Solving for the Future of Analytics & AI with Google Cloud (Cloud Next ‘19 UK)
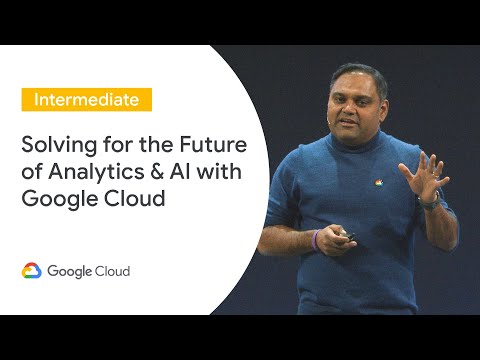
Hello. Everyone. How. Are we doing good still. Great. So uh the. Next 15 minutes we are going to spend on talking about smart analytics, if you heard the keynote earlier today we, highlighted few of the key capabilities that, we announced. At the show but. We want to take you through few, more details about couple, of customer examples we, also want to show you some cool demos of things, we did not highlight in the in the keynote earlier today, so. If you really think about it our first thing before that your. Feedback is really important. To us so please take time to go ahead and provide the feedback Allah, enables us to improve the content for next time the. Feedback form will be available after 20 minutes of session starting, so while. I'm talking if you get bored you're absolutely okay, to go and give, feedback but always give five stars okay so. If. You really think about it. You. Heard from lot of customers, even yesterday how, organizations. Are digitally. Transforming. Themselves using. Google cloud but. One of the things I am super excited about is how. Organizations. Are reimagining. Their, whole industries. And how things are moving like from, self-driving, cars and how massive amounts of data is being collected for, Ford. For driving, cars. Now and driven through the cloud but, more importantly, even the traditional industries. For. Okada which is the grocery, retail, organization. Out of UK, how, they are collecting, massive amounts of data, understanding. Their whole supply chain what, product, is going where how much time does it take and not, just leveraging, it to improve their own organization. But also sharing, some of those metrics with their partner, so that they, can improve their organization. Their supply, chain and all another. Great example of this is just gaming if. You think about King, which is one of our. Liked, users, of Google cloud they're, collecting, billions of events they have 200 plus games, that they that they have mobile games they collect all these events that are coming from all the various games trying. To provide much better customer. Experience, in game experiences. Built, on top of these massive, amounts of data sets and we see this across multiple, gaming, organizations. Across. The globe where where, real-time, events are being used to provide much, better customer, experience. Another. Example is, just traditional. Media. Organizations. Across. The globe the, Daily Telegraph in, in UK but, we have similar examples, with, Hurston. Us various, different organization, across the globe trying, to reimagine what, the industry, looks like taking. The information. About. What customers, want to go ahead and listen, what kind of articles. They're interested in how do you get new subscribers. On the digital platforms, digitizing. All the, content, and also super. Exciting, to see how the. Industries, are changing, and how industries, are reimagining. Themselves. If. You look at our just. Momentum of Google Cloud especially. Around analytics you. Will see we, have organizations. Across various, different. Industry. Verticals, from retail health care financial, services, media entertainment. Gaming. Energy. Automotive. Transportation across the globe, we are seeing tremendous momentum. Offered. For, the the smarter analytics platform, on on Google cloud and it's, also interesting to see the kinds of scenarios that people are using I will, talk more about a couple, of them later but we're not just limited, to, like. Just bi and, reporting, kind of scenarios, organizations. Are using their, data to, innovate, to build, different kind of customer experiences, and do, really, large-scale. Analysis. On top of their data so. What are we trying, to focus on at Google cloud our, vision is, very simple, it's, we, want to create a radically. Simple, to use intelligent. Data platform, that. Provides actionable, insights. In real time for. Organizations. To drive, digital transformations. With. With focus. Our loard focus on large, enterprises, what.
