a different way of framing their goals they would get a motivational message again no message or benefit self-efficacy opportunity message a third action variable was time of day so we wanted to see if there were times of day that would be more impactful to receive a message these are some of the factors the contextual variables that we included in the model so every morning the algorithm is retrained on data of all participants and all this data gets put into kind of thinking about it as a as a slot machine or a multi-arm bandit as it's called in the Cs world and so uh the the algorithm generates um predictions around what type of message will result in how many steps and then it chooses a random message from a category it's important to point out that the ideal or best category is not always the one that gets sent out because there are also combinations of messages that may have an impact um all right so uh so we apply this model to patients in at the General Hospital from primary care clinics English or Spanish speakers with diabetes diagnoses and high depression symptoms unfortunately during right we're getting off the ground during covid and had to shift gears and and include some social media and online recruitment primarily from Craigslist to supplement the the recruitment that was quite challenging during those initial stages of coven we ended up we just finished uh this trial back in December and this is the final um uh the final numbers we had three groups where we had an Adaptive arm that had the uh reinforcement learning intervention we had a random arm that received random messages um and then we had a control group that only got the app um and was asked to track their steps over time and all of these received a weekly kind of mood monitoring message as well um here's some of the participant demographics so importantly there are no significant differences of randomization worked um it you can see that we're relatively um diverse there's about 22 to 20 uh between 22 and 29 Spanish language speakers race and ethnicity is a broadly representative and education levels are also relatively broad this is likely that the inclusion of primary care participants at the safety net clinics you can also see a relatively large number of folks who are unemployed or experiencing disability which I think again reflects the low-income or vulnerable state of part of our population at least um the in terms of uh pre-intervention steps uh generally speaking folks had low levels of Step counts so between uh 3 000 to 3 500 or so um so low activity in this group and we found that the Adaptive environment did indeed increase steps over time compared to the random condition and the control arm so this is an indication that the reinforcement learner did indeed work um we just ran these analyzes so we're kind of digging in a little deeper and hope to publish these findings soon but we're excited about this finding so texting um Can can be helpful again particularly if we personalize and make it adaptive using some of this machine learning in the background ideally we can use these types of approaches broadly and I think what's really important is is to have broad representation within our samples particularly when we're training AI models machine learning models they're not always the most representative so we think that's really important to build this work from the ground up all right um I want to leave a little bit of time for questions so I'll run through this part um a little bit more quickly but I'll tell you about our StayWell project which started at the beginning of the kobit 19 pandemic um mostly as a service to send folks messages uh send folks messages for 60 days to help them cope through some of the challenges of social isolation and the mental health side effects of the coveted pandemic so we sent them two messages a day based on CBT and DBT principles these are some examples and we also asked them a mood rating question afterwards again we applied reinforcement learning to a third phase of the project so the first phase we just sent them out to a lot of people about 600 and found that there were improvements overall but we didn't have a control condition because we didn't feel it was quite ethical during that period but we wanted to apply some of the Diamante reinforcement learning to this project as well um so we had three groups in the third phase where uh the control group received a mood rating message and then sometimes feedback to that mood rating um the random group received a random message then a mood rating then feedback and then the reinforcement learner uh received um a more a reinforcement learning based message um using the mood rating as a reward variable and then a feedback to that mood so overall we found that um there were decreases in php9 and get seven symptoms so we did recruitment both in via Facebook and also via clinical trial registry called Health match as you can see the health match folks have higher symptoms um so they are above the moderate threshold and they dropped almost below almost in the mild range but not quite so there were overall effects however there were not um significant differences um across um the control random and reinforcement learner groups with the only exception being a difference in the Gad 7 score in the health match group between the reinforcement learner and the control group so that pairwise comparison only um so it didn't quite work um and we didn't get those significant findings that we saw in the Diamante trial um some of the reasons for this there are a couple reasons um one is that here we use an individual level model where the algorithm was trained on individuals responses to the messages whereas the amount that we use a group based model so we were taking advantage of everyone's data and so it may have taken too long for this intervention to learn since it was only 60 days and another reason is that it looks like the control condition which is a mood monitoring condition may have been too strong of an intervention and one of the indications we have for that is we looked at feedback from people and these are ranked from these are three random people that provided feedback the first uh the one on top was somebody who rated the intervention highly they loved it the middle one was somebody that thought it was okay and the third one is somebody that they didn't love the intervention they wouldn't recommend it but all of them you can notice found that it helped make them aware of their mood and I don't know what intervention what um what uh condition they were in um because they were pulled at random but this was a consistent uh piece of feedback that we received that people are aware of their mood so that may have been too uh strong of an intervention and we do know that being aware the mood is a core element of most of our interventions of CBT and in some ways it's almost a mindfulness intervention in some ways as well um there were some other kind of pieces of feedback mostly around personalization and uh providing more resources we also did another phase of the study where we compared um latinx participants with non-latinx white participants and found that the latinx folks had more Improvement overall compared to the the white participants based on phq-8 and there