3 New Groundbreaking Chips Explained: Outperforming Moore's Law
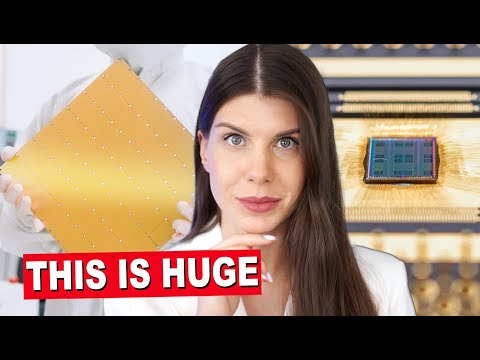
We need bigger GPUs last week was an incredibly huge one in tech and as a chip designer I'm beyond excited so today we will have a look at the three hottest headlines of the last week the new Nvidia Blackwell GPU why is NVIDIA going for larger chips and making some serious tradeoffs? then we will discuss the new 4 trillion transistor chip from Cerebras and the new kind of analog chip we were all waiting for. NVIDIA is now at the top of the world we've never seen such a profitability from a hardware company right that's one of the reasons why my Investment Portfolio looks so great and now they've revealed their new Blackwell GPU. 208 billion transistors and so so you could see see you I can see that there's a small line between two dies this is the first time two dies have been like this together in such a way that the two chip the two dies think it's one chip this new GPU providing four times the training performance and up to 30 times the inference performance compared to the previous generation the Hopper GPU first of all let's discuss how did they manage to achieve this fourfold performance. as a first step to double the performance Nvidia has to double the area huh right but that was an expensive decision because the price per chip is actually the price per area which depends of course on the technology note and the volume in fact Nvidia had to keep using the n4p process by TSMC n4p process is a refined version of the N4 with a 6% yes just 6% transistor density boost and 22% more energy efficiency over the N4 unfortunately Nvidia had to stay at this process node because TSMC is currently struggling with this 3 nm process to be specific they are struggling to achieve the satisfactory yields and this of course impacts not only Nvidia but also the road maps of AMD Intel and other chip makers in a bid to maintain its competitive advantage Nvidia had to introduce use double die design which is packaged using TSMC's Chip on Wafer on Substrate CoWoS-L packaging technology this packaging technology is used to integrate multiple dyes side by side to achieve better interconnect density and with that you can achieve high speed and high bandwidth communication between the chips compared to conventional packaging methods that's how they achieved nearly one single silicon now if we can see consider the dual die design and the packaging the cost of fabrication of this GPU more than doubles more than doubles in comparison to the previous HOA GPU so they will be definitely not getting to their legendary 85% margins as they used to and they had to go for this tradeoff for this painful tradeoff to maintain their competitive advantage because as we will see the competition is hitting up. all the hyperscalers are now developing their own
custom silicon like Amazon Google Meta everyone is designing their own AI chips and also as you know AMD and Intel also want to get the piece of this pie startups like Cerebras and Groq also have some solid alternatives so yes Nvidia is definitely a leader in AI hardware and making great efforts to stay so but the competition will not let them to rest for a moment. we've seen that doubling the silicon doubles the performance but where the second double fold is coming from? it definitely doesn't come from the new process node but rather from the new numbering format it's coming from lowering the precision of the whole calculations you know we can encode the same number in let's say 8bit 20 bit or in 4bit just what will change is the precision but for the most calculations within the neural network it's not really essential to compute let's say 20 digits of which number the network can accomplish the same task at the same accuracy at a lower level of precision and that's precisely the trick here if we lower the precision of calculation let's say instead of 8 bit numbers we will be using 4-bit numbers we can immediately save the half of the memory because smaller numbers requires less energy to compute requires less memory bandwidth and the logic which is required to do this math takes up less silicon in the previous Hopper GPU they've used floating point numbers up to 8bit precision but with Blackwell GPU they've taken it one step further in the new Blackwell architecture the matrix multiplication units doing math with numbers just 4 bits wide this is another area from where the improvement in performance comes from. honestly 4 bits is quite low and that makes me curious to see how well it's going to work for inference application for example let me know what you think in the comments to summarize it the improvement in performance coming from connecting two GPUs together supporting very low Precision FP4 format a massive amount of high bandwidth memory and improved interconnect bandwidth as simple as that this GPU and the super computer DGX Superpod built out of it will be available for sale later on this year in one of the interviews Jensen hang said that they're going to price it somewhere in between 30 to $40,000 and I have many doubts about this first of all since the h100 was selling for about 40,000 last year so Blackwell is likely to be priced higher than that for now I'm really looking forward to see the real world benchmarks we are discussing different AI chips today but just to give you a feeling of how high is demand for AI infrastructure is there is a recent quote from TSMC's founder Morris Chang regarding the demand for AI chips he says we are not talking about tens or hundreds of thousands of wafers but instead building three five or 10 fabs but we need bigger GPUs now let's discuss the new starting 4 trillion transistor chip from