Does That mean other key things that I try, to highlight is it has to be simple it. Has to be intelligent, it, has to be actionable, it. Has to be in real time and. Focus on enterprises. So, if you look at that and look at our strategy of investments, so, as part of my role I'm responsible for now. Where we invest what kind of capabilities, we are building so if you think about it it's, one being, enterprise ready what do I mean by that focusing. On scale. Reliability. And security. Making. Sure that that's the fundamental thing that we have focused on across, the whole platform, the, second is ease-of-use we, want it really, easy for organizations. To derive insights, from from, the data that they've collected it's, not just important to break, the data silos bring it into cloud but, also it, should be very simple to. Go ahead and access that information get. Value out of it this, is where our big investments. Around big. Sheets or connected, sheets comes in so, that you can derive, insights, and share it across your whole organization now. The, next thing is making it real-time and intelligent. This. Is one area around. Bigquery, ml real-time. Collection, like as Thomas mentioned we, have improved our ability for, you to go at an ingest data into bigquery by. Just 10x you can get millions of events per, second, in real time and then analyze on top of it so it's a lot of innovation, happening in that that space and finally. And most importantly, we are big believers in in, open source and and, multi cloud environments, so we, have we have been investing, a lot in that space making, sure that you have all the open source tools and capabilities you need in the platform, for for. Analysis, you. Saw this slide a version, of this slide earlier today in. The keynote but the key capabilities, that, we are trying, to provide with the whole platform is of course one ability, to collect data in streaming, and batch format, scale. Process. It in. With. Open source technologies, using. Data proc or with, our cloud native capabilities that, are there making. It super easy to go ahead and build ETL pipelines, with data fusion or. If, you want to do data wrangling, as as, a data analyst, you can do that with cloud data prep. You. Can then store your data structured, unstructured, on. Google cloud storage for a data leak or big, query for a data warehousing scenarios, and then use the, sequel, engine that we have with bigquery or use, a data, proc which provides, you different, kinds of engines from open source like, spark flink, and all of that to, run run those algorithms. And then finally you can understand your data with with, cloudy I as, well as various, BI partners, and then, more importantly underneath, it we are providing, data, catalog, capability, where, we will automatically.
Identify All. The data sets that you have cataloged so that you can discover content that you need for. Various different scenarios and we, have workflow, orchestration, with composer, which, allows you to stitch various, scenarios together, on top of the whole platform so, that's the whole end-to-end platform. Capabilities, that we are providing, with our smart analytics, capability, as. I, mentioned we have launched, more than 50, key, capabilities, just in 2019. This. Is just a subset of that, that. We have but. Now what I want to talk about is focus, on a key theme we. Have been noticing, across, the industry and. The theme is around convergence. If you. Think about it there are three, or four key, areas. Where we are seeing lot of conversion, first. Of all data, lakes data warehouses. These. These concepts. That were there in organizations. Are coming together and, organizations. Want to think about a fluid, system that allows you to go ahead and analyze, data at scale and. Not worry about what, a data warehouse is what a data Lake is but, want to have an environment where both of them can coexist. So. That's one a stream, and batch processing, we are seeing like, convergence. Across all of these systems hybrid. Multi, cloud environments. How they can coexist and then finally, analytics. AI ml. Organizations. Used to have another silos, where, you had analysts. Which were like data, people that understood data really well could. Analyze any data and then he had like PhD data scientists, within organizations, they were siloed, in their world to. Go ahead and create like complex, machine learning models, we are seeing convergence. Across that whole frame where, we. Now we are seeing with newer innovations ease, of use different. Folks are doing different. Power. Playing different parts within that organization. Let's. Talk about data Lake and data warehousing. Traditionally. Data warehouses, have been used to better understand, your business right you can do reporting, you can do dashboarding you can do basic analysis, you have standard metrics to, measure your measure, your business. And all the. Challenge was you could never scale, with. The data warehouse it's massive amounts of data that you had so, you aggregated. You summarized but but, allowed you to better understand, your business with, data Lakes you could explore, your business you, wanted to get. All the data within a data Lake and you could use it for different kind of exploration you, could go ahead and analyze, data, at scale in them now--and, and. Leverage it for that you could have structured, unstructured, semi-structured. Data in it what. We are seeing is a convergence. Across, all of them one. Of the challenges, of having the. Old model, of the data. Lakes and data warehouses, was it was always the compute and storage was, linked together one. Of the big challenges on data Lakes actually, is as the, size of data grows, within it you, are paying the price of compute, for. The growth in storage because they are all. Decoupled. Together within the same same, environment, where. We are planning to go is a very different approach so, if you think about it what we are trying to do is you. Can go ahead and decide to store your data either, in a data warehouse which, is a structured, data store with bigquery or in, your data Lake with the GCS, it doesn't, matter you. Can leverage the same set of engines or similar set of engines whether it is a MapReduce, SPARC engine, beamed flink, spark. Or. Bigquery, sequel, across, all the data that you have across different, environments, that you're storing so you could store data your park' and Orsi files in in. Google Cloud storage and use, bigquery to bigquery, sequel, to go ahead and analyze it or you. Can store it in bigquery and leverage. SPARC code to go ahead and analyze that side of the environment so completely. Fluid, environment is, what we are looking at and, if you think about bigquery big, are highly. Scalable, cloud. Data warehousing, solution, it. Can, handle petabytes, and petabytes of data we have our. Customers, who have like tens, to hundreds of petabytes of data within, within, bigquery and we have other customers who are building smaller. Data Mart's with few terabytes within, within, an organization's, we have a spectrum, of, companies. We have organizations. That, are actually, publishing, billions, of events every day within, bigquery, in real time and then, using, that for real-time analytics, and and, and. Getting value out of it and then, we as. Part of the as part of bigquery we also have embedded machine learning with just two lines of sequel, you can create machine, learning models and start start. Using them so you can do better. Segmentation. You can do better regression, you can do a linear regression logistical. Regression you. Can do matrix. Factorization clustering. And we also are adding different capabilities in that space, so. If you look at that the. Main thing for for, in.