was more people there were more people that moved into that improved into uh from the moderately depressed to the mild category among the latinx folks and this is um I think interesting and related to a point I made earlier about addressing uh more diverse participants and having more resources available to them that are targeting them um so as I mentioned Spanish speakers don't get a lot of don't have a lot of uh access interventions relative to English speakers um and uh it's a way to I think meet more of a need than continually going after kind of the same population so effects are relatively small but they are scalable all right and uh hopefully I didn't talk too fast but um wanted to make sure to leave some time for questions I want to thank um you know funders and everybody that's participated in this Pro on these projects it really is taking a team uh in particular um Chris Carr and audacious software who's been a partner through all these projects thank you all now I think we have some time for some questions thank you thank you Adrian that was a terrific talk so uh if folks have questions please go ahead and put them into the Q a um see that at the bottom of your screen um and and I'll I'll just uh go ahead and start I have a bunch of questions so one is you know one is it was kind of interesting in the first study that you did looking at at text messaging with uh to support you know standard CBT therapy you know it's clear that it it had it could you know it had a a strong effect even though it was you know just pretty rudimentary it seems like in the kinds of messages and then in the Diamante study you it looked like if I saw that correctly the the reinf the targeted personalized messages the ones that were delivered by a um with reinforcement learning produced a nice effect but you didn't see it with you didn't see much of an effect with the random messages and obviously you have a you know a fully automated treatment versus something that is adjunctive to care but I wonder if you can talk about those differences and why you think you might be seeing those yeah well I think that you know a lot of this goes back to kind of the supportive accountability idea I think the first one is very much the basis is um the face-to-face uh traditional intervention and you add on this layer of technology and so I think the technology is working to help with the engagement piece because most of the intervention is being delivered in person right and so it's a little bit of maybe a little bit of practice outside but the core you know you can't match that one and a half hour more intensive intervention compared to you know whatever you take a minute or two to read and respond to a message um and in the the amount of the trial it's uh it it's all uh all mobile so it's tracking your steps and then getting the messages right um and I think also importantly is that one lasted six months which I didn't mention so a lot of these interventions I think if we do them for a month or two we can get some good effects but over time sustaining that for six months is hard and my sense we haven't gone back into the data but my senses particularly with random messages they might get pretty old after a while right maybe after about two three months you just kind of um they don't have any effect right they're just kind of coming at you randomly and the Adaptive messages may allow for some of that targeted nature maybe they're they're um adapting over time as well um and so those are some of the things we're going to look at particularly which categories um maybe were most impactful most helpful so I think those are a couple of different factors and I think it goes back to thinking about again not just the technology but how we're applying the technology where does technology fit into the broader kind of ecosystem of care yeah yeah there's another question but I just want to have one file take my My Prerogative here with one follow-up it's in the the comparison of the the the the reinforcement learning in the Diamante study versus the the uh the health the healthy SMS part of the difference there in the the how useful reinforcement learning was is is um that you know in Diamante you're you're you're using the a behavioral Target uh for reinforcing you know that people is actually actionable and yet and and and using mood I mean that's not really actionable you know that's not what people need to do and I'm wondering if that might be part of the difference that you're seeing there I do think that that's part of it I think one of the things we want to do um so I think you know behaviors are so much nicer because they're they are concrete and they're not subject to interpretation you either did it or you didn't do it right and sure there's there's error around the sensing and whether we caught all the steps but some of that washes off or washes out over time with mood you know I could say I'm at a nine but maybe really I'm I I'm you know avoiding something right I've was telling somebody um uh last week that you know I I used to have I had a client that would have zeros on his phq nine every week but the second he started talking in therapy would start crying right and so the self-report we all know that self-report is not quite as uh accurate um as you know what you're doing as you know reporting by walking right there yeah so one of the things where just the last point there we're gonna try to run some simulations to look at you know what a group level model improve the outcomes of let's say the StayWell algorithm um to try to understand that aspect of it a little bit Yeah Yeah all right um so a couple questions here from Neo Gabriel um so thanks for your talk do you have thoughts on how to tailor texts in the future or make things more interactive while maintaining scalability and then the second one uh can you please share your experiences with healthy SMS we're preparing to launch a text messaging study and are considering uh text I.T any Lessons Learned and tips using the texting programs software yeah um so I think there's a lot um there's a lot to be done with tailoring I think that um I really like the reinforcement learning approach broadly speaking it's in some ways It's relatively straightforward but it's learning it's adapting over time so I want to keep kind of doing that um I think we have to look at um uh language models chat GPT Etc to think about how to integrate those I think that what we have to do there is be careful if how we're presenting those options um some of you may have seen that Coco did a kind of internal study where they had chat GPT generate responses to participants and participants rated those more highly than than responses by live humans until they realized that they were generated by a chat bot versus a a person right and I think that you know we need to be transparent but we can also blend things to some extent so there may be ways for example of what we're starting a project working with peers in the community who may not have a lot of training but maybe we could use these language models to generate options right so of these three options maybe choose one to send or to adapt and so we need to figure out ways to blend these things to as you mentioned to to maintain scalability um in terms of healthy SMS I'm biased