Cerebras this one is pretty unique and they are crashing the Moors low you know that since the advent of microchips in 1972 the semiconductor industry has followed Moore's law it states that the number of transistors on a chip is able to double roughly every 2 years as you can see from this plot Cerebras seems to be outperforming this law which many had believed was no longer applicable their previous chip was fabricated at 7 nm by tsmc and the new one the wafer scale engine 3 is at 5 nanometers the number of transistors on the chip is more than doubled since the previous generation thanks to the technology node upgrade but as we know a huge success of this chip is a success by tsmc which is able to fabricate such a giant gigantic chip at 5 nm with a high yield one of the reasons why Cerebras was successful over the last years is that they were doing things differently than others while a silicon wafer can typically accommodate many chips and that's what typically AMD Nvidia and Intel are doing they are cutting such a 300 mm wafer or 12in wafer into let's say 65 gpus while Cerebras takes this wafer and makes a single giant chip out of it to give you a feeling of the scale of this this is the new Cerebras chip next to the Nvidia h100 GPU it's 56 times larger than Nvidia h100. Amidst the ongoing AI boom there are many promising tech startups you may like to invest in such as cerebras but the problem is that investing in private equity generally is not easy however Linqto removes these barriers making the access to private markets simple and open to everyone through Linqto platform you can invest in some of the most promising AI Tech startups I've discussed on my channel such as Lightmatter the photonics AI startup I disc discussed in the previous episode in addition you can invest in SambaNova Spark cognition and others you can check out the full list of startups on their website if you're interested in investing in the future of artificial intelligence consider starting your private equity portfolio today using the link below by using the code ANASTASI500 you will receive a discount $500 off on your first investment the code is valid for 30 days only thank you Linqto for sponsoring this video the rate at which we're advancing Computing is insane and it's still not fast enough so we built another chip Hopper is fantastic but we need bigger GPUs going for larger silicon is such a great idea and it totally makes sense for today's AI workloads and Cerebras was doing it before it became mainstream it's beneficial because many GPUs have to be used for a single AI task and interconnecting them and distributing the load is a complex and expensive task to do but by having one giant chip you can significantly reduce the costs and complexity needed this new Cerebras chip features nearly 1 million AI cores 900,000 AI cores and 44 GB of memory and when it comes to memory in this case it is on chip memory that is intertwined between the computing cores and this has exactly the same goal that we discussed in many of my previous videos to keep the memory and the computing cores as close as possible together to reduce the bottleneck and that's another architectural difference compared to Nvidia and AMD gpus which have off chip memory this new AI chip is designed to train the Next Generation of giant large language models with up to 24 trillion parameters in size just think about it it's 10 times larger than open's AI GPT4 and Google's Gemini the next step is to connect 2048 of such chips together to build an AI supercomputer and this one will be capable of reaching one quarter of a zettaflop (10^21) performance as one of my colleagues like to say oh dear such machine for example could train a 70 billion parameters llama model from scratch in one day it's pretty clear that the trend is headed towards larger silicon but the thing is with the larger silicon that whenever I talk about cerebras for example you always ask me about the yield about the defects and you're totally right the bigger the Silicon gets the greater is the yield challenge in especially for the small process nodes like sub 10 nm because then the transistor features become so uh fragile and so tiny that a single particle a single Dust Landing on a chip or a single defect in a chip can kill not just a transistor but a large part of the circuit can you imagine that and obviously you cannot get 100% yield and this would mean that cerebras would have to scrap every single wafer this would have been such a disaster anyway Cerebras manages to sell every single chip that they make and whenever defects occur they have a workaround a defective AI Core can be bypassed in the software and then replaced with one of the Redundant or so-called spare course this way you always get a configuration of 900,000 AI cores with no wafers wasted and of course Nvidia is facing the same challenge which is a headache for tsmc and that's the reason why they didn't get to uh three nm process because the yield is at I don't know 80% so it's quite poor eventually they were able to find a tradeoff let me know what you think in the comments and if you're enjoying this video consider subscribing to the channel and sharing this video with your friends and on social media this helps the channel a lot thank you it's clear that AI is in desperate need for a Hardware Revolution and everyone is looking for a type of architecture that can mimic our human brain because our human brain is still the most efficient engine for non-artificial intelligence we've known for decades that analog can be much more energy efficient and area efficient than conventional digital chips if so then why analog chips haven't become mainstream yet well because there are a plethora of