2019. That we have focused on is this interoperability. Of data. Lakes so, there are as I was mentioning there are two key capabilities, with bigquery now, you, can fire a federated, query on your data in, data. Lake in GCS, and then, you, can use any of the engines on top of bigquery to go and analyze that with. That let me actually invite, James Malone to the stage to, share more about our, new. Capabilities, in open source as well as show, us a demo. Thanks. I appreciate it it's nice to be here so, I'm James I'm a product, manager in, data. Analytics and what, I want to do is I'm going to show you a demo in just a minute to showcase, how we're trying to move managed, open-source forward but, quickly I want to explain why this is really important, Google, has great infrastructure, and open, source projects, are essentially, the amalgamation, of thousands, and tens of thousands and hundreds of thousands, of needs we, think there's real power and joining the two together and managed services that solve problems for you here, you see the logos of two of our managed open-source services, cloud data proc which runs the SPARC and Hadoop and friends, like presto, ecosystem, and cloud, composer and we really want to lower the bar of running the managed open source so you can use the open source tools without having to be an expert on standing them up and tuning, them and trying to debug them and figure them out I mentioned. Moving open source forward, the, SPARC and Adobe ecosystem, has been somewhat stagnant for a while there's, a general interest, in moving to kubernetes, recently. We announced that, cloud data proxeny. Spark on kubernetes. Clusters, that are managed by data proc this, gives you a unified control plane to run open source workloads, in this case spark and flink soon, without. Having to think about yarn, or kubernetes, and that's exactly, the demo that I'm going to show you Sudhir. Also mentioned interoperability, I'm going to show you a really cool feature of data proc and bigquery that, hits it how we've tried to make open source interoperable, as well so. With that I'm going to go ahead and show, you my demo. Perfect. Ok so, here. We see cloud data proc this is the Google Cloud console, here, I have a production, cluster that I've set up this, is a traditional. Hadoop yarn cluster, so if you've used spark and Hadoop you'll sort of understand this, is a you, know a traditional Hadoop, cluster, here it, has 20 nodes I created this right before the the, demonstration, started you'll. Actually see I over, provisioned, this cluster, and we have, the autoscaler, turned on and the autoscaler realized, nothing was going on and intelligently, scaled my cluster down to try and save me money because there's, no sense in running more than I have to I also. Have a kubernetes. Engine cluster, here and maybe, an, IT admin. Or somebody created this cluster and they want multiple, groups to share this cluster to better utilize resources, but. As you, see this cluster wasn't part of cloud data proc so what I'm going to walk you through is how we actually attach this cluster, to cloud data proc and make it a spark and Hadoop cluster as well the. First thing that I'm going to do though, is show. You exactly, how we do that. So. The way that we attach, to this cluster cloud data proc is we run a helm install and if you're familiar with kubernetes, you're. Probably somewhat familiar with helm the package manager for kubernetes. There's. Just a few options here that i've set you. Can see that I've shaded my my. White, listing token if you. Want to join our alpha there's an email, that you can just email us at we're. Happy to invite anybody to our alpha that that wants to join well. That's running I'm gonna go ahead and run a job on this, yarn cluster, I will, explain what this job does after, I go ahead and run the job I'm. Just gonna clone a pre-existing, job because I think as much as you all want me to stand up here and type, in front of you it's not that exciting. We're. Gonna go ahead and name the job and label, it as yarn so we know what's going on and this. Job if you're not familiar with data proc data proc has an API, for managing clusters, and also jobs and workflows so you don't have to be an expert and use SSH tunnels, and all that crazy business if.