because um it should be in my disclosures uh I I developed healthy SMS with um with Chris Carr and we've licensed it to folks so that's really mostly what we focused on in part because when we developed it there wasn't much out there um so um it's it's worked really well as a scheduling um kind of software to meet our needs and some other folks have adapted it in different ways and now we're also integrating some of the machine learning modules through our colleague Joseph Williams in Toronto so there's some interesting aspects to it but it's not quite as refined as something like text it or text ID I don't know it's pronounced all right um and a question from Chelsea Brown so thank you for sharing your findings in the mood Tech study um mood day before session predicts attendance was there a brief mood intervention that emerged as the most successful way to improve mood prior to a session and therefore improve attendance or is that something you'll explore in the future yeah that's a great question so we didn't um uh we didn't quite get to that point the what we were um hoping to do and we actually got um interrupted by the pandemic again is to um provide kind of an enhanced reminder to people who had lower mood so if you sent a lower mood to say um you know maybe the day before we've noticed you may not be feeling um you know as great as you have been in the past uh you know remember that you know coming to session maybe may help you get out of that funk or something along those lines right so the idea is wording things in different ways but that's the way we've been thinking about targeting is to recognize what's going on and to provide that nudge all right um well there's no other questions coming in so I'm just going to go ahead and ask other questions that I have so this I'm torn here there's two directions I could go so so one I guess is in the implementation Direction so like you the first study that you presented I mean it's just so it seems so obviously clear that this is a benefit and and you know could be relatively easily imp you know it seems like something that would be relatively easily implementable but I you know I think any of us have tried to implement know that it's like it's uh extremely difficult so so what are your thoughts about like how you know how to successfully Implement something like that because it seems like the benefits are so obviously so obvious and clear yeah I I mean I agree it's honestly been a little bit probably a little bit frustrating that um we haven't been able to do a little bit more I think we were on the track of doing a little bit more of that implementation and then covid kind of messed things up we're going back to a little bit more of that you know regular um world of of having more of this type of care um but some of the key factors that I think limit implementation um are actually based on more clinician level factors um there are a few clinicians that I worked with on this project who are really excited and wanted to roll it out um but there were many who were worried about being responsible for messages coming in at all times of the day um you know liability around suicidality um those are some of the key factors that people worry about right and I think this speaks to a little bit of the policy challenges and and the balances of um you know being worried about liability um but then that sometimes leads us to withhold something that could be beneficial to parchments and that's a balance that I think we always need to hold and it's one of the things that I think has limited um this taking off a little bit more quickly um I mean another is more practical in the sense that we developed it for this type of CBT intervention so it was kind of scripted out in that sense so we would have to think about different ways um to develop it with interventions that are a bit more um you know adaptive in a sense right and maybe uh flexible by a participant but I still think that um something as simple as mood monitoring right um throughout the week in uh in combination with with therapy I think can be really valuable um but but you're right we haven't quite rolled it out part of it's also a matter of you know Finding partners and kind of scaling this up a little bit so folks are interested I'd be happy and you only have 24 hours in a day like the rest of us yeah there's that too so uh Chelsea Brown has won an additional uh request to share a reference or a link with the reference to the chat GPT study if you have that I'll look for it it was it was a just a Twitter post to be honest but I'll see if I can find then uh uh Karina claimshack um I'm interested in using ml with my tech work but it seems like uh such a jump to Learn Python how did you get started with ML as a psychologist or do you worked with cs collaborators uh who do ml implementation yeah um so the the answer is the letter so I've worked with folks who are um in computer science and so I don't do the ml I've um you know started to learn how to talk about it learn the terms but haven't done it myself one thing I will say is um you know I I also think chat GPT and things like this are helping because there's some level of coding that they can start to do um and you know it's not perfect but um that combined with the Cs uh you know student for example can get you somewhere at least for a smaller scale study all right and then there's another question from uh Jason koldis which sounds like the same one that Chelsea had wanting the the citation to that and you know given that it was a you know a post that you saw there it sounds like it's uh the things that people could use to search uh it sounds like [Music] here I just found it right now oh you did okay it's more it's more his article but it gives um but you can uh oops yeah no but it sounds it it certainly sounds plausible and it also sounds like the kind of thing that um you know our culture around this is probably going to be changing pretty rapidly um so what people are finding today may not reflect how people feel about it uh you know six months or a year from now and it's also generational I think that you know people growing up in this age of that kind of interaction they're probably going to take to it much differently than people who have you know grown up and more human interactions for better and worse right I think we also need to kind of keep an eye on to what that means about how we you know live and relate to other humans but um there's a lot of variability yeah well all this world of texting and chat gbt we're probably all thinking about how how we can use it and what the benefits are and what the uh you know what the what the risks are uh patients so we are just about at the top of the hour here so thank you very very much Adrian for a really stimulating talk yeah thanks so much for the invitation all right and folks so for uh next next month the first Tuesday uh will be Gene Rhodes who will be talking about scaling Mental Health Services through technology enhanced mentoring and Gene's work is really around how to develop programs that can use lay and peer coaches how to manage them how to set them up and she'll be talking about how sort of more on the coaching side so until then thank you very much and we will see you next month goodbye
2023-05-11 11:28