problems we've discussed them in my previous videos we will also talk about it today but the new in charge chip addressing the most of them and also taking analog Computing to the whole new level first of all many Computing tasks and especially generative AI requires tons of memory tons of memory to deal with data and parameters of neural networks these computing tasks are dominated by just a few basic operations that draw on memory the cost of accessing the memory can be orders of magnitude higher than the energy expanded on the computing operation itself now what if we could make this memory intense tasks more efficient and by that make the overall thing orders of magnitude more efficient one of the emerging approaches addressing this memory bottleneck is near memory or in-memory computing and that's usually implemented in analog fashion analog means that instead of operating with digital signals like zeros and ones and conventional transistors analog chips are working with continuous signals and a continuous signal can be anything between zero and one and then we use analog circuits which are consisting of for example resistors and capacitors and the new EnCharge chip is taking this concept to the new level actually the main the key operation that is at the heart of AI programs is so-called matrix multiply accumulate operation you may remember talking about it in many of my previous videos so you probably already know it what happens is that a chip loads input values into the memory and then multiplies these values by so-called weights many such multiplications are performed in parallel and then the result the output is added so added up this is known as accumulate operation and there were already many and many attempts in the past to implement this operation in analog way for example the Mythic chip which I previously discussed it performs multiply accumulate operations in an analog circuit using resistors and then sums up the currents at the output however along with this various problems associated with noise mismatch accuracy cropped up Mythic has really struggled really struggled to find solutions to these issues over the last years and eventually they pivoted to a different application well and charge approach is different their computing is carried out using charge-domain computation with metal capacitors and I think it's a great idea let me explain instead of performing the entire Matrix multiply accumulate operation in analog they're performing multiply operation in digital with transistors and then the accumulated operation is implemented in a very interesting way in analog using capacitors and the trick here is that instead of adding up currents at the output they are adding up the charge in a capacitor so they're basically accumulating the charge in the capacitor which is a great thing to do because it's quite easy and precise and moreover they're using the capacitors which are coming anyway for free you know billions of transistors on a chip they are interconnected with the metal wires which can be seen like a multi-level highway with up to 10 or 20 layers deep and in this chip they are utilizing the capacitors which are made of the parts of this metal interconnects that sit on top of the transistors and the best part about this that these metal capacitors are really easy to deal with they don't have any uh temperature dependency so company mismatch and the size is very well controlled with the CMOS technology so it's a good element in general you know and the best part about this that they're performing analog computing using digital technology which is very Advanced which which is easy to deal with with all the EDA tools that we have now and they've already made a first prototype of this chip which is reportedly showing a striking Improvement in energy efficiency it's capable of 150 trillion operations per second per Watt which is at least 20 times more energy efficient than previous analog chips like Mythic for example on top of that they've also built a software stack for it that manages this whole access to the memory and their first commercial product is already coming later on this year looking forward to it as a first step they are targeting inference applications which means taking an already pre-trained model and running it locally on the chip And here the main goal is to make it more energy efficient and that's exactly what Analog Computing is good for and it's such a low power you can put it to the age devices for example to your phone but afterwards according to the end charge this approach can also be scaled to the AI training I really love this approach when I read it I was like that's good because the trick is that in CMOS technology a capacitor is the most reliable thing you can really get and in general this approach takes the Best of Both Worlds analog and digital and as it's based on digital it can also scale quite well you know it's been a dark time or you call it also winter for analog technology I think but now it's getting warmer and the spring is coming and as always I'm looking forward to reading what you think about this technology in the comments I love this decade the decade of technological acceleration and I love making the videos about it you know for you guys and to build the community around this channel thank you for being a part of it and if you want to support the channel me creating these videos you can check out the patreon the link is in the description below and also check out the sponsor and if you want let's connect on LinkedIn honestly I never used it but I changed my mind so if you want you can scan this code and uh let's connect thank you so much and I will see you in the next episode ciao
2024-03-29 21:53