I Were you I'd be wondering what does this job do and this is the feature that I wanted to show on how we've tried to create interoperability, between cloud data products like bigquery and open source so. This, job tries. To find the most popular sub reddits in 2018, and 2019 but. What's really cool about this job is we're doing it all in spark data frames and reading directly from bigquery, storage so. You can see that we're creating a spark data frame we're. Specifying the, big table that excuse, me the bigquery data sets that we want to read from and they're split out by yours and then, we're directly. Reading that data into a spark data frame we're not moving data around we're not having to copy data all, of this is basically spark native code and it's directly, reading, from bigquery you don't have to really think about trying. To duplicate data and copy data and shuffle data we're. Then grouping that data and counting the number of posts by each subreddit, and the, job finishes, by enumerate, in all of the specific, results, so if you're unfamiliar with this connector it's a really interesting thing to be aware of because, it allows you to seamlessly use, bigquery. And spark, together quite, well you. Can also now see that two, things actually happen tier 1, the autoscaler, has realized that there's more work going on on the cluster so it's actually started adding capacity. To, the original, yarn cluster, which, is quite interesting and. It's actually started adding preemptable, workers so, that's useful but. You can also see that the kubernetes cluster here, is now and you don't think about yarn or kubernetes we're not creating this split world it's, all seamlessly, integrated, together. To. Sort of show you an example of why this is really important, I can take this job that I ran on my yarn cluster, I can, simply clone it give, it a new name and. Moving. This work load from yarn to kubernetes is as simple as just changing the cluster and, then. Submitting, it that's. It so, you're not thinking about having to re architect, for kubernetes you're, not having to think about what, to do and how to shift it's all it's all very integrated, and seamlessly and we're bringing all of the features of data proc the security. The logging the integrations, to the kubernetes world as seamlessly as possible and, finally you can see that I have the results from querying bigquery, directly and we can now ascertain, which sub reddits are the most popular thank. You very much. Thank. You. I, think. The the most important, thing that James. Showed you the autoscaler, which is key, capability, we went GA with it's. Pretty amazing, it will automatically, scale make sure that it only uses capacity, that you really need to the, other thing was the ability to run spark jobs directly on bigquery data we, have a great. Customer story from. 4. To 2 Sigma they are actually a hedge fund out of New York they. Collect, massive, amounts of data they, use machine, learning with, spark ml to, go ahead and run on top of bigquery using. Using. Our capabilities, and then make, trading decisions, and. Define, trading, strategies, on top of top, of all of that I love sequel but I know sometimes sequel, can be limiting, enabling. Spark on top of bigquery just opens up a completely different, kinds. Of scenarios, for organizations. With that, I want to invite Shane. Who. Is CTO. Of a big data at the HSBC to share their story have they thought about these. Sanction. So. Good afternoon, everyone really, excited, to be here I'm Shane, Lamont and I have the privilege of being part of an awesome IT team here helping to transform the. Way HSBC. Offers, banking, products to, our customers you may, be familiar with the HSBC, brand but let me give you a few stats about the company, nearly. 4,000, offices 14. Million customers, were, present in 66. Countries and, territories, 53. Billion dollars in revenue and, we have over. 230,000. Employees, we, were founded in 1865. And that's a long heritage and to put 150. Years into context, I'd like to show you something. It's. A typewriter and, here's something else that, has been around for 150, years and while the technology has changed that. Keyboard is, entirely, relevant today and you, might have one on your phone and for, HSBC over. 150, years our, technology, has changed but. Secure, banking and offering. Great banking services, to customers is still essential, today. So. Because, we have lots of customers and we process 1.5. Trillion, dollars, in payments every day we, gather a lot of data so if we're about to start a data and analytics, project, with.
A Lot of data that's a big decision and because, that decision can impact our, employees. Our customers and. Our reputation we. Want to make sure that we get it right. So. What, is a big decision in an analytics, project and Sudhir. Covered some of this very. Well I might add but. For us a typical big decision, is do, you want a data Lake or a data. Warehouse very common and the, data Lake is where we load lots of unstructured data we, want to understand, it and explore, it and see what insights are in it whereas, a data warehouse, is where, after we understand, what it is we want to structure, it explain, it, and reported and. It's. A big decision and you might be making one of those decisions, at the moment you might have made it a few years ago or you, might be about to make it so, what. If that decision looked like if you're doing it on premise, or. If you're doing it on cloud. So. If you're making a big decision on premise that's like buying a nice shiny, new car you're. Going to spend a lot of money you. Have to decide what you want to do because, you're making a choice. Capacity. Or speed, and, once. You've made that decision and you've gone through all of that analysis, once you order it you have to wait for it to be delivered and then once you start using it you're going to use it for a long time that's, what on-premise looks like. Now. If you're making that decision on cloud, you. Have a lot more options that's more like going. On holiday, and rent in a car you, want it for a specific purpose or. A short period of time and. You. Therefore will use it for a particular outcome, that. You want you, might want it for somewhere sunny space, carrying, things or a convertible and. Therefore. At the, end of the short period of time you return it you get the outcome you get the value and if you start a new project or want to rent it again you, can make a different decision that, flexibility, is really important. But. Nevertheless it's. Still a decision and you might be thinking okay. Shane what, does hsbc, do do. You load your and, process your data in a, data. Warehouse or, do. You put it into a data Lake and my. Answer to that is yes. We do, we. Use both because. We need both and cloud. Enables. Us to have both so. Let's take a little look at an. Architecture, on cloud. Through Google cloud where, it enables us to have both of these, you've. Probably seen lots of architecture, diagrams, like this so let me walk you through this particular one we. Acquire. Data explore. It and report, it and the, way we do that in HSBC, is we, have lots. And lots of data sources, thousands. Not not, a couple, and we. Move that data first of all securely, and within all of the data processing, regulations, and, and we move that first, of all to google cloud storage through. A product called Juniper X now. Once you've got it in Google Cloud storage we have lots of choices if we, want to use MapReduce. Or spark. Because, we've got some legacy code in there we, can have a data Lake by spinning up data proc but. If we want to use some clever third-party, libraries, or perhaps we've written them ourselves we might process, it through compute engine and if, we are fortunate enough to have a Greenfield project. Then. We may use server lists with something like dataflow or some of the other tools. Putting. That information back, into Google Cloud storage once you've process that means that it's then available to the bigquery data, warehouse and, once it's in the data warehouse all, your third-party tools, and your analysts. And everybody who wants to use that get. All the benefits up get all the benefits of that so. The. Great thing about this architecture is is it's not theoretical we're, using this in HSBC, today and through, the great work that we get through our cloud services, team and our, finance, team we, use, this architecture, to reduce the reporting, for liquidity risk, down, from over 10 hours to 30 minutes and that's, a really fantastic outcome. If you, have daily, reporting. With, tight regulatory, deadlines because. That gives us more time to deal with some of the issues that come up in any, large complex, operational, system, now. Sudhir, did say earlier that. You. Can now run some of your spark and clever processing, on the data that's in your data Lake and, you're. Sorry on your data warehouse. And the, data warehouse can, query some of the data that's in your lake so now, that I think about this I might. Have misled you and I possibly, should apologize, it's. Not that cloud gives you both. It's. That it gives you more than both and. That's. Really important for us because it keeps our options open and we. Therefore it can make those decisions time and time again and the best decision that we want to make for us at that time. So. To summarize, the. The. Business environment especially, in banking, is changing. Rapidly and the rate of change is the fastest, it has ever been and the, slowest, that it will ever be.
So. The ability to make decisions quickly and. Flexibly. Is, really. Really important it's a competitive advantage for us and Google. Cloud enables. Us to focus less. On the infrastructure, and all the admin, around the decision and it. Enables us to focus more on the outcome and for. HSBC that, outcome, is to. Make banking. With HSBC, simpler. Better and faster, for our customers, and that for, us is a great decision okay. I'd like to pass back to Sudhir, thank you very much for your time and over. Here. Thank. You. So. As, Shane. Was saying you. Don't have to make the choices it's the the convergence, is allowing, you to go ahead and pick, any one of the technologies, as you go one, of the big announcements that we have that. We're launching this week that's. Coming is bigquery. Reservations, we, understand, with bigquery, we. Have an on-demand model, which was like pretty popular with lot of a lot of customers, a lot of organizations. Do want to have a consistent, payment. Model where they basically, get a predictable bill and with, bigquery reservations. We are providing two key capabilities. One, give. You, flat. Billing, model which you can decide how much you, want to spend on analytics. And then go. Ahead and reserve, that and that's the maximum bill you will get for, bigquery going, forward so that's, one key capability, second. Is your workload, management you, do want to take whatever, you want to spend on analytics, and maybe decide. By. Department you want to go ahead and split that spend, go, ahead and give different amount, of spend for marketing, versus, finance. Department, versus a different team you, can do that you can also make it based on workloads, where you, say hey the BI and, reporting, should, get X amount of X, amount of spend versus. ETL jobs get why you, can do those workload, based decision, making so, super excited about that this has been one of the big, asks, from our large enterprise, customer, so this, will give you predictability, as well as flexibility, in managing it and the second thing is it's all going to be available online so to, get more, bigquery. Capacity. That you need you can just go online and start, start. Leveraging the tooling now that's, one big big, thing here. Is an example of a TCO comparison, that we have done actually we haven't liked the ESG, is I think the agency that did did, this where. You can see what is the total cost of ownership over, a period of time with, bigquery versus, all the different key, cloud based, analytic solutions, that, you have. The. Next thing that I want to talk about is convergence, of stream and batch processing. Batch. Des the streaming data is growing, really fast across, organizations. And we do want to go ahead and make sure that, we are we are providing you with capability. That, allows you to now build one, set of code, that, runs, consistently, for, streaming, as well as bad scenarios, we have lot of customers, where the, similar kind of data may be coming from streaming, let's, say with pub/sub or Kafka, or it's. Coming on G CSS, files and they want to go ahead and do common processing, and and with, beam you can basically do that as, a data flow as an engine or many, of the other engines. One of the other things that that we have focused on is not, just bringing, together stream, and batch processing, but also providing. You the consistent, framework which. Allows you to go ahead and pick your choice off of, programming.
Languages, You can use Java or Python, you. Can leverage go. You can leverage sequel, with dataflow, sequel now and you. Can use a for execution, any one of the engines that that, comes with it like you can use dataflow of course as a service product but you can use fling you can use, spark. You can use various different engines for for you to run this or. Run your workloads that's, in short the key thing is we, are making it easy for you to build consistent. Programming. Model where you can process your streams of data or batches of data in, a single consistent environment and run, it and with dataflow. Sequel, which, we are announcing, beta. Of it allows you a few more capabilities, and now like running. Information. From GCS files and all just make it more easier for you to go ahead and do that with, that let me actually invite Rob to share more of what they're doing in Spotify, leveraging, this platform, you. Know thanks. Thanks. Sudhir and quick. Show of hands who's familiar with Spotify. Okay. I thought so so I don't have to explain myself quite, that much so. My name is Rob I'm a product manager at Spotify responsible. For data collection and data is. The foundation, of almost everything we do at Spotify for example. We'll analyze your listening habits packaged. Together 30 songs which we, think you're gonna like but, you probably, haven't discovered yet put, them in a 30 song playlist and deliver to you every Monday that's, called discover weekly and it's one of Spotify the most popular features. What. We use data for so much more than personalization. Recommendation. If, you listen to a track or a podcast, we. Record that so, that way we can pay the artist the label and the other rights holders, then. We use data for business intelligence to. Determine, what features we should build next and. We use data to run experiments, on those features to figure out if they were any good and the, list goes on, at. The center of all this is the event delivery infrastructure, and that's what my team is responsible for. So. In 2016. Spotify, was moving to the cloud, the. On-premise event delivery infrastructure was processing a million and a half events, per second and this, was growing quickly. And. We as a company had an opportunity to get to the cloud as fast, as possible, so, we were willing to accept some tech debt in order, to make the migration path backwards, compatible, for data production and data consumption. By. Doing this this was a great decision in 2016. As we were able to get to the cloud but now it's happening some adverse effects on our data quality and I'll get to that in a second. So. This is what the architecture, looks like in 2018. So. To explain a little bit about what's going on in the diagram if, you listen to a track or podcast, on your mobile phone we, send that to the access point where, we log the data to disk and the, fact that we logged this to disk and the libraries we use to do so this is really the tech debt that's been getting us problems, then. A process picks, up the data from the access point sent, it to pub/sub the. ETL process, reads it from pubsub puts, it on an hourly bucket with all the other like data that was produced that our removes. Duplicates makes. It GDP our compliance and, puts it in its final resting location, in cloud, storage at this, point we, have an immutable data set accessible, to the rest of the company. But. Things change drastically from, moving the cloud in 2016. To, getting to this point in 2018, the. Big one which swept through the industry was gdpr and, we. Did design with use of privacy in mind in 2016. Rajini, PR was an entirely different animal we. Had to adapt the architecture, drastically. To me able to meet the compliant the new compliance needs. More. Interesting, changes perhaps was. Just how popular bigquery, became with Spotify his data science community and the, industry, in general and. Similarly. The popularity of consuming, data in a streaming fashion, whereas, we had only designed the system to support patch so.
As You see at the top of the diagram there we, have it's, almost a different event delivery infrastructure to support streaming. But. Possibly an even bigger change was, our explosion of scale. So, in 2016. The on-premise infrastructure had a million-and-a-half events per second so, we designed the cloud, solution for four and a half so triple two. Years later we far exceeded our design, so. We had a almost, 8 million events per second 2018, and I. Tend, to think about data, and the, size of data in events, per second but, the real Wow numbers is what I just pulled up this morning, 502. Of billion, events every day so. Over half a trillion events, every day and that's, 350, terabytes of data produced every day and, the. Thing is data. Increases faster than just with your number of users as the. Company becomes more and more successful you invest more and more in your data and insights community, which. Then increases the, demand for data and therefore, also the supply, so. We need to make sure that we're ready for the next several years of quadratic, scale increase. So. Late last year we decided it was time to rebuild we. Want to be ready for the scale of the future but we also want to make sure that we're designing with. 2019. S tech landscape, in mind. Concretely. That means we take advantage of. The latest and greatest from Google Cloud latest. And greatest from Spotify score infrastructure, and design. With gdpr in mind, from the beginning. And. The. Business value that we get from this is, to remove the tech debt and improves, modifies data quality to unlock lots more. So. The strategy, that we're taking in order to achieve this we're, redesigning the way that you produce data so, the we've added SDKs, for all the different ways that people use Spotify we've. Changed what this data looks like on the other side so we've updated, the schemas and change the consumption, format and we, redesigned, the workflow, that users use to add new datasets and to modify schemas. Once. We've, redesigned. These interfaces we, put the old we spun up the old infrastructure, behind the new interfaces and began, moving some production traffic over and, we're. Able to do this easily because of largely. Because of the cloud, once. The code is all ready we press some buttons would pay some money and now we have to massive event delivery systems, running with production data. And. While that's going on while, we're more and more production data over to the new infrastructure. Which is at this point new, interfaces, but the old infrastructure, the, real rebuild is happening under the surface. So. This is where we are today so November, 2019. Because. We changed the interfaces, because, we, wanted to double down on kubernetes, and dataflow we've. Had to rebuild, almost, all the components, that we had in the 2018, infrastructure, so everything you see in a green circle there is new. Yes. You, can see that streaming is still sort of an appendage there we've, managed to reduce streaming, from a separate infrastructure, to, a dataflow job which, is. Doing some schema transformation, in gdpr and there's, more that we can do there but, this this is where we're at today. And. The. Preliminary results, have, been super promising, we've, managed to reduce costs significantly both. In terms of actual, dollars but also in, terms of operational, savings but. The hidden benefit here has been that spot. Our engineers, are now able to focus more on what's giving real value so in this case it's improving, the data quality as opposed. To putting out fires that we had from the scale, we didn't expect or focusing. On tech dead. So. We changed the wheels of the bus while it's moving we, moved to 23% of production traffic to. The new infrastructure. But. Now it's time to try to change the engine, we've. Been working heavily with Google and this is still aspirational, but, we've been working heavily with Google to make this the reality and. We firmly believe that an event delivery, architecture, of this scale can, be just a service publishing to pub/sub and a, dataflow job which, do it does all the parts of the ETL and puts, it in the three places where Spotify his data community would like to read, the data, so. We're doubling down on our investment, in infrastructure, so, we can stop investing, so much in infrastructure, in the future and. Isn't that why we're all moving to cloud in the first place. If you thought this was exciting, we've got some blog posts on Spotify labs where you can read more there's.
Three Blog posts which are articulate, how we move to the cloud in the first place as well as some war stories along, the way and, then there's a blog post we published last week which talks about the last two years and the experience there. And. We. Found this really exciting we're hiring. Sudhir. It's. Actually, pretty fascinating to, see the journey of once, you're migrated, to cloud how you can keep, modernizing. The infrastructure, to reduce the complexity, and not carry the tended technique. That you have and, the other corner conversions. That we are seeing is this hybrid and multi cloud environment, where, your data environment, which is spanning across multiple different data centers on Prem across, multiple clouds bringing. Them together is, actually one of the key focus. Areas for us especially, with data fusions, general, availability and, more, importantly, ability, to. Go ahead and bring data from on from Isis systems as well, as your different. Environments, that you may have in cloud in into. A single place, is what data fusion allows you to do and especially with, this lineage, capability. You will be able to do feel level lineage across. Your whole data pipelines, and manage, that so it's like pretty exciting, for us to, go ahead and see what, organizations. Can do with it and how they can benefit from from, these key capabilities. It's just a visual view of at, every field level you should be able to go ahead and track, back where the data came from and if something goes wrong you'll, be able to figure out what, was the source system, for it at, what time it was updated when, did the pipelines run so whole, end-to-end, audit, capability, on your data pipelines, is what, will be available to you in, coming a couple, of weeks, with. That another, thing is we we see lot of, organizations. Leveraging, our datasets. That are in IR ad systems, to, go ahead and do better management. Of their spend better return on investment, now. Based, on that platform, so one, of the things we are going to do is all of the ad sources. That we have around. From Google Club ability. To pull the data from ads. As well as YouTube sources, into bigquery we, are going to make that absolutely free, starting. First of Jan so, it will be easier for more organizations. To go ahead and collect, this data process. It and analyze it, better, the. Fourth, area which is super, critical for, organizations where we're seeing convergence, is this, AI and analytics, as I said earlier. Organizations. Have lot of challenges, and historically. The challenge was, there. Was not enough capacity. To go ahead and work on all of these problems organizations. Have with. Their talent, that they had because. Doing machine learning and AI was such, a niche. Skill. With, very few PhD, data scientists, that you always had more problems, then you could work on our. Goal, actually, is how, do we democratize. Solving. Of these problems, making it super simple for you, to go ahead and work, on these machine learning problems, or these, key problems, that can be solved with better machine, learning models and all and we are enabling, organizations. To, do that using. Bigquery ml what. Is bigquery ml it's literally, two lines of code along, with the sequel statement, you, say create model, model name and give. The input data set and say which column, do you want to go ahead and.
Predict. At the at the end of it and it, will automatically, create a machine learning model based, on that and for, prediction, you can just go ahead and call select. Ml dot predict and then give, us what what you are predicting and then give the input data set and that's it it's, as simple as that so, you will be able to go do that with bigquery ml, of course you can also use auto ml tables which, allows you to pick up a table. In bigquery pick. A column that you want to predict and we will create the machine learning model but, a lot of times organizations. Want to use models. That they're familiar with the, analysts. May understand, and they want to go ahead and do that and that's what bigquery ml allows you to do we, have all the different types of models that that, we provide around. Classification. Models regression, models as, I said earlier you have, k-means clustering. You have recommendations. From, 4-legged. No you can use matrix factorization for. For, recommendations. A lot, of marketing analysts, are using the. K-means clustering to do segmentation you can take all your data set around your, customer, data and you can say can, you go in and cluster them based, on different attributes, and then do, better customer. Experiences. For. Your for your organization's. So now, a lot of different, kinds of models we are enabling one. Of the key things we are also focusing, on is model. Import/export, I think, it's super important, that you build a model in bigquery you. Can do some batch actions on it like for example you do better segmentation, take, that input and go ahead and do a better experience, in an offline fashion, maybe, sending emails maybe sending a different, kind of offers to or to folks but, we do believe more. And more organizations will, take those models and operationalize. Them into their front-end, applications, and for, that we, are basically enabling. Importing, as well, as exporting, off of, models, through, tensorflow over, a period of time so, when you do that you can create a model you can take it out and then actually host it in machine, learning engine ml engine cube, flow and then actually start running those for. Serving. Platforms, a great, example of this is geotab. Geotab runs actually, a fleet of vehicles. Across, Canada, and they have sensors, on each one of these vehicles they're, basically, collecting, our various different temperature. Speed. Different. Sensor. Attributes. And then, basically, able to consolidate. That run, machine learning models on a geospatial data at scale and then, provide interesting. Insights, to city planners and selling, that as a service now so, our really innovative company, that's, doing this so, bringing it all home the, key thing is there. Is convergence happening across, different, kind of scenarios, and with. Google Cloud especially, our smart analytics platform, what, we are enabling organizations to, do is not, get stuck into one or another type of systems, we, are building platform, that allows you to converge, these concepts. Whether, it is data Lake or data warehouse you don't have to think of you can you, can, yesterday. Shane was saying this you can have a data Lake in the morning and Daryl a data warehouse in the afternoon and you can leverage both of them interchangeably you, just have to worry about what data you're bringing and what, value you're going to get out of it . similarly.
Stream. And batch processing, i think, over a period of time most, of the systems are going to be streaming. Based so, today, if you start building applications, that are, both. Stream. And batch processing, in a consistent fashion, you can absolutely move it to the next generation without, having that much tech debt and then. Hybrid, multi cloud environments, we are living in an hybrid environment, in most cases making. It easier to go ahead and break down the silos is. One of the key areas and then of course analytics, AI machine, learning is going, to be a key component across, across. The. Whole organizations. This. Is not just possible for. Us to do it by ourselves we. Do have a huge partner ecosystem we. Do, know. That you have invested a lot in different kind of tooling whether, it's bi tooling, with this ETL tooling whether it is data, integration. Analysis. Visualization, we. Work with a huge, set of partner ecosystem to. Go ahead and make sure all of those tools are working great on top of our platform so, that your investments, are always protected, and you're getting the best value from the whole whole. Environment, we. Also have a massive. Ecosystem. Of our GSI, partners, and we are working closely, with them to go ahead and provide targeted. Solutions, for, example we are working with Accenture. To. Go ahead and provide Accenture. As well as Infosys, on migration, services, and, we're working with Wipro on some of the BI capabilities. That we are going to provide so, basically, different kind of solutions, across, all of the different kinds of GSI. Partners, that are there worldwide. And then, finally. Looker. We. Announced. The intent to by looker earlier this year it's. A great tool a lot of our customers, already, go. Ahead and use them they were one of our top. Partners, and in bi space. Already. And our, whole, thing is we're still pending. Approvals, but our whole goal will be to provide end to an integrated, platform, starting. From collection, processing. Storing, analyzing. And building. Data applications, and visualizing so, that's going to solve a key end-to-end problem. Having. A common data model with, their modeling, layer is going to be super, critical for organizations one. Of the challenges, I see with organizations, when I talk to leader. Says, there's, no common understanding, of metrics, within organizations, if you go ask what the cost of goods is are or margin, definition, is in different departments, you get different answers and so, providing a common semantics, modeling layer is going, to help a lot for organizations. Augmented. Analytics, we have this team around AI, powered, bi so, we want to go out and invest in that and make it available and then of course industry, applications so. We have end-to-end solutions, for our for. Our customers, is going to be key focus area other. Than that I do want to mention we. Have been we, have made a lot of progress in last three years, or so when. I joined Google two years back I think, we were in mostly Challenger, or different. Like. You know in quadrants, and all, Challenger. And visionary, and all of that but in last one year we have been in leader quadrant, or leader, in every one of the waves that has come out around analytics, and. Whether it's dmsa, whether it's foresters, cloud. Data warehousing, wave or the recent one which, is streaming analytics wave which is our, debut like this is the first time we, just, were. Invited, to it and we directly launched, into the the. Leader space. In that so super, excited, about the recognition we are getting. Finally. I'm going to thank you all for coming here I know it is almost afternoon, time so thanks. For joining us and please do give us feedback through. The feedback application. Thank you. You.
2019-12-15 16:15